A Bayesian Spatial Analysis of the Heterogeneity in Human Mobility Changes During the First Wave of the COVID-19 Epidemic in the United States
AMERICAN STATISTICIAN(2022)
摘要
The spread of COVID-19 in the U.S. prompted nonpharmaceutical interventions which caused a reduction in mobility everywhere, although with large disparities between different counties. Using a Bayesian spatial modeling framework, we investigated the association of county-level demographic and socioeconomic factors with changes in workplace mobility at two points in time: during the early stages of the epidemic (lockdown phase) and in the following phase (recovery phase) up to July 2020. While controlling for the perceived risk of infection, socioeconomic and demographic covariates explain about 40% of the variance in changes in workplace mobility during the lockdown phase, which reduces to about 10% during the recovery phase. During the lockdown phase, the results show larger drops in mobility in counties with richer families, that are less densely populated, with an older population living in dense neighborhoods, and with a lower proportion of Hispanic population. When also accounting for the residual spatial variability, the variance explained by the model increases to more than 70%, suggesting strong proximity effects potentially related to state- and county-wise regulations. These results provide community-level insights on the evolution of the U.S. mobility during the first wave of the epidemic that could directly benefit policy evaluation and interventions.
更多查看译文
关键词
Bayesian methods, COVID-19, Human mobility, SARS-CoV-2, Social distancing, Spatial modeling
AI 理解论文
溯源树
样例
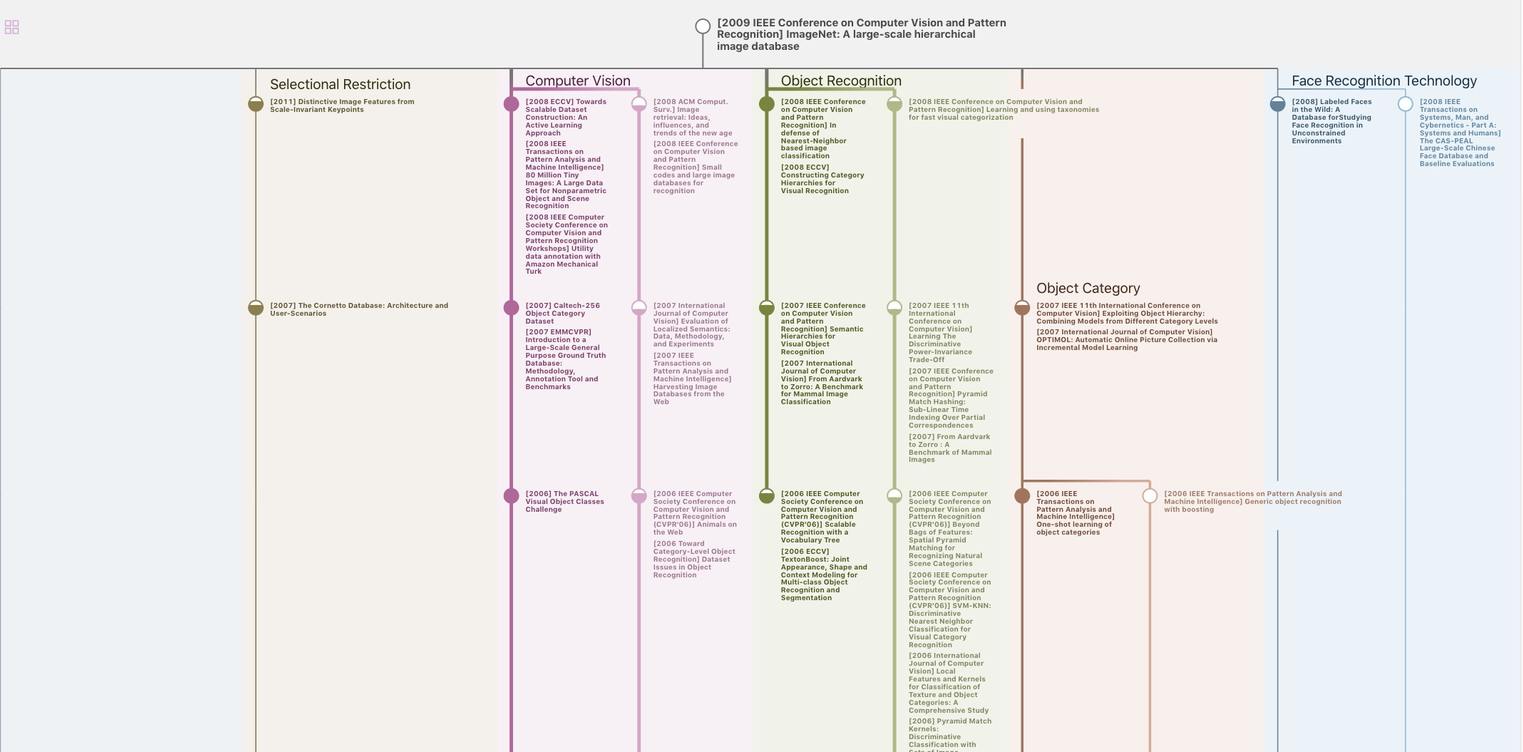
生成溯源树,研究论文发展脉络
Chat Paper
正在生成论文摘要