Structured Receptive Field Networks And Applications To Hyperspectral Image Classification
WAVELETS AND SPARSITY XVIII(2019)
摘要
Deep neural networks have achieved impressive performance in problems of object detection and object category classifications. To perform efficiently though, such methods typically require a large number of training samples. Unfortunately, this requirement is highly impractical or impossible in applications such as hyperspectral classification where it is expensive and labor intensive to generate labeled data for training. A few ideas have been proposed in the literature to address this problem such as transfer learning and domain adaptation. In this work, we propose an alternative strategy to reduce the number of network parameters based on Structured Receptive Field Networks (SRFN), a class of convolutional neural networks (CNNs) where each convolutional filter is a linear combination from a predefined dictionary. To better exploit the characteristics of hyperspectral data to be learned, we choose a filter dictionary consisting of directional filters inspired by the theory of shearlets and we train a SRFN by imposing that the convolutional filters form sparse linear combinations in such dictionary. The application of our SRFN to problems of hyperspectral classification shows that this approach achieves very competitive performance as compared to conventional CNNs.
更多查看译文
关键词
Hyperspectral data, convolutional neural networks, deep learning, multiscale analysis
AI 理解论文
溯源树
样例
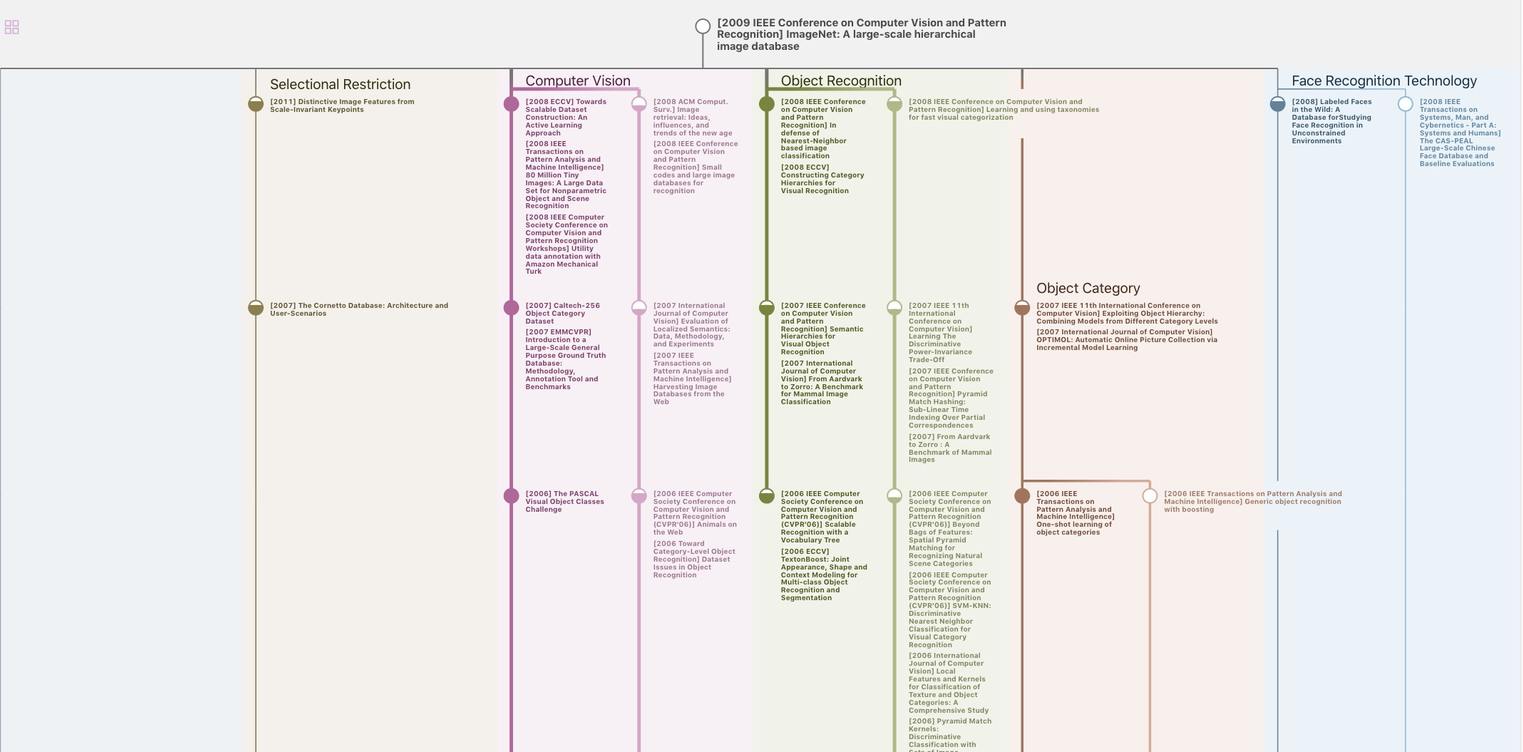
生成溯源树,研究论文发展脉络
Chat Paper
正在生成论文摘要