Statistical Seasonal Prediction Of European Summer Mean Temperature Using Observational, Reanalysis, And Satellite Data
WEATHER AND FORECASTING(2021)
摘要
Statistical climate prediction has sometimes demonstrated higher accuracy than coupled dynamical forecast systems. This study tests the applicability of springtime soil moisture (SM) over Europe and sea surface temperatures (SSTs) of three North Atlantic (NA) regions as statistical predictors of European mean summer temperature (t2m). We set up two statistical-learning (SL) frameworks, based on methods commonly applied in climate research. The SL models are trained with gridded products derived from station, reanalysis, and satellite data (ERA-20C, ERA-Land, CERA, COBE2, CRU, and ESA-CCI). The predictive potential of SM anomalies in statistical forecasting had so far remained elusive. Our statistical models trained with SM achieve high summer t2m prediction skill in terms of Pearson correlation coefficient (r), with r >= 0.5 over central and eastern Europe. Moreover, we find that the reanalysis and satellite SM data contain similar information that can be extracted by our methods and used in fitting the forecast models. Furthermore, the predictive potential of SSTs within different areas in the NA basin was tested. The predictive power of SSTs might increase, as in our case, when specific areas are selected. Forecasts based on extratropical SSTs achieve high prediction skill over south Europe. The combined prediction, using SM and SST predictor data, results in r >= 0.5 over all European regions south of 50 degrees N and east of 5 degrees W. This is a better skill than the one achieved by other prediction schemes based on dynamical models. Our analysis highlights specific NA midlatitude regions that are more strongly connected to summer mean European temperature.Significance Statement The prediction of summer temperature is important for the estimation of weather related risks relevant for the society. Specifically for economy, agriculture, and implications on human health. Due to the chaotic system, and in the absence of only a single agent controlling the climate system, physics based dynamical models do not estimate correctly seasonal mean temperature. We tested whether a statistical model could achieve better predictions of summer temperature over Europe. Our data-driven model uses information from datasets of North Atlantic sea surface temperatures and European soil moisture, and achieves predictions with higher skill compared to physical numerical models. Moreover, we identified the regions in the North Atlantic Ocean that are more strongly related to the high prediction skill of European summer temperature. These findings suggest that statistical models should be used in operational seasonal forecasting.
更多查看译文
关键词
Europe, Atmosphere-land interaction, Atmosphere-ocean interaction, Seasonal forecasting, Statistical forecasting, Seasonal variability, Machine learning
AI 理解论文
溯源树
样例
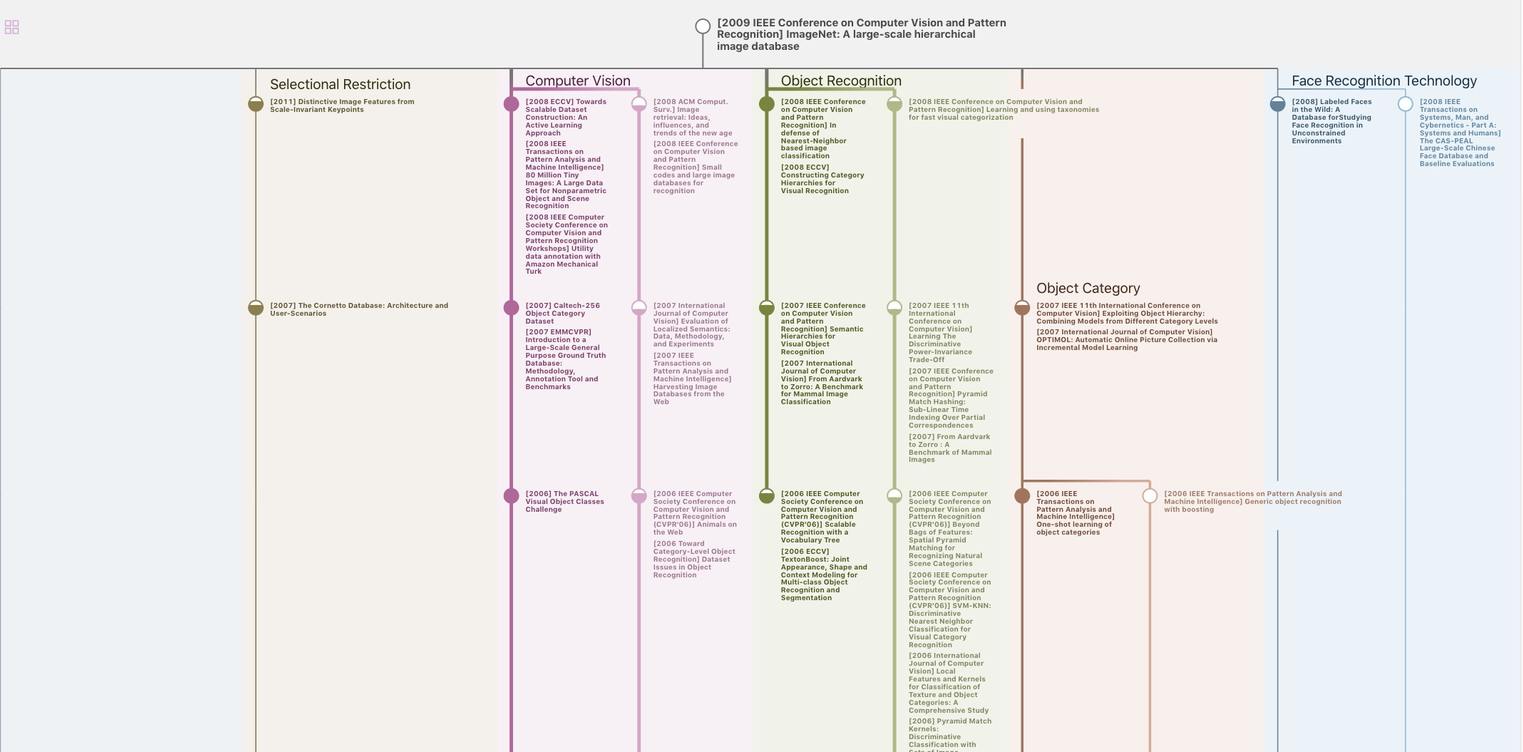
生成溯源树,研究论文发展脉络
Chat Paper
正在生成论文摘要