Rigid Motion Invariant Classification of 3D Textures and Its Application to Hepatic Tumor Detection
BIOMEDICAL TEXTURE ANALYSIS: FUNDAMENTALS, TOOLS AND CHALLENGES(2017)
摘要
Soft tissue in 3D medical data sets is often associated with 3D textures. The granularity demonstrated by tissues in medical images due to natural tissue structure gives rise to 3D or 2D textures. In this chapter, we focus on 3D textures for the purpose of classifying tissue patches into healthy and pathological. In our case, both patch types come fromthe same organ but one is neoplastic. We view the problem of tissue segmentation/classification into pathological and normal as a problem of 3D texture segmentation/identification. Texture segmentation/identification requires two major components, which are often developed together: the representation of the original data and the segmentation/classification algorithm. We model textures as spatial stochastic processes in order to address the variability due to intratissue natural variation, and due to noise. To address this problem, we use isotropic multiresolution analysis to extract 3D rigid motion invariant texture patch signatures. Using a measure of statistical disparity combined with local average intensities, we create low dimensional features that can be used for distinguishing normal tissue patches from pathological ones. We describe how to eliminate the additional variability of 3D tissue texture models due to rigid motions. We also present experimental results on contrast enhanced CT scans of the liver to demonstrate our approach for tissue discrimination, using 3D rigid motion invariant texture classification.
更多查看译文
关键词
Isotropic MultiResolution Analysis (IMRA), Gaussian Markov Random Field (GMRF), Support vector machines, Liver cancer, 3D texture classification, Isotropic filter, Kullback-Leibler divergence, Feature space
AI 理解论文
溯源树
样例
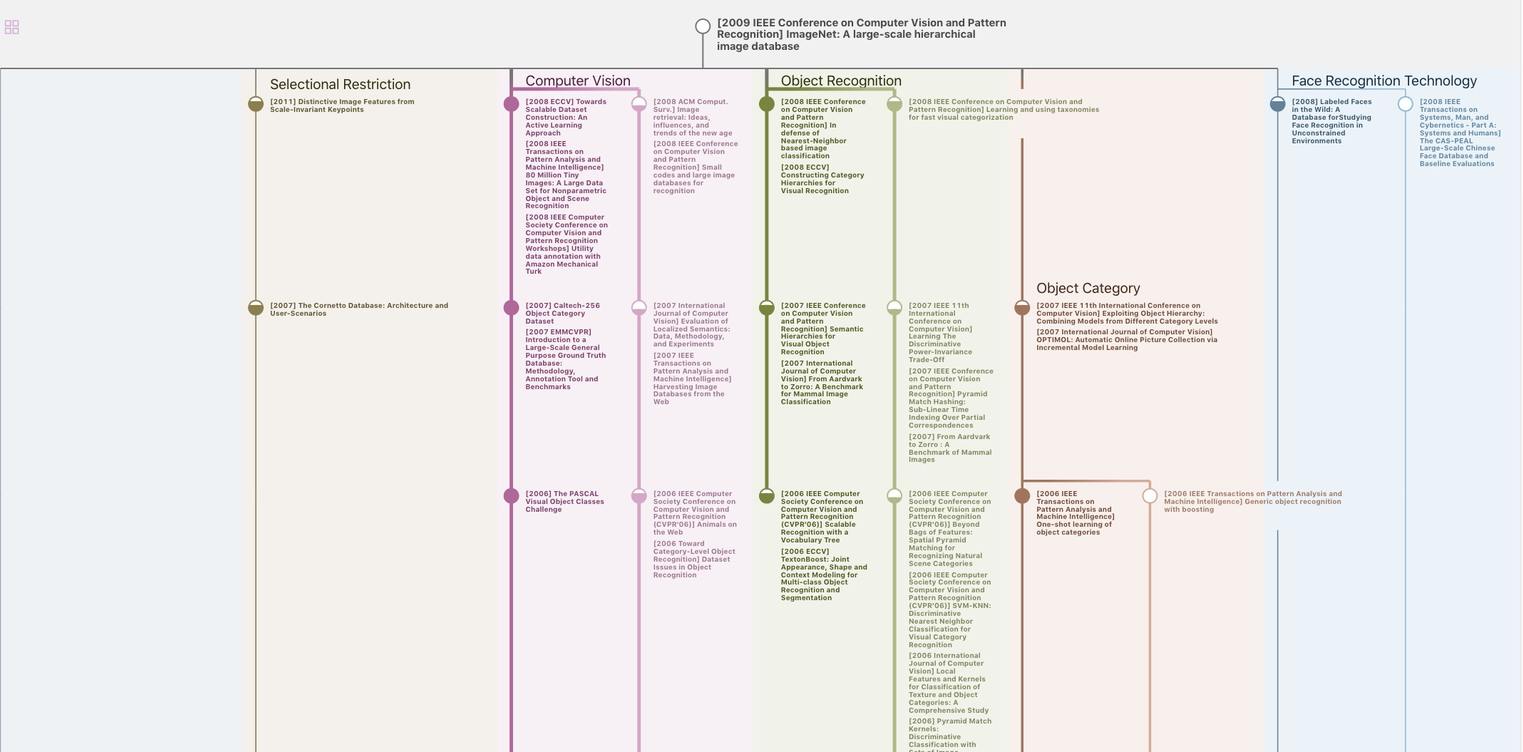
生成溯源树,研究论文发展脉络
Chat Paper
正在生成论文摘要