Connecting Neural Reconstruction Integrity (Nri) To Graph Metrics And Biological Priors
COMPLEX NETWORKS XI(2020)
摘要
We previously introduced the Neural Reconstruction Integrity (NRI) metric as a measure of how well the connectivity of the brain is measured in a neural circuit reconstruction, which can be represented as a graph or network. While powerful, NRI requires ground truth data for evaluation, which is conventionally obtained through time-intensive human annotation. NRI is a proxy for graph-based metrics since it focuses on the pre- and post-synaptic connections (or in and out edges) at a single neuron or vertex rather than overall graph structure in order to satisfy the format of available ground truth and provide rapid assessments. In this paper, we study the relationship between the NRI and graph theoretic metrics in order to understand the relationship of NRI to small world properties, centrality measures, and cost of information flow, as well as minimize our dependence on ground truth. The common errors under evaluation are synapse insertions and deletions and neuron splits and merges. We also elucidate the connection between graph metrics and biological priors for more meaningful interpretation of our results. We identified the most useful local metric to be local clustering coefficient, while the most useful global metrics are characteristic path length, rich-club coefficient, and density due to their strong correlations with NRI and perturbation errors.
更多查看译文
关键词
Neural Reconstruction Integrity, Graph metrics, Connectomics, Evaluation
AI 理解论文
溯源树
样例
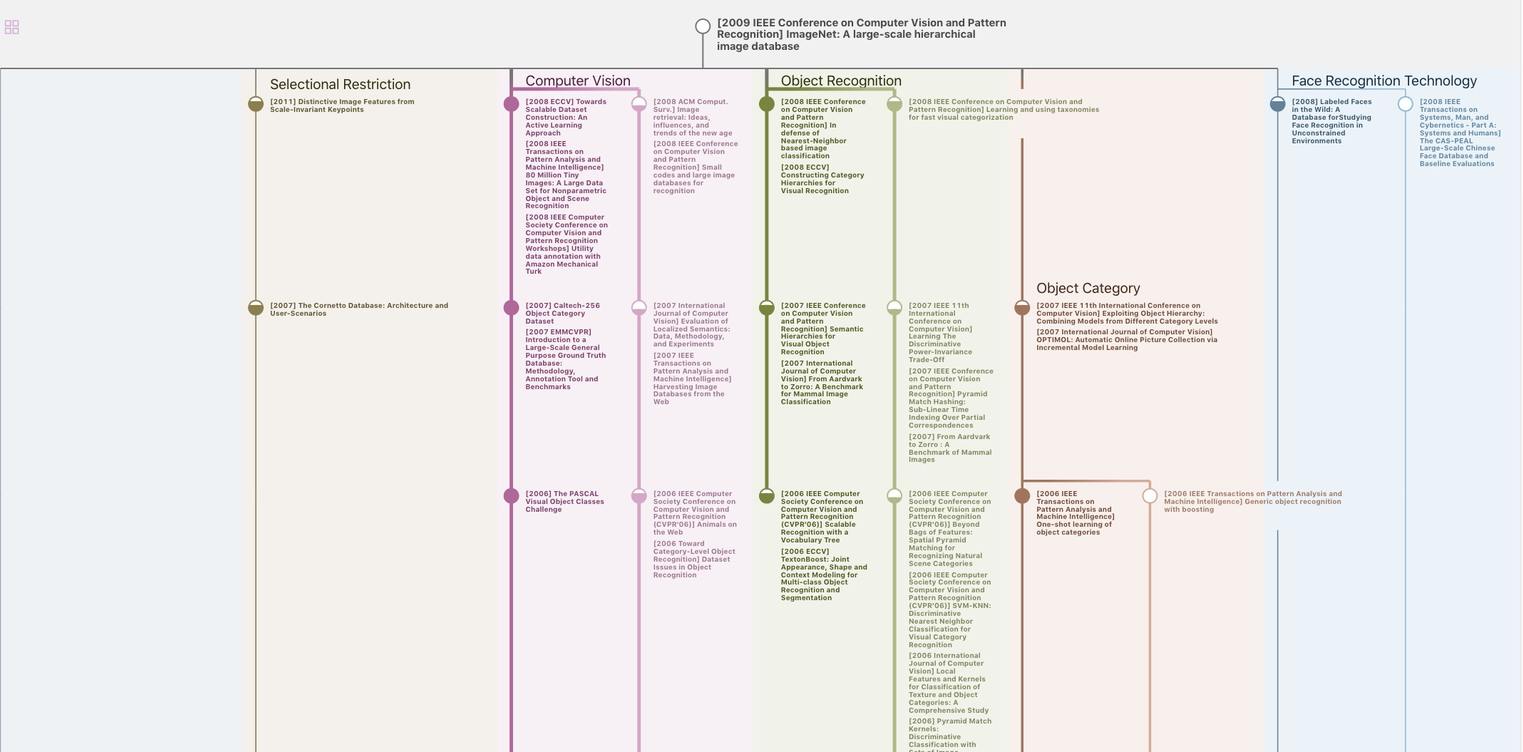
生成溯源树,研究论文发展脉络
Chat Paper
正在生成论文摘要