Deep Learning Experiments For Tropical Cyclone Intensity Forecasts
WEATHER AND FORECASTING(2021)
摘要
Significance statement Scientists have been searching for decades for breakthroughs in tropical cyclone intensity modeling to provide more accurate and timely tropical cyclone warnings. To this end, we developed a deep learning (DL)-based predictive model for North Atlantic 24- and 6-h intensity forecast. We simulated 2019 and 2020 tropical cyclones as if in an operational forecast mode, and found that the model's 24-h intensity forecast outperformed some of the most skillful operational models by 5%-22%. Also, the 6-h intensity model produced realistic intensity labels for synthetic tropical cyclone tracks. These results highlight the potential for using deep neural network-based models to improve operational hurricane intensity forecasts and synthetic tropical cyclone generation.Reducing tropical cyclone (TC) intensity forecast errors is a challenging task that has interested the operational forecasting and research community for decades. To address this, we developed a deep learning (DL)-based multilayer perceptron (MLP) TC intensity prediction model. The model was trained using the global Statistical Hurricane Intensity Prediction Scheme (SHIPS) predictors to forecast the change in TC maximum wind speed for the Atlantic basin. In the first experiment, a 24-h forecast period was considered. To overcome sample size limitations, we adopted a leave one year out (LOYO) testing scheme, where a model is trained using data from all years except one and then evaluated on the year that is left out. When tested on 2010-18 operational data using the LOYO scheme, the MLP outperformed other statistical-dynamical models by 9%-20%. Additional independent tests in 2019 and 2020 were conducted to simulate real-time operational forecasts, where the MLP model again outperformed the statistical-dynamical models by 5%-22% and achieved comparable results as HWFI. The MLP model also correctly predicted more rapid intensification events than all the four operational TC intensity models compared. In the second experiment, we developed a lightweight MLP for 6-h intensity predictions. When coupled with a synthetic TC track model, the lightweight MLP generated realistic TC intensity distribution in the Atlantic basin. Therefore, the MLP-based approach has the potential to improve operational TC intensity forecasts, and will also be a viable option for generating synthetic TCs for climate studies.
更多查看译文
关键词
Tropical cyclones, Forecast verification, skill, Forecasting techniques, Operational forecasting, Short-range prediction, Statistical forecasting, Deep learning, Neural networks
AI 理解论文
溯源树
样例
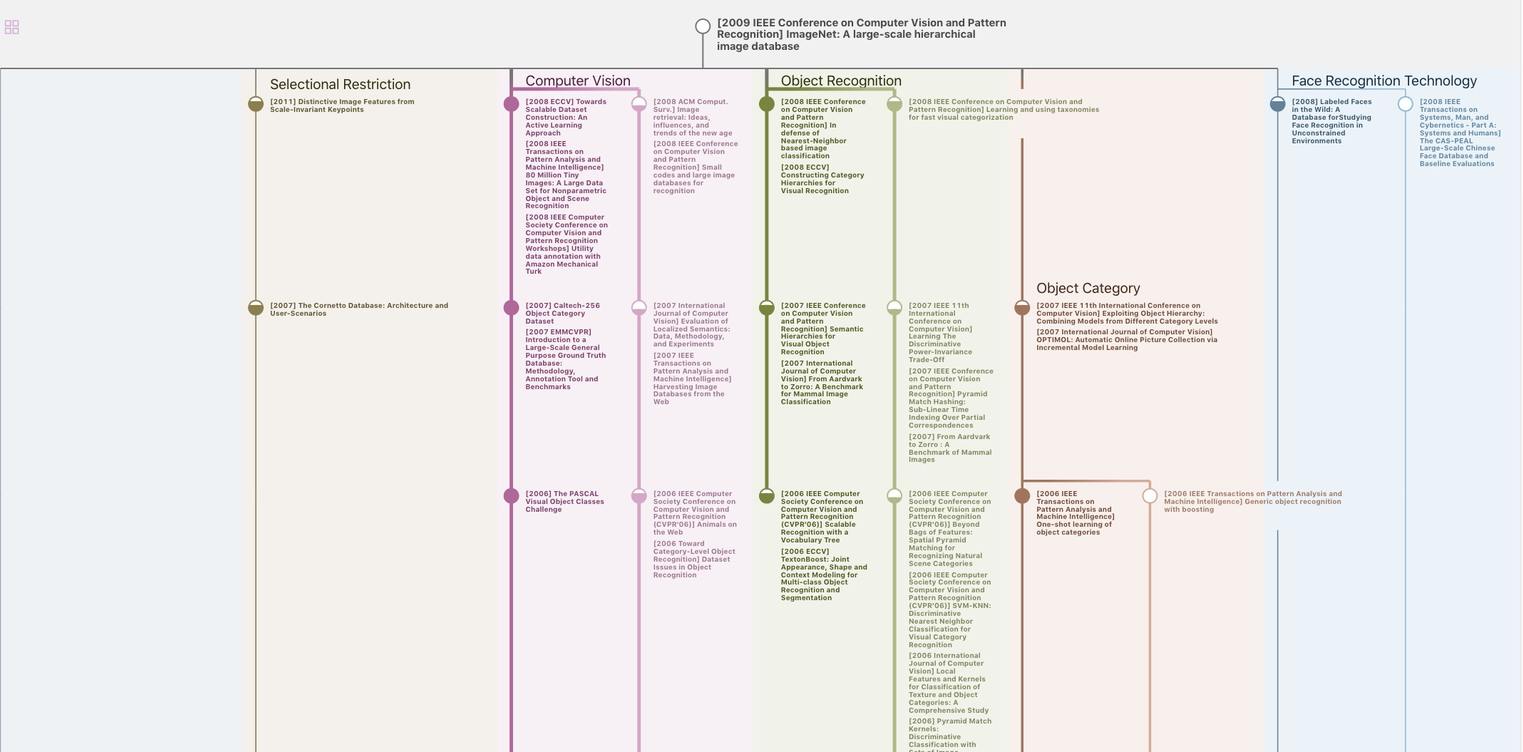
生成溯源树,研究论文发展脉络
Chat Paper
正在生成论文摘要