Adoption Of Machine Learning Technology For Failure Prediction In Industrial Maintenance: A Systematic Review
JOURNAL OF MANUFACTURING SYSTEMS(2021)
摘要
Failure prediction is the task of forecasting whether a material system of interest will fail at a specific point of time in the future. This task attains significance for strategies of industrial maintenance, such as predictive maintenance. For solving the prediction task, machine learning (ML) technology is increasingly being used, and the literature provides evidence for the effectiveness of ML-based prediction models. However, the state of recent research and the lessons learned are not well documented. Therefore, the objective of this review is to assess the adoption of ML technology for failure prediction in industrial maintenance and synthesize the reported results. We conducted a systematic search for experimental studies in peer-reviewed outlets published from 2012 to 2020. We screened a total of 1,024 articles, of which 34 met the inclusion criteria. We focused on understanding the datasets analyzed, the preprocessing to generate features, and the training and evaluation of prediction models. The results reveal (1) a broad range of systems and domains addressed, (2) the adoption of up-to-date approaches to preprocessing and training, (3) some lack of performance evaluation mitigating the overfitting problem, and (4) considerable heterogeneity in the reporting of experimental designs and results. We identify opportunities for future research and suggest ways to facilitate the comparison and integration of evidence obtained from single studies.
更多查看译文
关键词
Failure prediction, Fault prediction, Machine learning, Predictive maintenance, Systematic review
AI 理解论文
溯源树
样例
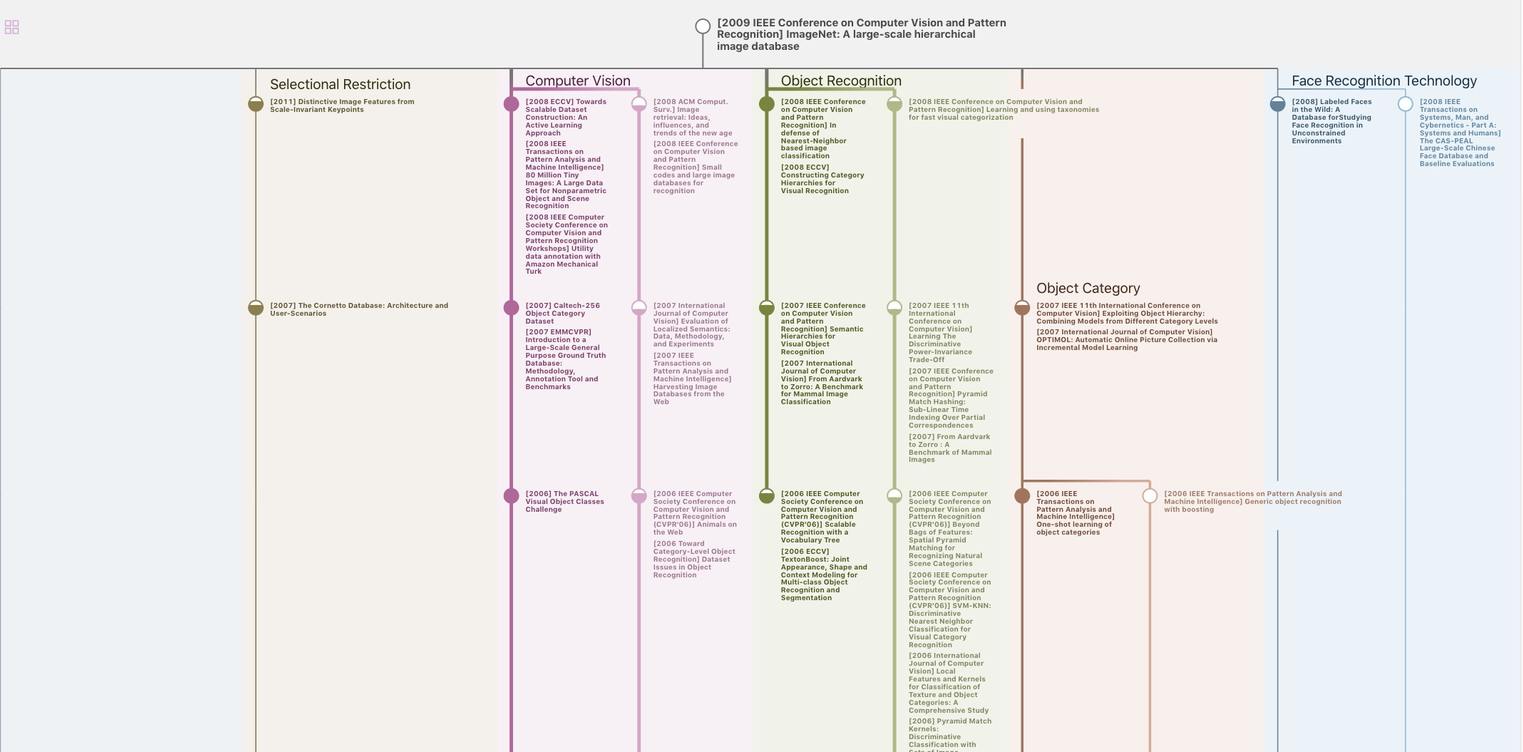
生成溯源树,研究论文发展脉络
Chat Paper
正在生成论文摘要