Fast Multi-Modal Reuse: Co-Occurrence Pre-Trained Deep Learning Models
REAL-TIME IMAGE PROCESSING AND DEEP LEARNING 2019(2019)
摘要
The purpose of this paper is to study data fusion applications in traditional, spatial, and aerial video stream applications which addresses the processing of data from multiple sources using co-occurrence information and uses a common semantic metric. Use of co-occurrence information to infer semantic relations between measurements avoids the need to make use of such external information, such as labels. Many of the current Vector Space Models (VSM) do not preserve the co-occurrence information, leading to a less than useful similarity metric. We propose a proximity matrix embedding part of the learning metric representation which has entries showing the relations between co-occurrence frequency observed in input sets. First, we show an implicit spatial sensor proximity matrix calculation using Jaccard similarity for an array of sensor measurements and compare with the state-of-the-art kernel PCA learning from feature space proximity representation; it relates to a k-radius ball of nearest neighbors. Finally, we extend the class co-occurrence boosting of our unsupervised model using pre-trained multi-modal reuse.
更多查看译文
关键词
Sensor Selection, Semantic Hashing, Autoencoder, Jaccard Similarity, Minhashing
AI 理解论文
溯源树
样例
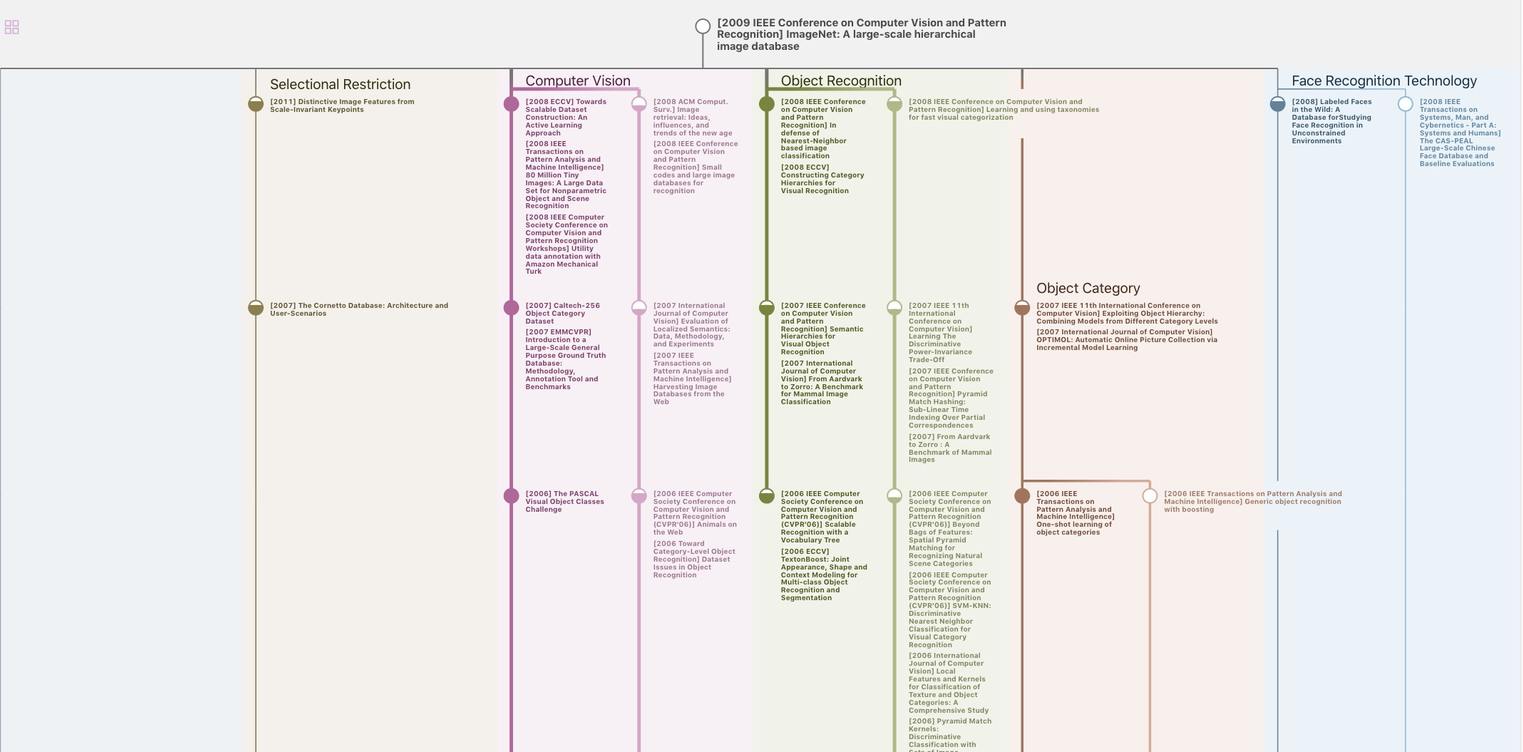
生成溯源树,研究论文发展脉络
Chat Paper
正在生成论文摘要