Side-constrained minimum sum-of-squares clustering: mathematical programming and random projections
Journal of Global Optimization(2021)
摘要
This paper investigates a mathematical programming based methodology for solving the minimum sum-of-squares clustering problem, also known as the “k-means problem”, in the presence of side constraints. We propose several exact and approximate mixed-integer linear and nonlinear formulations. The approximations are based on norm inequalities and random projections, the approximation guarantees of which are based on an additive version of the Johnson–Lindenstrauss lemma. We perform computational testing (with fixed CPU time) on a range of randomly generated and real data instances of medium size, but with high dimensionality. We show that when side constraints make k-means inapplicable, our proposed methodology—which is easy and fast to implement and deploy—can obtain good solutions in limited amounts of time.
更多查看译文
关键词
MINLP, k-means, Random projections, Side constraints
AI 理解论文
溯源树
样例
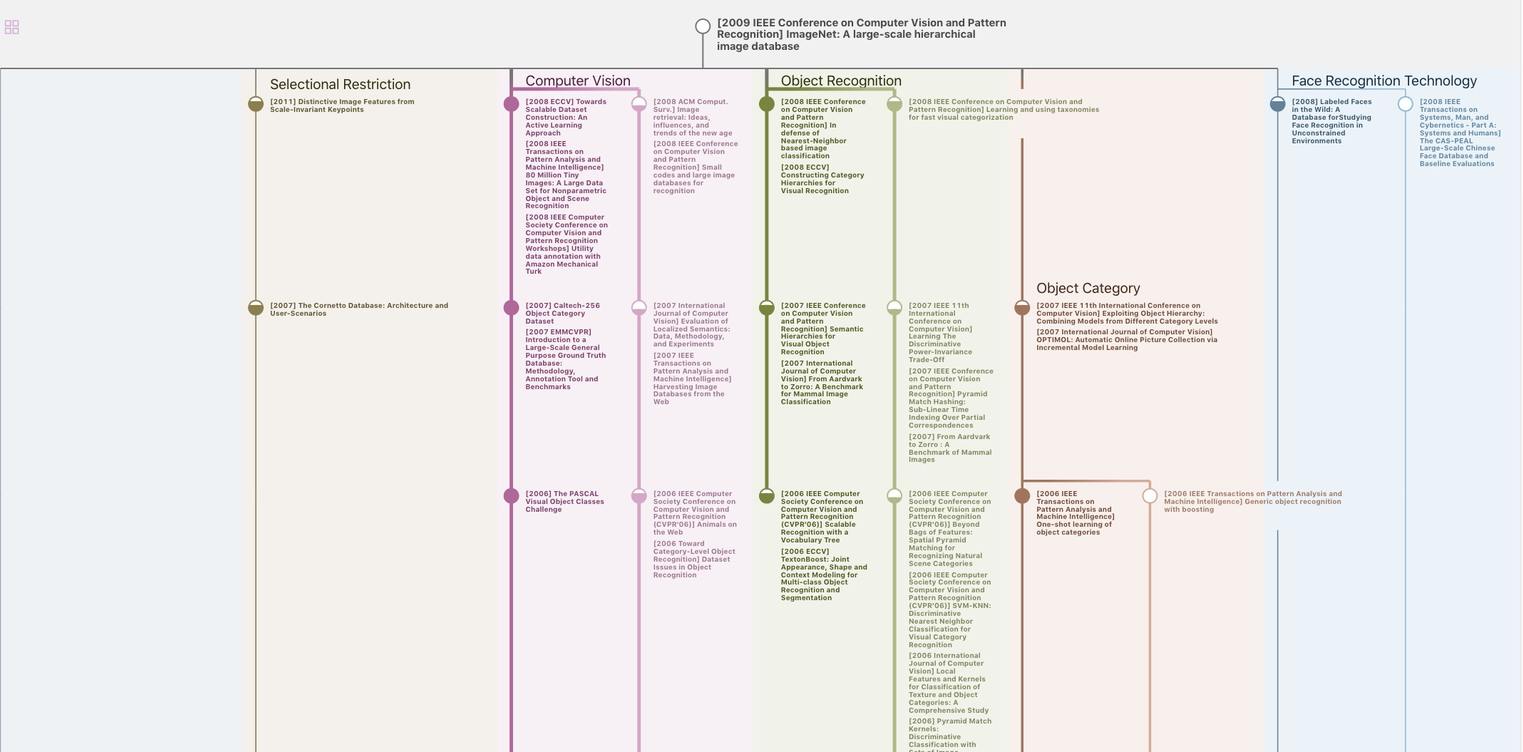
生成溯源树,研究论文发展脉络
Chat Paper
正在生成论文摘要