Using Repeated Measurements to Predict Cardiovascular Risk in Patients With Type 2 Diabetes Mellitus
AMERICAN JOURNAL OF CARDIOLOGY(2024)
摘要
The QRISK cardiovascular disease (CVD) risk assessment model is not currently optimized for patients with type 2 diabetes mellitus (T2DM). We aim to identify if the abundantly available repeatedly measured data for patients with T2D improves the predictive capability of QRISK to support the decision-making process regarding CVD prevention in patients with T2DM. We identified patients with T2DM aged 25 to 85, not on statin treatment and without pre-existing CVD from the IQVIA Medical Research Data United Kingdom primary care database and then followed them up until the first diagnosis of CVD, ischemic heart disease, or stroke/transient ischemic attack. We included traditional, nontraditional risk factors and relevant treatments for our analysis. We then undertook a Cox's hazards model accounting for time-dependent covariates to estimate the hazard rates for each risk factor and calculated a 10-year risk score. Models were developed for males and females separately. We tested the performance of our models using validation data and calculated discrimination and calibration statistics. The study included 198,835 (180,143 male with 11,976 outcomes and 90,466 female with 8,258 outcomes) patients. The 10-year predicted survival probabilities for females was 0.87 (0.87 to 0.87), whereas the observed survival estimates from the Kaplan-Meier curve for all female models was 0.87 (0.86 to 0.87). The predicted and observed survival estimates for males were 0.84 (0.84 to 0.84) and 0.84 (0.83 to 0.84) respectively. The Harrell's C-index of all female models and all male models were 0.71 and 0.69 respectively. We found that including time-varying repeated measures, only mildly improved CVD risk prediction for T2DM patients in comparison to the current practice standard. We advocate for further research using time-varying data to identify if the involvement of further covariates may improve the accuracy of currently accepted prediction models.(c) 2023 The Author(s). Published by Elsevier Inc. This is an open access article under the CC BY license (http://creativecommons.org/ licenses/by/4.0/) (Am J Cardiol 2024;210:133-142)
更多查看译文
关键词
cardiovascular risk,epidemiology,extended Cox-proportional hazards regression,repeatedly measured data,time-varying co-variates,type 2 diabetes
AI 理解论文
溯源树
样例
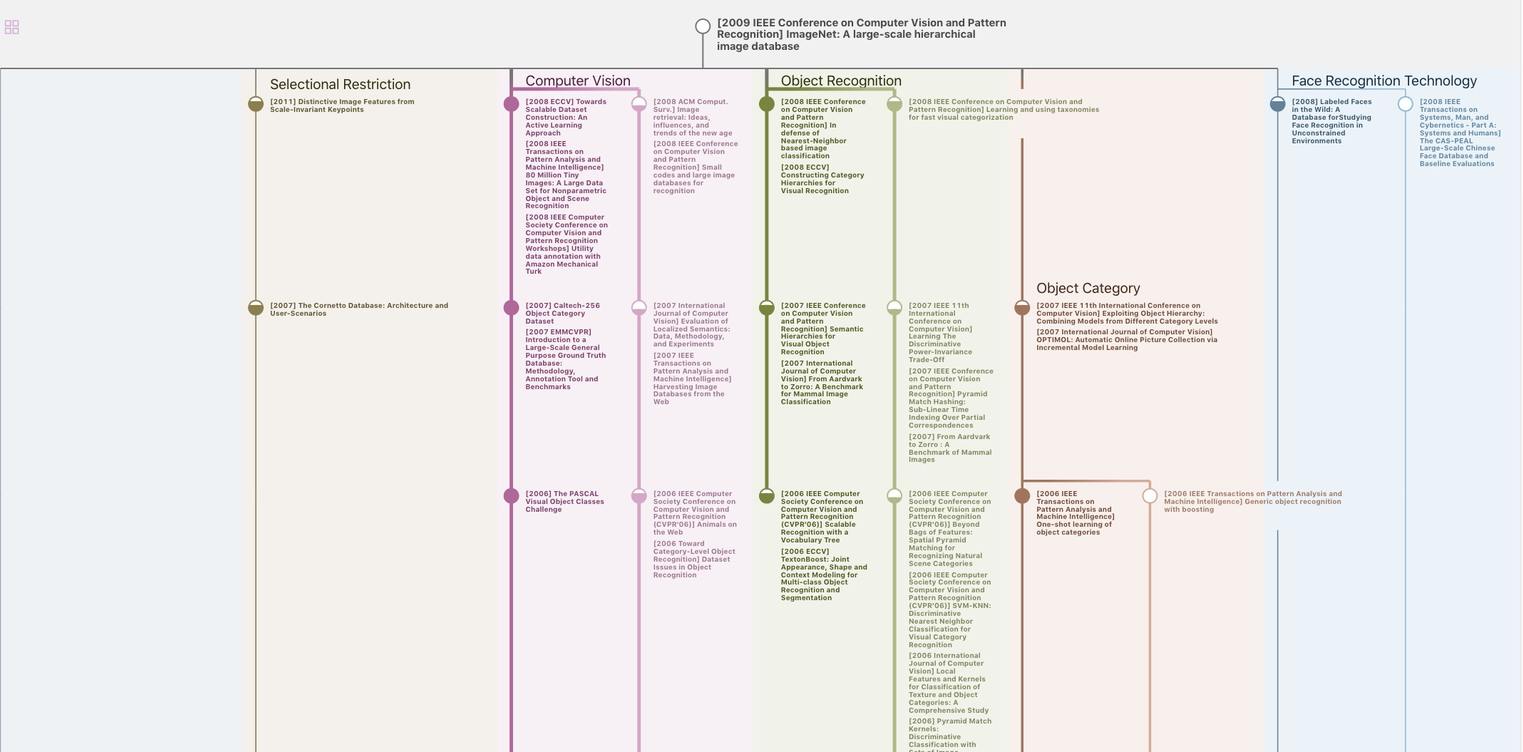
生成溯源树,研究论文发展脉络
Chat Paper
正在生成论文摘要