Fine-Grained Comparisons With Attributes
VISUAL ATTRIBUTES(2017)
摘要
Given two images, we want to predict which exhibits a particular visual attribute more than the other-even when the two images are quite similar. For example, given two beach scenes, which looks more calm? Given two high-heeled shoes, which is more ornate? Existing relative attribute methods rely on global ranking functions. However, rarely will the visual cues relevant to a comparison be constant for all data, nor will humans' perception of the attribute necessarily permit a global ordering. At the same time, not every image pair is even orderable for a given attribute. Attempting to map relative attribute ranks to "equality" predictions is nontrivial, particularly since the span of indistinguishable pairs in attribute space may vary in different parts of the feature space. To address these issues, we introduce local learning approaches for fine-grained visual comparisons, where a predictive model is trained on the fly using only the data most relevant to the novel input. In particular, given a novel pair of images, we develop local learning methods to (1) infer their relative attribute ordering with a ranking function trained using only analogous labeled image pairs, (2) infer the optimal "neighborhood," i.e., the subset of the training instances most relevant for training a given local model, and (3) infer whether the pair is even distinguishable, based on a local model for just noticeable differences in attributes. Our methods outperform state-of-the-art methods for relative attribute prediction on challenging datasets, including a large newly curated shoe dataset for fine-grained comparisons. We find that for fine-grained comparisons, more labeled data is not necessarily preferable to isolating the right data.
更多查看译文
AI 理解论文
溯源树
样例
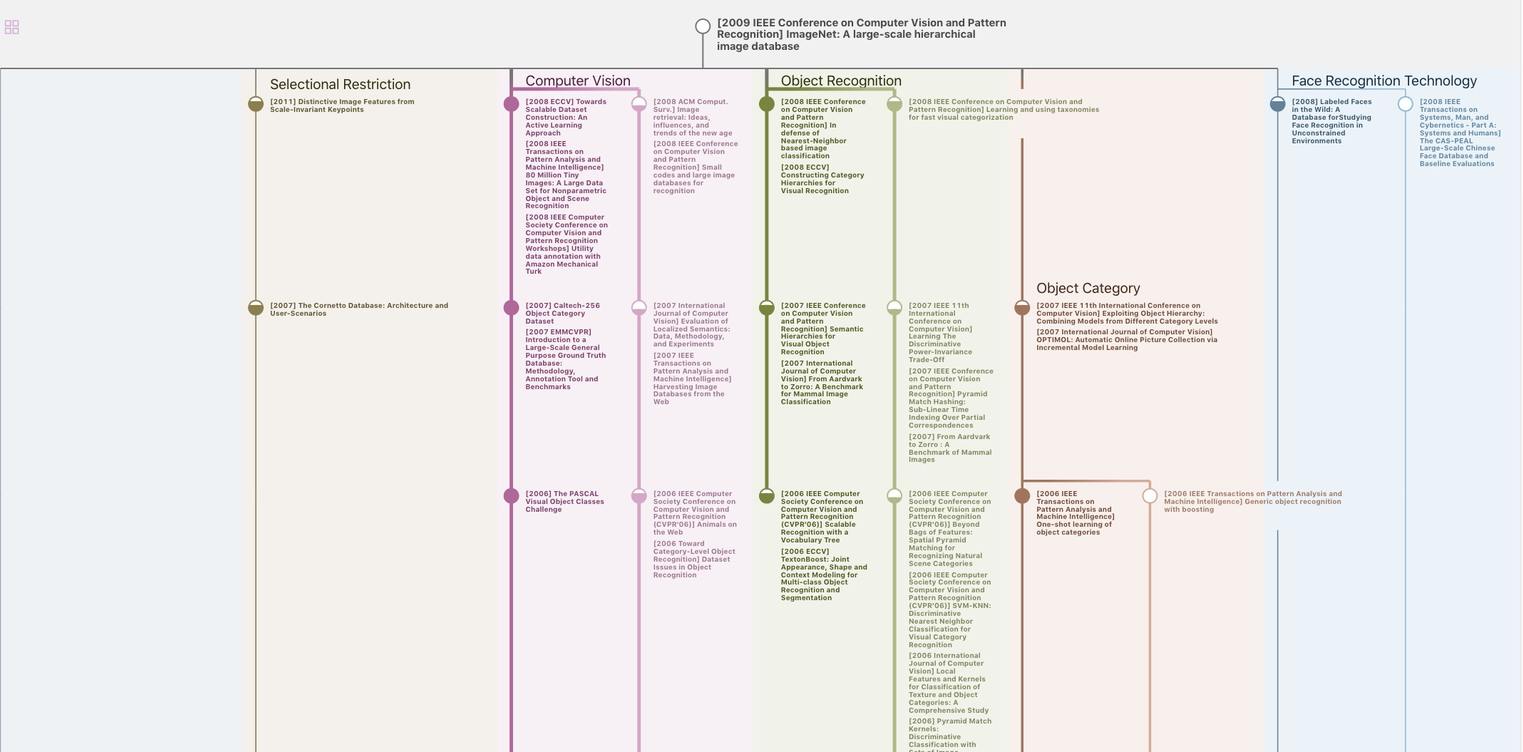
生成溯源树,研究论文发展脉络
Chat Paper
正在生成论文摘要