Exploiting Spatio-Temporal Dependencies For Rnn-Based Wind Power Forecasts
2019 IEEE PES GTD GRAND INTERNATIONAL CONFERENCE AND EXPOSITION ASIA (GTD ASIA)(2019)
摘要
Forecasting wind power production using machine learning techniques proves difficult in practice due to the high stochasticity of the wind power time-series. To improve these forecasts, we include wind speed and wind direction from neighboring points in the vicinity of the wind turbine or wind farm in question. We assume that this additional information can improve the wind power forecast as the wind speed and direction at the wind turbine is influenced by the distributed wind speeds and directions scattered over the topology.To make best use of these additional inputs, we propose using convolutional long-short term recurrent neural networks (convLSTM). Their advantage is the property of including temporal dependencies arising from the (wind) time-series as well as spatial dependencies obtained from geographically scattered wind forecasts. convLSTM shows promising results to modulate both temporal as well as spatial dependencies on wind power output time-series.
更多查看译文
关键词
convolutional neural networks, recurrent neural networks, wind power forecasting
AI 理解论文
溯源树
样例
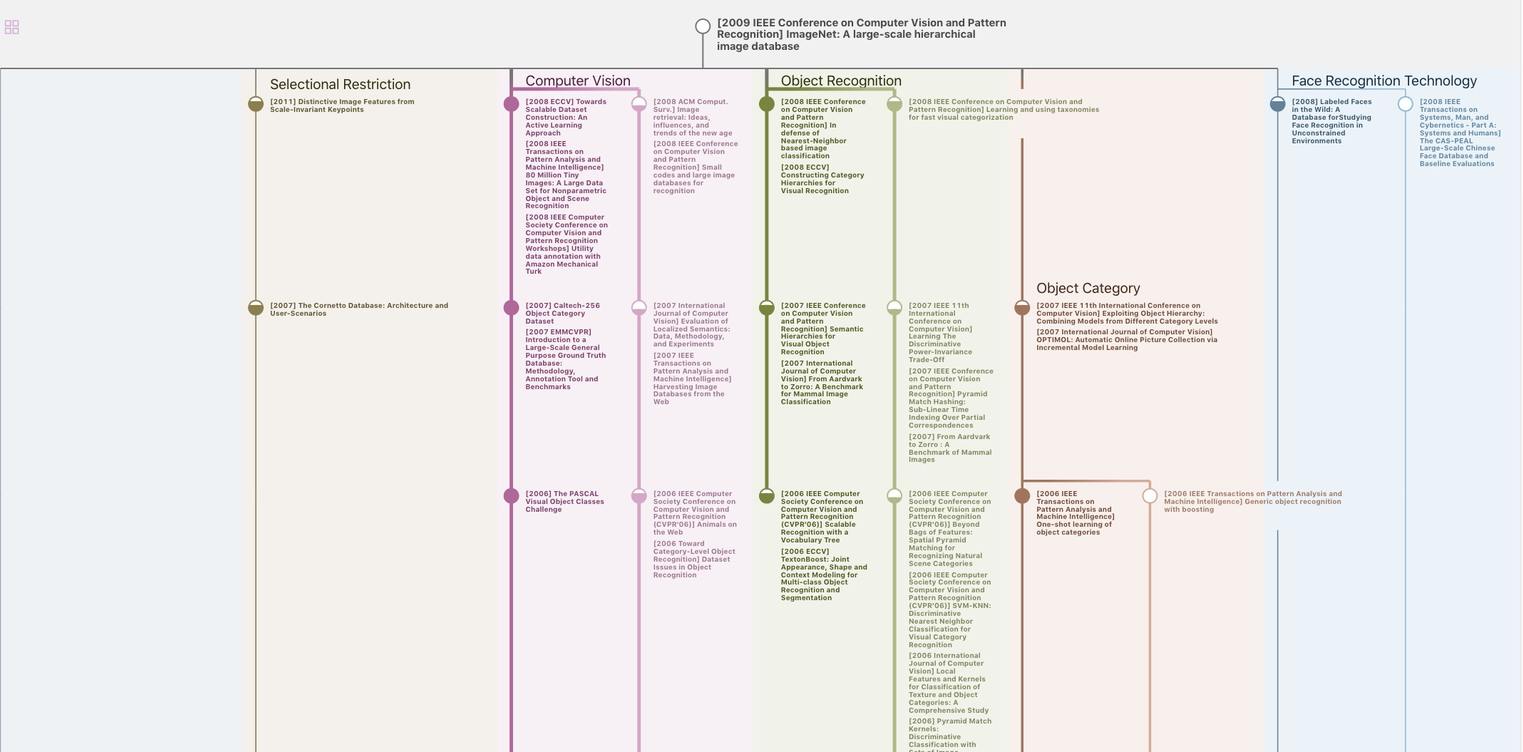
生成溯源树,研究论文发展脉络
Chat Paper
正在生成论文摘要