An Empirical Investigation On A Multiple Filters-Based Approach For Remaining Useful Life Prediction
MACHINES(2018)
摘要
Feature construction is critical in data-driven remaining useful life (RUL) prediction of machinery systems, and most previous studies have attempted to find a best single-filter method. However, there is no best single filter that is appropriate for all machinery systems. In this work, we devise a straightforward but efficient approach for RUL prediction by combining multiple filters and then reducing the dimension through principal component analysis. We apply multilayer perceptron and random forest methods to learn the underlying model. We compare our approach with traditional single-filtering approaches using two benchmark datasets. The former approach is significantly better than the latter in terms of a scoring function with a penalty for late prediction. In particular, we note that selecting a best single filter over the training set is not efficient because of overfitting. Taken together, we validate that our multiple filters-based approach can be a robust solution for RUL prediction of various machinery systems.
更多查看译文
关键词
prognostics, remaining useful life, feature construction, multiple filters, data-driven prediction, machine learning, overfitting, principal component analysis
AI 理解论文
溯源树
样例
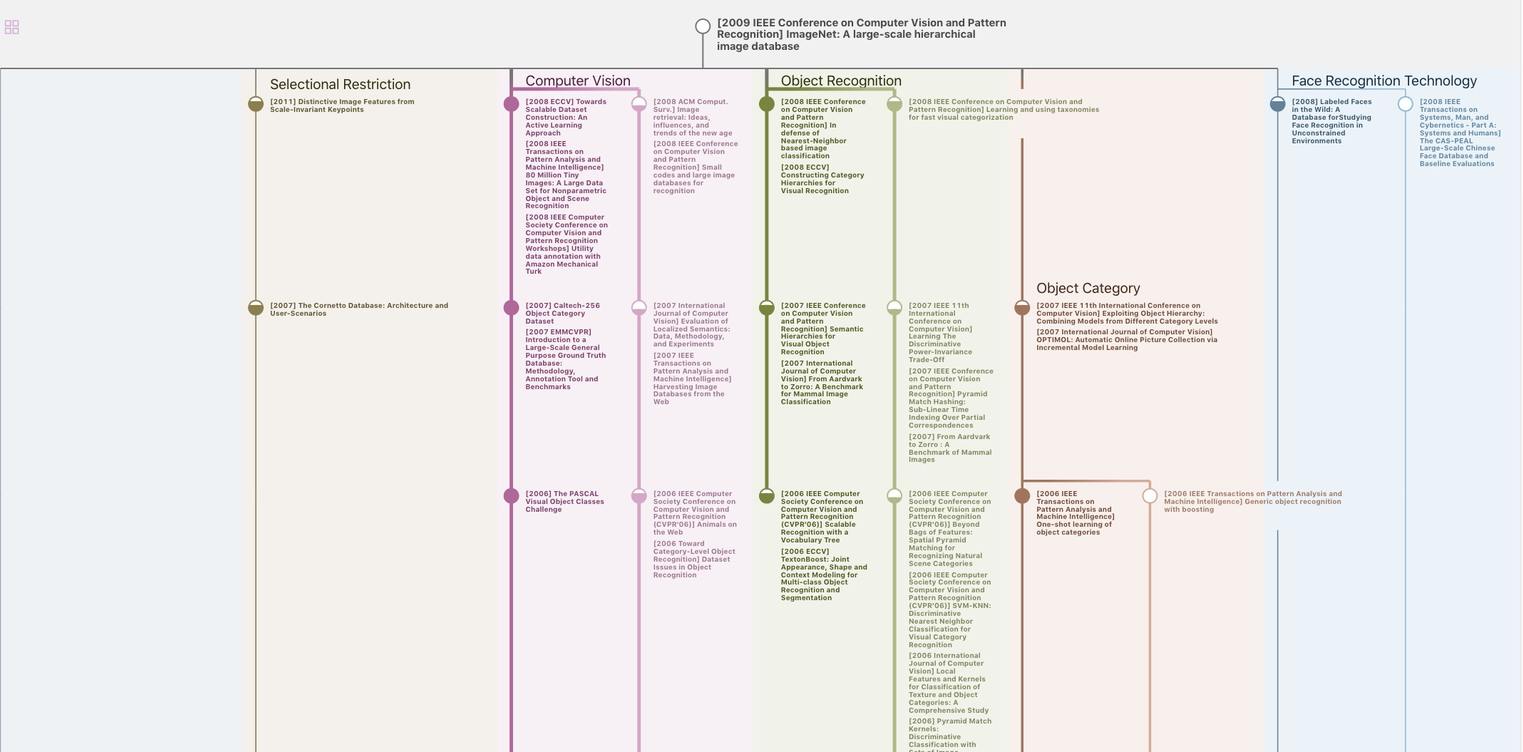
生成溯源树,研究论文发展脉络
Chat Paper
正在生成论文摘要