Class-dependent Pruning of Deep Neural Networks
2020 IEEE Second Workshop on Machine Learning on Edge in Sensor Systems (SenSys-ML)(2020)
摘要
Today’s deep neural networks require substantial computation resources for their training, storage and inference, which limits their effective use on resource-constrained devices. Many recent research activities explore different options for compressing and optimizing deep models. On the one hand, in many real-world applications we face the data imbalance challenge, i.e., when the number of labeled instances of one class considerably outweighs the number of labeled instances of the other class. On the other hand, applications may pose a class imbalance problem, i.e., higher number of false positives produced when training a model and optimizing its performance may be tolerable, yet the number of false negatives must stay low. The problem originates from the fact that some classes are more important for the application than others, e.g., detection problems in medical and surveillance domains. Motivated by the success of the lottery ticket hypothesis, in this paper we propose an iterative deep model compression technique, which keeps the number of false negatives of the compressed model close to the one of the original model at the price of increasing the number of false positives if necessary. Our experimental evaluation using two benchmark data sets shows that the resulting compressed sub-networks 1) achieve up to 35% lower number of false negatives than the compressed model without class optimization, 2) provide an overall higher AUC-ROC measure compared to conventional Lottery Ticket algorithm and three recent popular pruning methods, and 3) use up to 99% fewer parameters compared to the original network. The code is publicly available
1
.
1
https://github.com/rahimentezari/Sensys-ml2020
更多查看译文
关键词
deep neural network compression,pruning,lottery ticket hypothesis,data imbalance,class imbalance
AI 理解论文
溯源树
样例
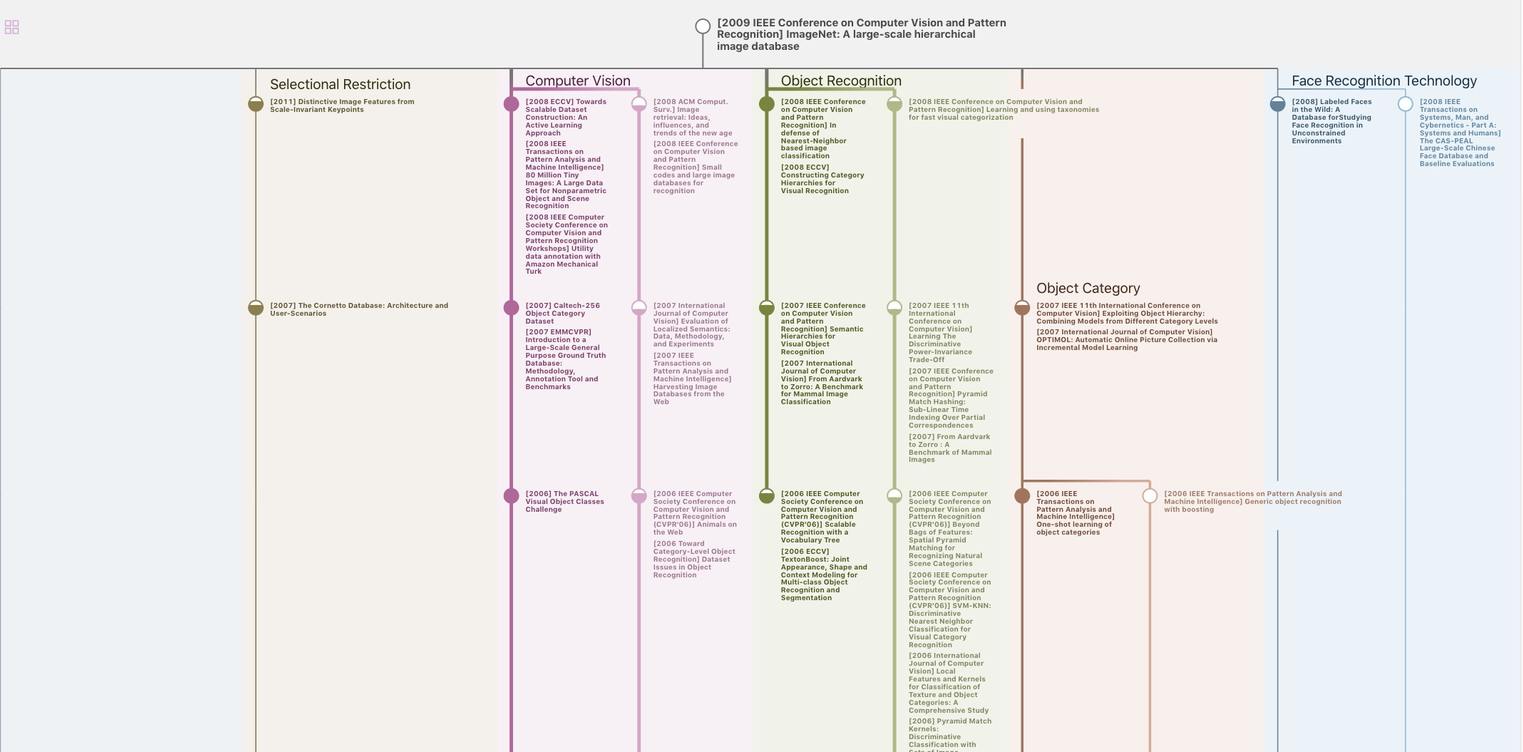
生成溯源树,研究论文发展脉络
Chat Paper
正在生成论文摘要