Closed walk sampler: An efficient method for estimating the spectral radius of large graphs
2017 IEEE International Conference on Big Data (Big Data)(2017)
摘要
The spectral radius of a graph, i.e., the largest eigenvalue of its adjacency matrix, is of high interest in graph analytics for Big Data due to its relevance to many important properties of the graph including network resilience, community detection and the speed of viral propagation. Accurate computation of the largest eigenvalue of extremely large graphs is infeasible due to the prohibitive computational and storage costs, and also because full access to many social network graphs is often restricted to most researchers. In this paper, we present a new and efficient algorithm called Closed Walk Sampler (cWalker) which solves both of the above-mentioned problems. Unlike previous methods which try to extract a subgraph with the most influential nodes, the cWalker samples only a small portion of the large graph via a simple random walk, and arrives at an estimate of the largest eigenvalue by estimating the number of closed walks of a certain length. Our experimental results using real graphs show that the cWalker is substantially faster while also achieving significantly better accuracy on most graphs than the current state-of-the-art algorithms.
更多查看译文
关键词
cWalker,prohibitive storage costs,prohibitive computational costs,viral propagation speed,community detection,Big Data,largest adjacency matrix eigenvalue,spectral radius estimation,network resilience,simple random walk,social network graphs,graph analytics,Closed walk sampler,closed walks
AI 理解论文
溯源树
样例
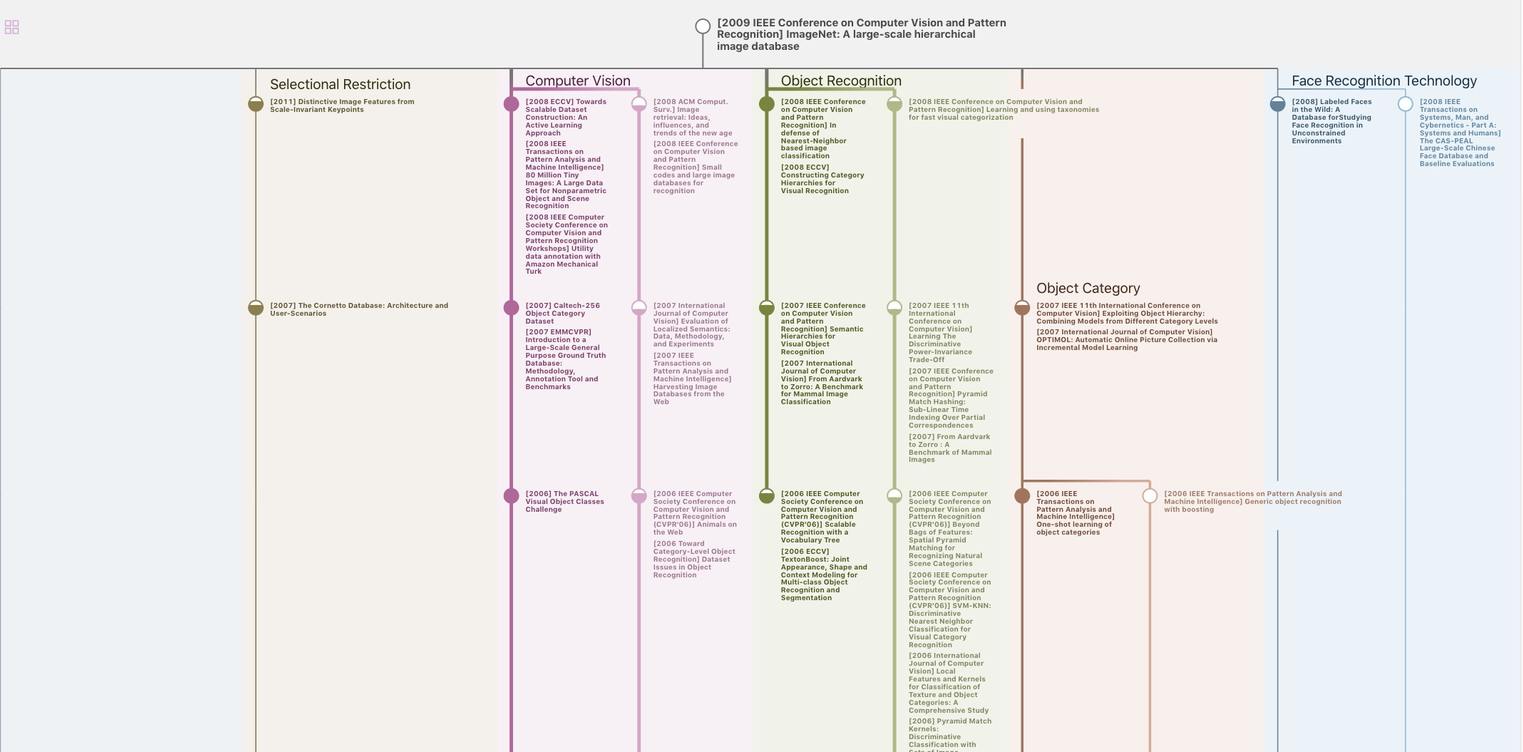
生成溯源树,研究论文发展脉络
Chat Paper
正在生成论文摘要