Source tasks selection for transfer deep reinforcement learning: a case of study on Atari games
NEURAL COMPUTING & APPLICATIONS(2021)
摘要
Deep reinforcement learning (DRL) combines the benefits of deep learning and reinforcement learning. However, it still requires long training times and a large number of instances to reach an acceptable performance. Transfer learning (TL) offers an alternative to reduce the training time of DRL agents, using less instances and in some cases improving performance. In this work, we propose a transfer learning formulation for DRL across tasks. A relevant problem of TL that we address herein is how to select a proper pre-trained model that will be useful for the target task. We consider the entropy of feature maps in the hidden layers of the convolutional neural network and their actions spaces as relevant features to select a pre-trained model that is then fine-tuned for the target task. We report experimental results of the proposed source task selection methodology when using Deep Q-Networks for learning to play Atari games. Nevertheless, the proposed method could be used in other DRL algorithms (e.g., DDQN, C51, etc.) and also other domains. Results reveal that most of the time our proposed method is capable of selecting source tasks that improve the performance of a model trained from scratch. Additionally, we introduce a method for selecting the most relevant kernels for the target task, the results show that transferring a subset of the convolutional kernels results in similar performance to training the model from scratch while using less parameters.
更多查看译文
关键词
Source task selection, Kernel selection, Transfer learning, Deep reinforcement learning
AI 理解论文
溯源树
样例
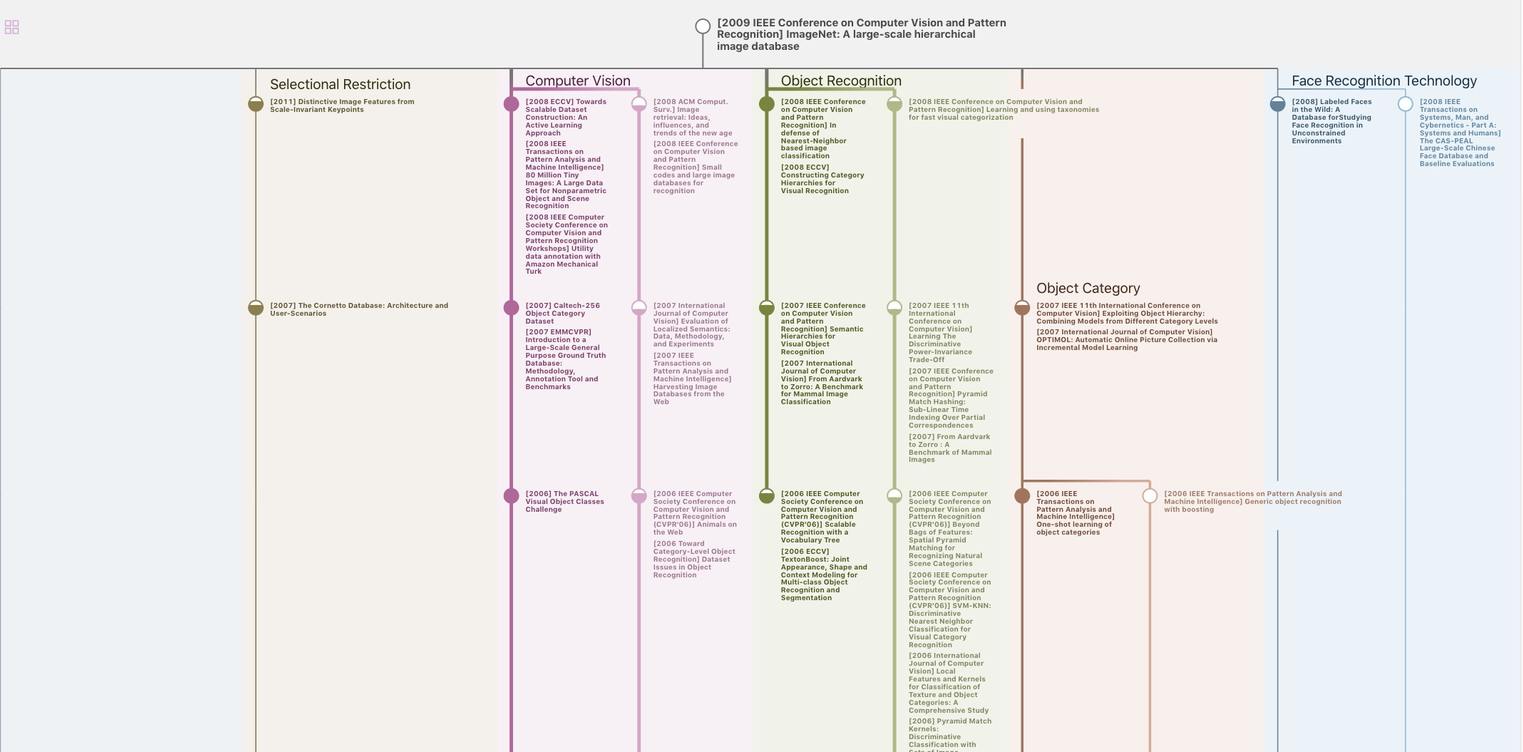
生成溯源树,研究论文发展脉络
Chat Paper
正在生成论文摘要