Fully Bayesian Human-Machine Data Fusion for Robust Online Dynamic Target Characterization
JOURNAL OF AEROSPACE INFORMATION SYSTEMS(2021)
摘要
This work examines the problem of fusing human operator observations with probabilistic information extracted by an automated data fusion system, in the context of dynamic multitarget track characterization for large-scale surveillance. This soft data fusion problem is challenging because human operator observation errors are difficult to calibrate a priori and in general exhibit subtle conditional dependencies. Furthermore, the efficacy of combining inputs from operators depends heavily on operator error characteristics as well as track characterization uncertainty. A hierarchical fully Bayesian probabilistic model is developed to explicitly account for human sensor quality and Markovian dependencies in human reports. This model is used to perform online Bayesian inference via Gibbs sampling to simultaneously update the data fusion system's knowledge of human sensor characteristics and target type probabilities. A strategy is also developed for value of information assessments to automatically query human operators for inputs as needed. Efficient approximations of high-dimensional human sensor parameter posterior distributions via Dirichlet probability density function (pdf) moment matching and parameter tying are also developed to provide significant computational speedups with little information loss. Results for different simulated operator profiles and automated target characterization baselines show that fully Bayesian fusion improves track characterization performance, while intelligently accounting for uncertain operator characteristics.
更多查看译文
关键词
Data Visualization,Multisensor Fusion,Probability Density Functions,Automated Machine Learning,False Positive Rate,Computing,Automatic Target Recognition,Maximum Likelihood Estimation,Long Short Term Memory,Kalman Filter
AI 理解论文
溯源树
样例
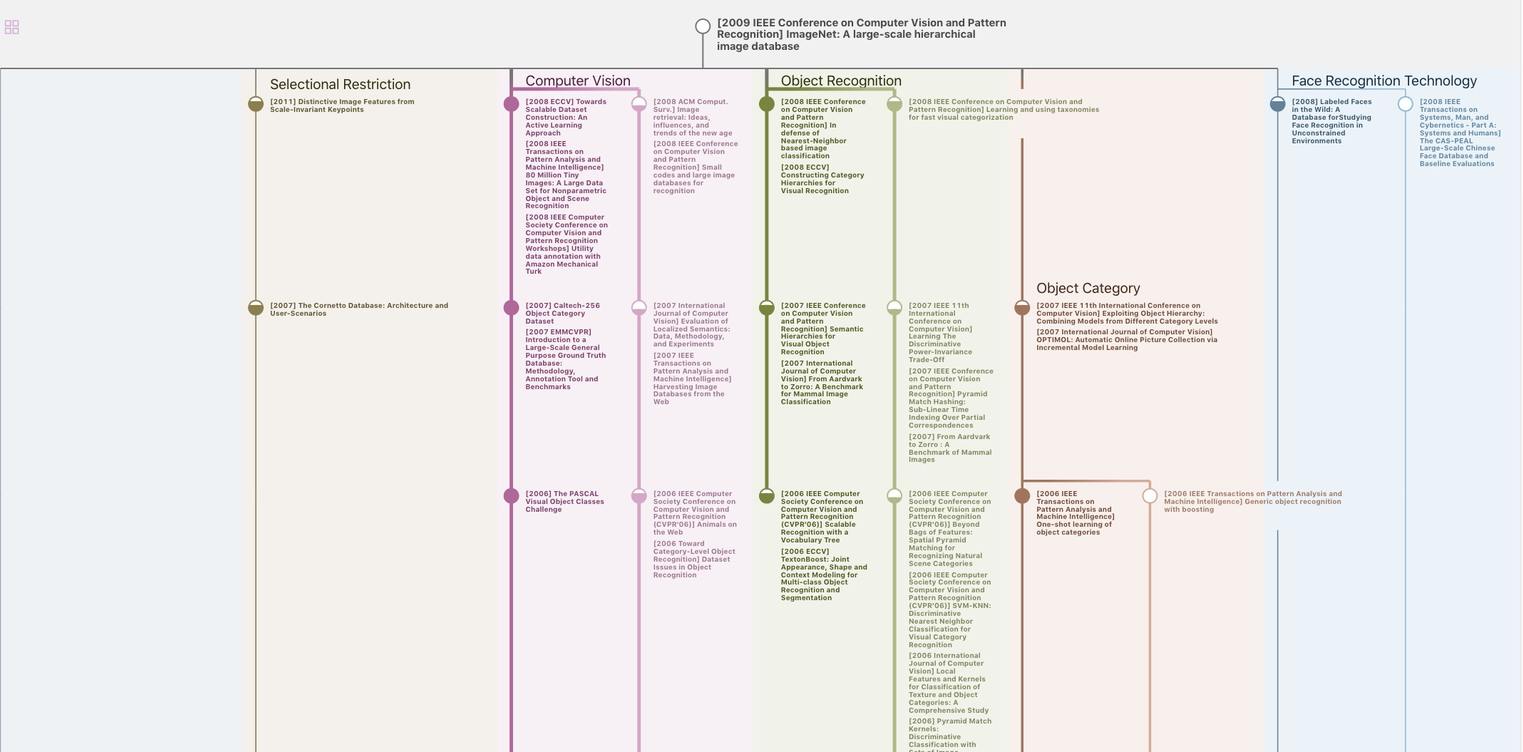
生成溯源树,研究论文发展脉络
Chat Paper
正在生成论文摘要