Logical modeling: Combining manual curation and automated parameterization to predict drug synergies
biorxiv(2021)
摘要
Treatment with drug combinations carries great promise for personalized therapy. We have previously shown that drug synergies targeting cancer can manually be identified based on a logical framework. We now demonstrate how automated adjustments of model topology and logic equations can greatly reduce the workload traditionally associated with logical model optimization. Our methodology allows the exploration of larger model ensembles that all obey a set of observations. We benchmark synergy predictions against a dataset of 153 targeted drug combinations. We show that well-performing manual models faithfully represent measured biomarker data and that their performance can be outmatched by automated parameterization using a genetic algorithm. The predictive performance of a curated model is strongly affected by simulated curation errors, while data-guided deletion of a small subset of edges can improve prediction quality. With correct topology we find some tolerance to simulated errors in the biomarker calibration data. With our framework we predict the synergy of joint inhibition of PI3K and TAK1, and further substantiate this prediction with observation in cancer cell cultures and in xenograft experiments.
更多查看译文
关键词
drug synergies,logical modeling,manual curation
AI 理解论文
溯源树
样例
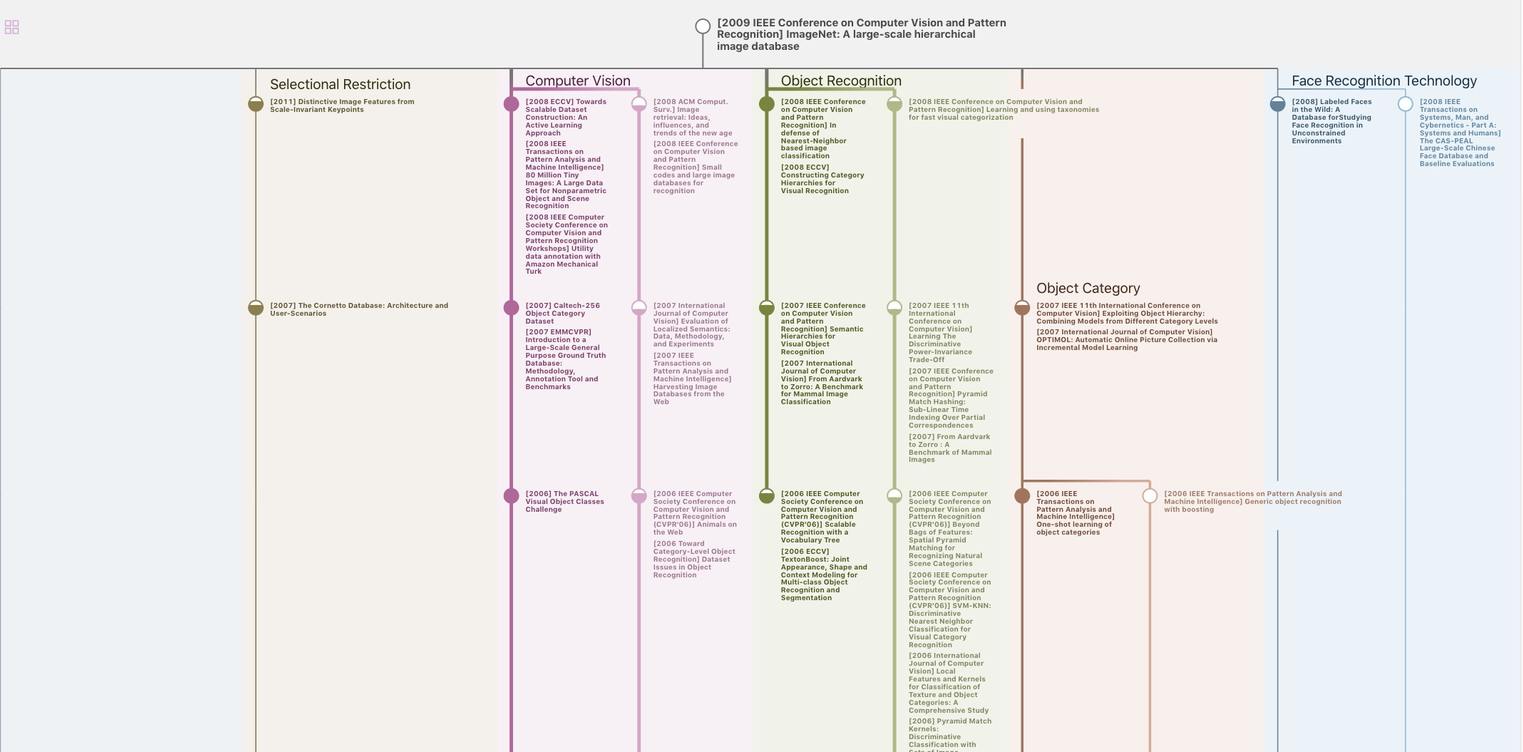
生成溯源树,研究论文发展脉络
Chat Paper
正在生成论文摘要