Error Bounding For Araim Integrity Support Message Generation
PROCEEDINGS OF THE 33RD INTERNATIONAL TECHNICAL MEETING OF THE SATELLITE DIVISION OF THE INSTITUTE OF NAVIGATION (ION GNSS+ 2020)(2020)
摘要
The Advanced RAIM (ARAIM) concept aims to provide global integrity service to Safety-of-Life (SoL) users. The safety is ensured through a robust user algorithms and the use of an Integrity Support Message (ISM) containing information on the GNSS constellations performance. An external entity, the so-called ISM Generator (ISMG), is in charge of monitoring the performance of the constellations and broadcast updated and validated information to the user.This paper provides an overview of the ISM generation architecture and processing developed in the project ARTEX of the European Commission. In this project an ISMG Algorithm Description Document was developed and will be presented in this paper. The focus is in particular on two aspects, for which ad-hoc solutions were developed.The first part presents method to bound the Signal In Space ranging errors. The problem of assessing the confidence of the bounding and the number of independent samples was addressed using a solution based on spectrum analysis. This approach allows to take into account the temporal correlation of the data when assessing confidence margins without increasing significantly the ISM parameters. Besides the bounding needs to be valid for any type of distribution including asymmetric and not unimodal ones. For these distributions, problems raise when combining several ranging bounds, that is after the convolution of different bounding distributions. A method was developed to compute the bias of the gaussian bounding distribution, the so called BNOM, and ensure that the bounding is valid in all conditions. The method was validated processing Galileo real data from its Initial Service (December 2016). In comparison with other bounding methodologies, this approach provides reduced ISM parameters and therefore improves the user availability.Secondly a high update rate monitoring method is described based on the processing of observations collected every 30 seconds and complementing the method based on reference products collected every 5 minutes. Results show how this method can improve the accuracy of the estimation of the fault magnitude, the fault onset and the identification of the anomaly source. In addition, it is able to capture faults with short durations, as for example signal distortions, phase fluctuations, flex power spikes, which cannot be properly detected with 5 minutes sp3 products. If and when needed, additional contributions are included in the ISM parameters using this additional monitoring.
更多查看译文
AI 理解论文
溯源树
样例
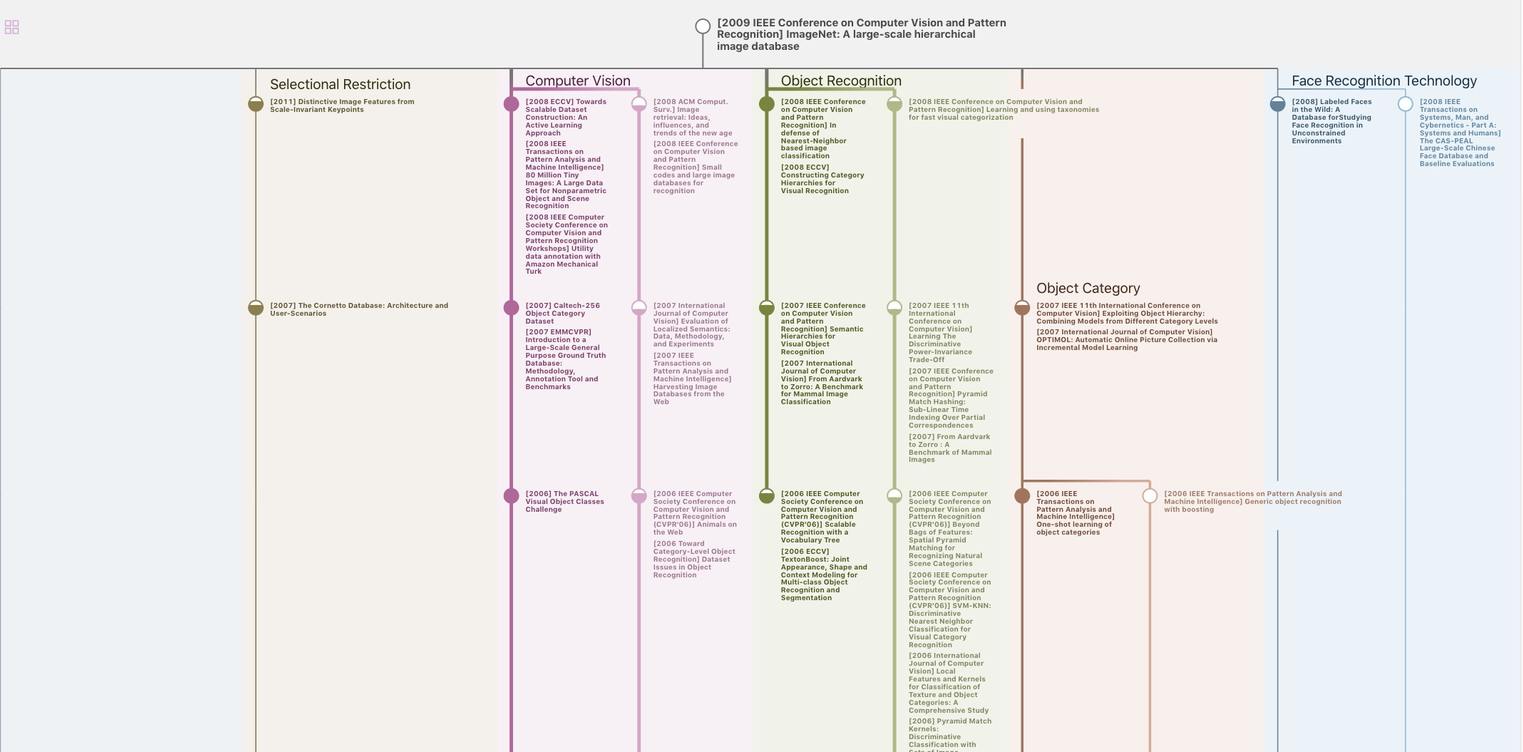
生成溯源树,研究论文发展脉络
Chat Paper
正在生成论文摘要