Bayesian mixture model for clustering rare-variant effects in human genetic studies
biorxiv(2021)
摘要
Rare-variant aggregate analysis from exome and whole genome sequencing data typically summarizes with a single statistic the signal for a gene or the unit that is being aggregated. However, when doing so, the effect profile within the unit may not be easily characterized across one or multiple phenotypes. Here, we present an approach we call Multiple Rarevariants and Phenotypes Mixture Model (MRPMM), which clusters rare variants into groups based on their effects on the multivariate phenotype and makes statistical inferences about the properties of the underlying mixture of genetic effects. Using summary statistic data from a meta-analysis of exome sequencing data of 184,698 individuals in the UK Biobank across 6 populations, we demonstrate that our mixture model can identify clusters of variants responsible for significantly disparate effects across a multivariate phenotype; we study three lipid and three renal traits separately. The method is able to estimate (1) the proportion of non-null variants, (2) whether variants with the same predicted consequence in one gene behave similarly, (3) whether variants across genes share effect profiles across the multivariate phenotype, and (4) whether different annotations differ in the magnitude of their effects. As rare-variant data and aggregation techniques become more common, this method can be used to ascribe further meaning to association results.
### Competing Interest Statement
M.A.R. is on the SAB of 54Gene, Related Sciences and scientific founder of Broadwing Bio and has advised BioMarin, Third Rock Ventures and MazeTx.
更多查看译文
AI 理解论文
溯源树
样例
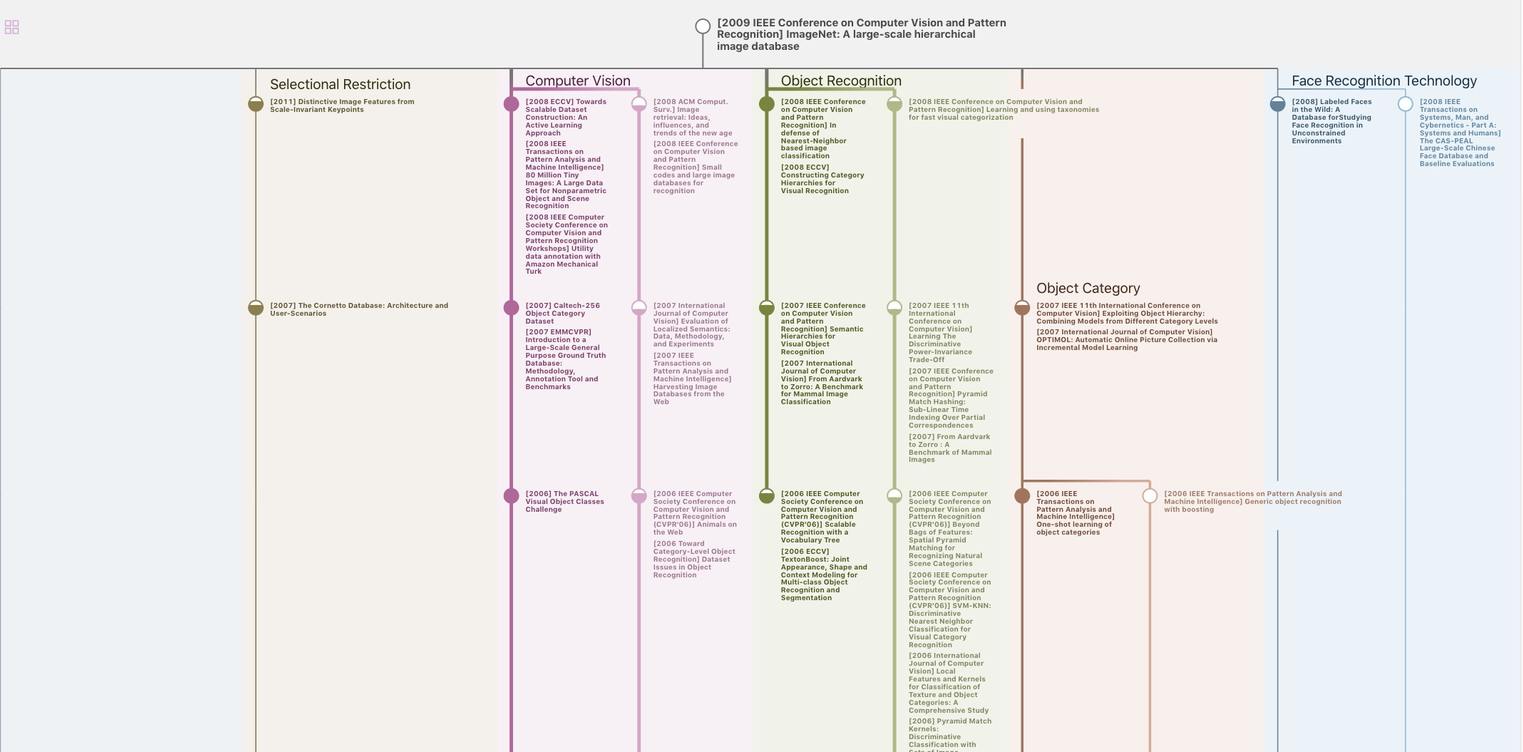
生成溯源树,研究论文发展脉络
Chat Paper
正在生成论文摘要