Automatic Vertebra Labeling In Large-Scale Medical Images Using Deep Image-To-Image Network With Message Passing And Sparsity Regularization
DEEP LEARNING AND CONVOLUTIONAL NEURAL NETWORKS FOR MEDICAL IMAGING AND CLINICAL INFORMATICS(2019)
摘要
Efficient and accurate vertebra labeling in medical images is important for longitudinal assessment, pathological diagnosis, and clinical treatment of the spinal diseases. In practice, the abnormal conditions in the images increase the difficulties to accurately identify the vertebrae locations. Such conditions include uncommon spinal curvature, bright imaging artifacts caused by metal implants, and limited field of the imaging view, etc. In this chapter, we propose an automatic vertebra localization and labeling method with high accuracy and efficiency for medical images. First, we introduce a deep image-to-image network (DI2IN) which generates the probability maps for vertebral centroids. The DI2IN adopts multiple prevailing techniques, including feature concatenation and deep supervision, to boost its performance. Second, a message-passing scheme is used to evolve the probability maps from DI2IN within multiple iterations, according to the spatial relationship of vertebrae. Finally, the locations of vertebra are refined and constrained with a learned sparse representation. We evaluate the proposed method on two categories of public databases, 3D CT volumes, and 2D X-ray scans, under various pathologies. The experimental results show that our method outperforms other state-of-the-art methods in terms of localization accuracy. In order to further boost the performance, we add 1000 extra 3D CT volumes with expert annotation when training the DI2IN for CT images. The results justify that large databases can improve the generalization capability and the performance of the deep neural networks. To the best of our knowledge, it is the first time that more than 1000 3D CT volumes are utilized for the anatomical landmark detection and the overall identification rate reaches 90% in spine labeling.
更多查看译文
AI 理解论文
溯源树
样例
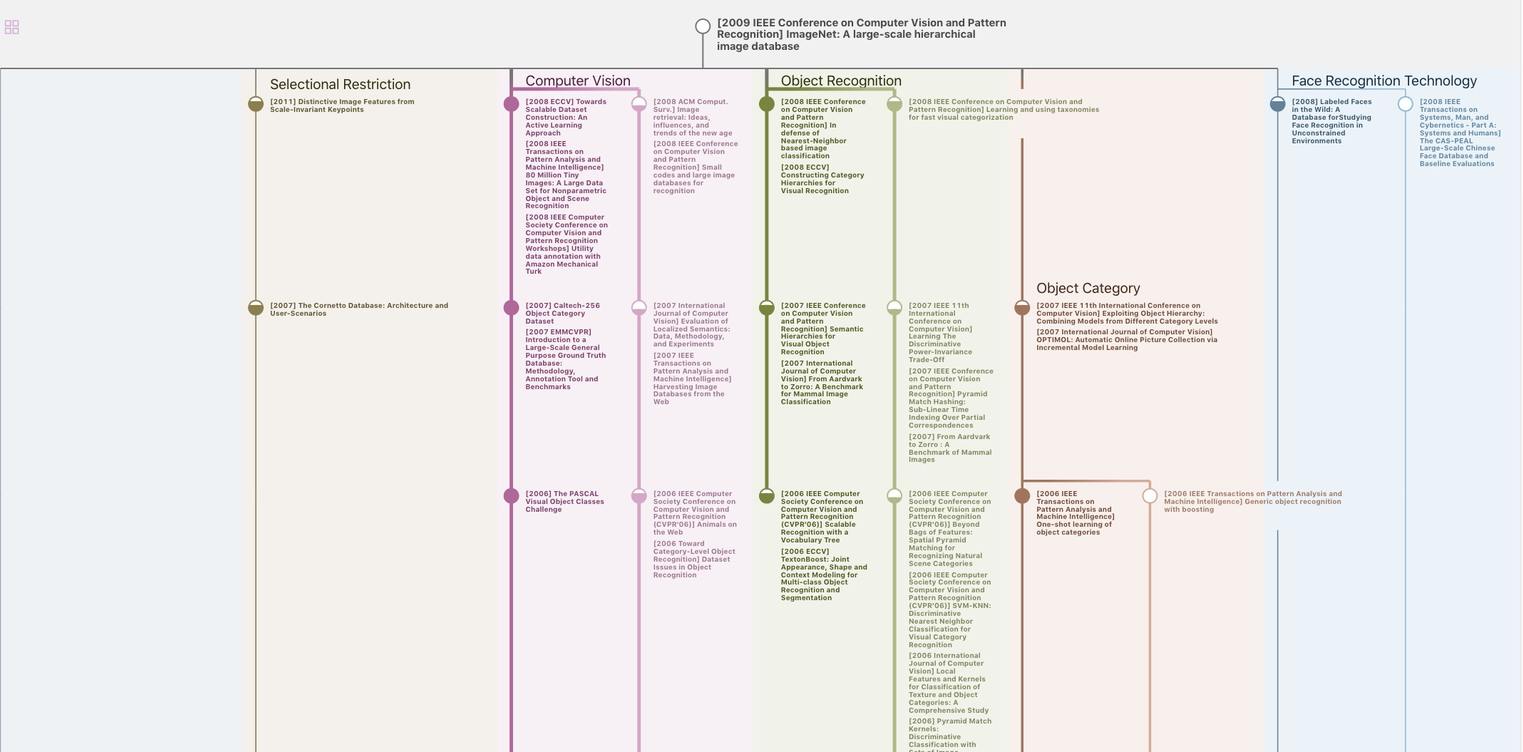
生成溯源树,研究论文发展脉络
Chat Paper
正在生成论文摘要