Whole-cell segmentation of tissue images with human-level performance using large-scale data annotation and deep learning
bioRxiv (Cold Spring Harbor Laboratory)(2021)
摘要
Understanding the spatial organization of tissues is of critical importance for both basic and translational research. While recent advances in tissue imaging are opening an exciting new window into the biology of human tissues, interpreting the data that they create is a significant computational challenge. Cell segmentation, the task of uniquely identifying each cell in an image, remains a substantial barrier for tissue imaging, as existing approaches are inaccurate or require a substantial amount of manual curation to yield useful results. Here, we addressed the problem of cell segmentation in tissue imaging data through large-scale data annotation and deep learning. We constructed TissueNet, an image dataset containing >1 million paired whole-cell and nuclear annotations for tissue images from nine organs and six imaging platforms. We created Mesmer, a deep learning-enabled segmentation algorithm trained on TissueNet that performs nuclear and whole-cell segmentation in tissue imaging data. We demonstrated that Mesmer has better speed and accuracy than previous methods, generalizes to the full diversity of tissue types and imaging platforms in TissueNet, and achieves human-level performance for whole-cell segmentation. Mesmer enabled the automated extraction of key cellular features, such as subcellular localization of protein signal, which was challenging with previous approaches. We further showed that Mesmer could be adapted to harness cell lineage information present in highly multiplexed datasets. We used this enhanced version to quantify cell morphology changes during human gestation. All underlying code and models are released with permissive licenses as a community resource.
### Competing Interest Statement
M.A. is an inventor on patent US20150287578A1. M.A. is a board member and shareholder in IonPath Inc. T.R. has previously consulted for IonPath Inc. The authors have filed a provisional patent for this work.
更多查看译文
关键词
tissue images,segmentation,deep learning,whole-cell,human-level,large-scale
AI 理解论文
溯源树
样例
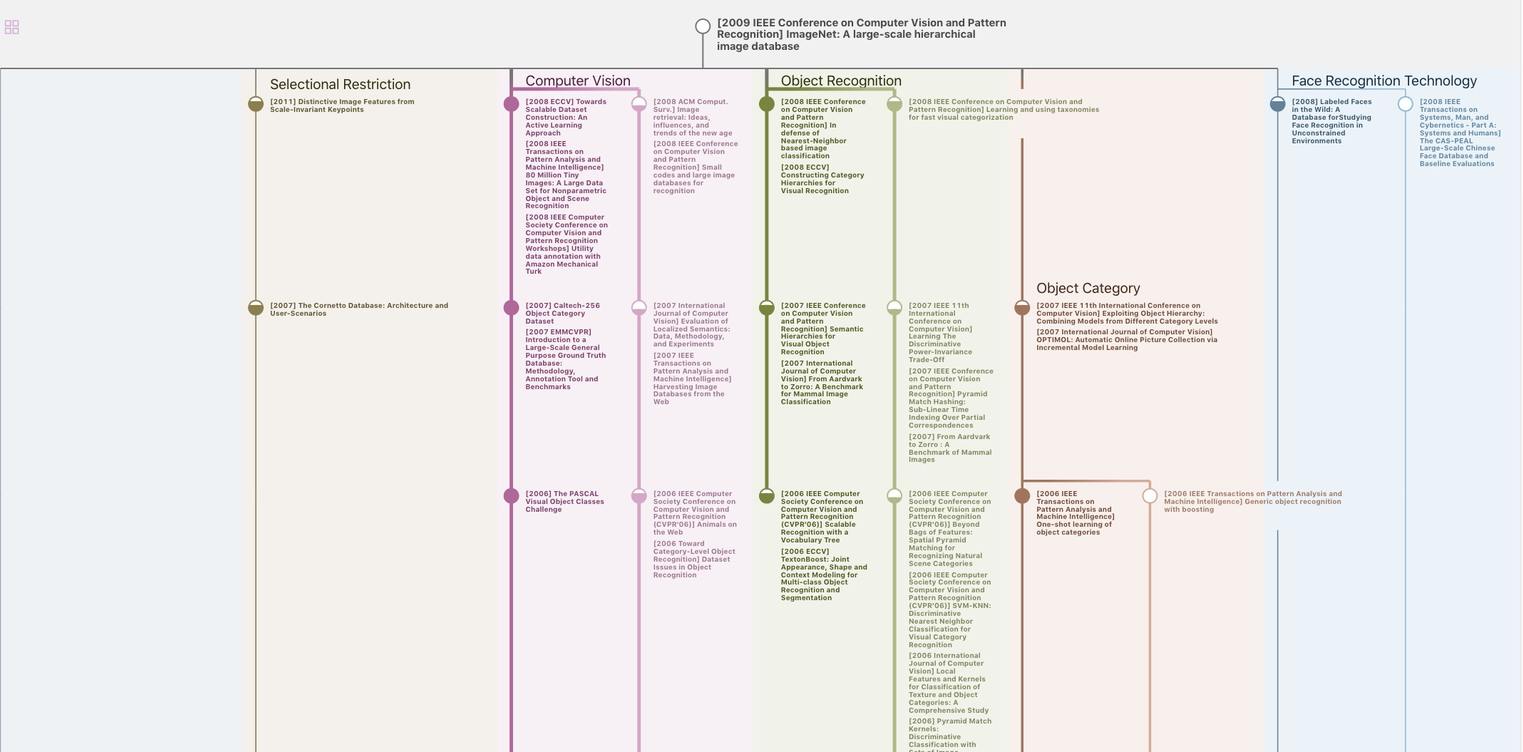
生成溯源树,研究论文发展脉络
Chat Paper
正在生成论文摘要