Contrasting Conventional And Machine Learning Approaches To Optical Critical Dimension Measurements
METROLOGY, INSPECTION, AND PROCESS CONTROL FOR MICROLITHOGRAPHY XXXIV(2020)
摘要
Accurate, optics-based measurement of feature sizes at deep sub-wavelength dimensions has been conventionally challenged by improved manufacturing, including smaller linewidths, denser layouts, and greater materials complexity at near-atomic scales. Electromagnetic modeling is relied upon heavily for forward maps used to solve the inverse problem of optical measurements for parametric estimation. Machine learning (ML) approaches are continually under consideration, either as a means to bypass direct comparison to simulation or as a method to augment nonlinear regression. In this work, ML approaches are investigated using a well-characterized experimental data set and its simulation library that assumes a 2-D geometry. The benefits and limitations of ML for optical critical dimension (OCD) metrology are illustrated by comparing a straightforward library lookup method and two ML approaches, a data-driven surrogate model for nonlinear regression using radial basis functions (RBF) and multiple-output Gaussian process regression (GPR) that indirectly applies the simulated intensity data. Both RBF and GPR generally improve accuracy over the conventional method with as few as 32 training points. However, as measurement noise is decreased the uncertainties from RBF and GPR differ greatly as the GPR posterior estimate of the variance appears to overestimate parametric uncertainties. Both accuracy and uncertainty must be addressed in OCD while balancing simulation versus ML computational requirements.
更多查看译文
AI 理解论文
溯源树
样例
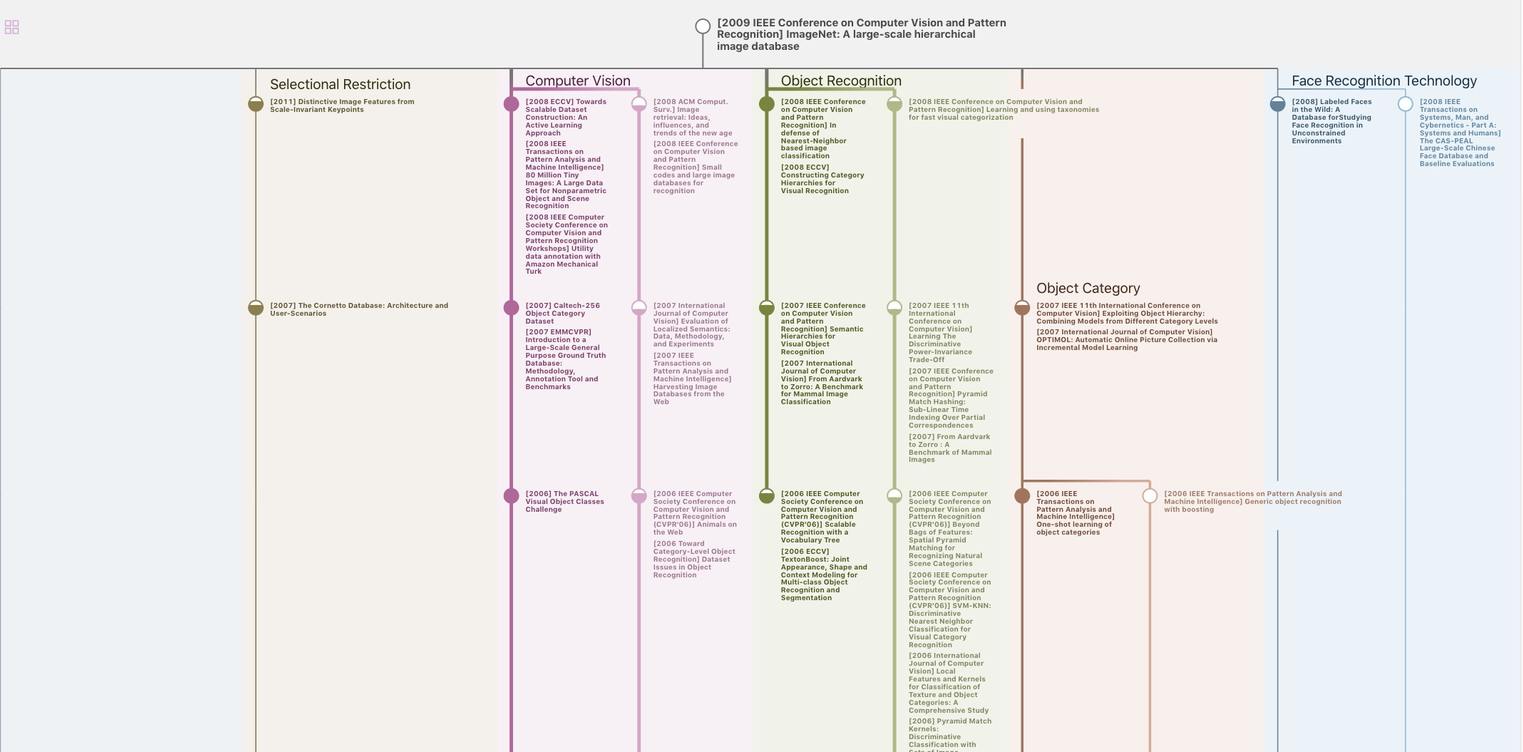
生成溯源树,研究论文发展脉络
Chat Paper
正在生成论文摘要