A comprehensive texture feature analysis framework of renal cell carcinoma: pathological, prognostic, and genomic evaluation based on CT images
European Radiology(2021)
摘要
Objectives We tried to realize accurate pathological classification, assessment of prognosis, and genomic molecular typing of renal cell carcinoma by CT texture feature analysis. To determine whether CT texture features can perform accurate pathological classification and evaluation of prognosis and genomic characteristics in renal cell carcinoma. Methods Patients with renal cell carcinoma from five open-source cohorts were analyzed retrospectively in this study. These data were randomly split to train and test machine learning algorithms to segment the lesion, predict the histological subtype, tumor stage, and pathological grade. Dice coefficient and performance metrics such as accuracy and AUC were calculated to evaluate the segmentation and classification model. Quantitative decomposition of the predictive model was conducted to explore the contribution of each feature. Besides, survival analysis and the statistical correlation between CT texture features, pathological, and genomic signatures were investigated. Results A total of 569 enhanced CT images of 443 patients (mean age 59.4, 278 males) were included in the analysis. In the segmentation task, the mean dice coefficient was 0.96 for the kidney and 0.88 for the cancer region. For classification of histologic subtype, tumor stage, and pathological grade, the model was on a par with radiologists and the AUC was 0.83 ± 0.1, 0.80 ± 0.1, and 0.77 ± 0.1 at 95
更多查看译文
关键词
Imaging genomics,Prognosis,Latent class analysis,Carcinoma,Renal cell
AI 理解论文
溯源树
样例
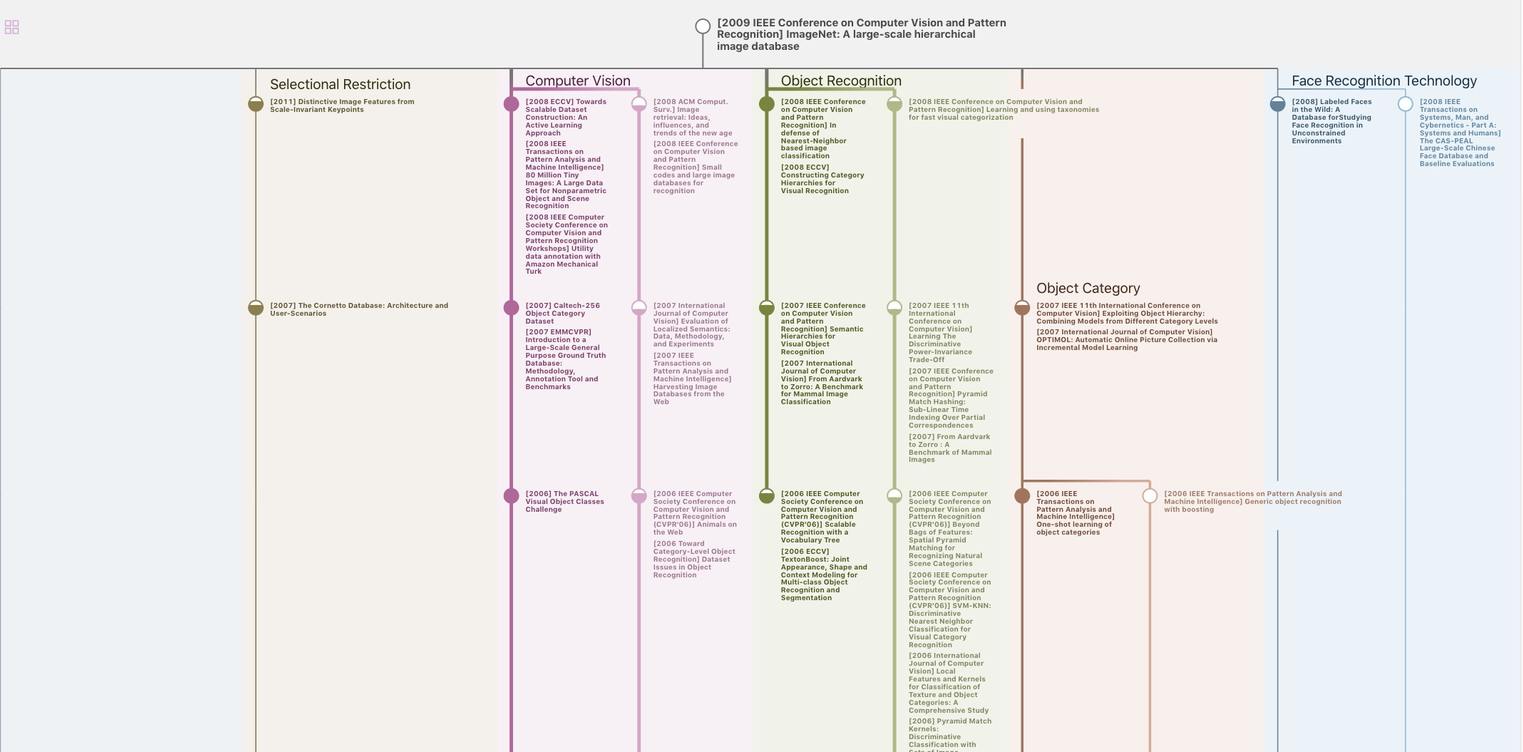
生成溯源树,研究论文发展脉络
Chat Paper
正在生成论文摘要