Deciphering the language of antibodies using self-supervised learning
Patterns(2022)
摘要
An individual’s B cell receptor (BCR) repertoire encodes information about past immune responses and potential for future disease protection. Deciphering the information stored in BCR sequence datasets will transform our understanding of disease and enable discovery of novel diagnostics and antibody therapeutics. A key challenge of BCR sequence analysis is the prediction of BCR properties from their amino acid sequence alone. Here, we present an antibody-specific language model, Antibody-specific Bidirectional Encoder Representation from Transformers (AntiBERTa), which provides a contextualized representation of BCR sequences. Following pre-training, we show that AntiBERTa embeddings capture biologically relevant information, generalizable to a range of applications. As a case study, we fine-tune AntiBERTa to predict paratope positions from an antibody sequence, outperforming public tools across multiple metrics. To our knowledge, AntiBERTa is the deepest protein-family-specific language model, providing a rich representation of BCRs. AntiBERTa embeddings are primed for multiple downstream tasks and can improve our understanding of the language of antibodies.
更多查看译文
关键词
B cell receptors,antibodies,representation learning,self-supervised learning,transfer learning,language models,paratope prediction,transformers
AI 理解论文
溯源树
样例
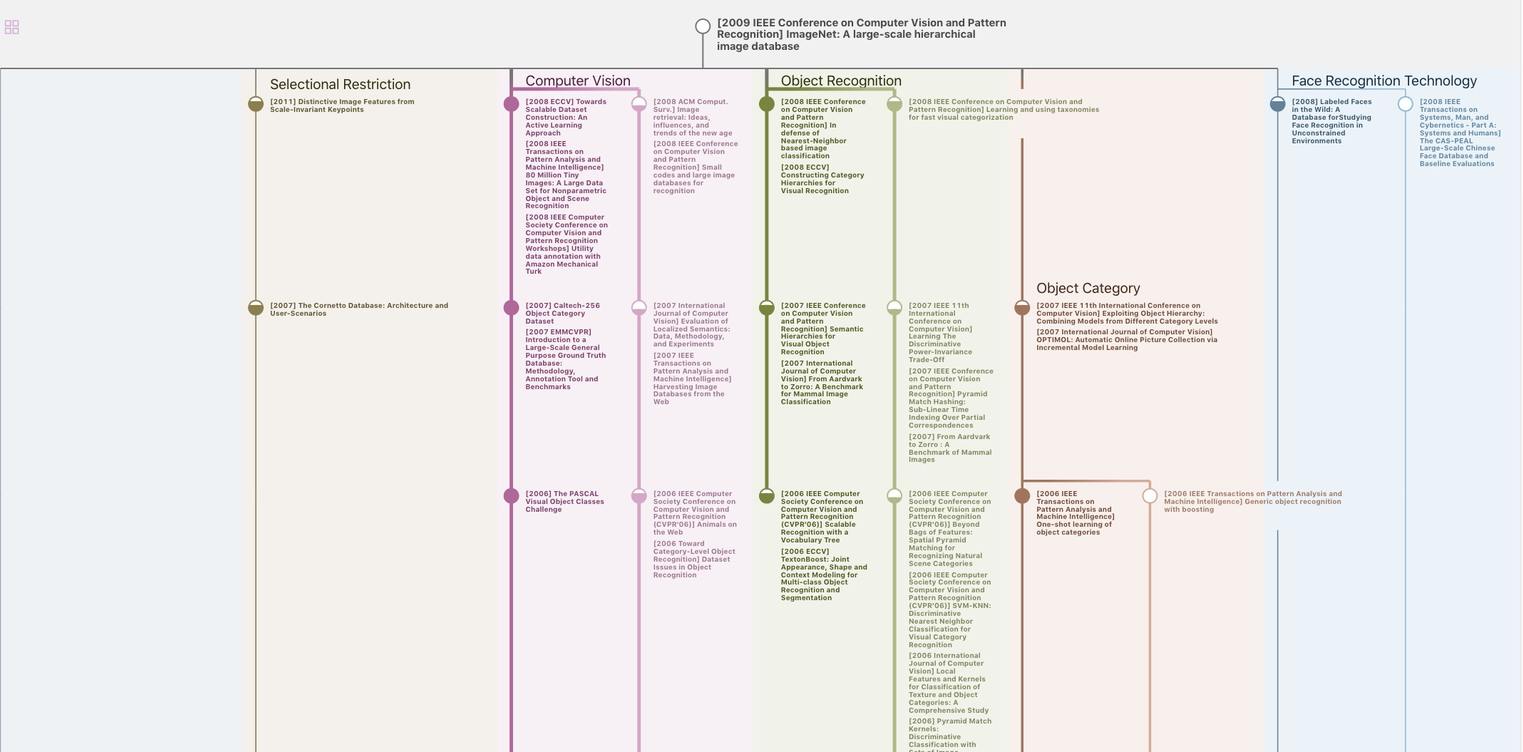
生成溯源树,研究论文发展脉络
Chat Paper
正在生成论文摘要