Membership Inference Attacks against GANs by Leveraging Over-representation Regions
Computer and Communications Security(2021)
摘要
ABSTRACTGenerative adversarial networks (GANs) have made unprecedented performance in image synthesis and play a key role in various downstream applications of computer vision. However, GAN models trained on sensitive data also pose a distinct threat to privacy. In this poster, we present a novel over-representation based membership inference attack. Unlike prior attacks against GANs which focus on the overall metrics, such as the attack accuracy, our attack aims to make inference from the high-precision perspective, which allows the adversary to concentrate on inferring a sample as a member confidently. Initial experimental results demonstrate that the adversary can achieve a high precision attack even if the overall attack accuracy is about 50% for a well-trained GAN model. Our work will raise awareness of the importance of precision when GAN owners evaluate the privacy risks of their models.
更多查看译文
关键词
Membership Inference Attacks, Generative Adversarial Networks, Human Face Generation, Over-representation
AI 理解论文
溯源树
样例
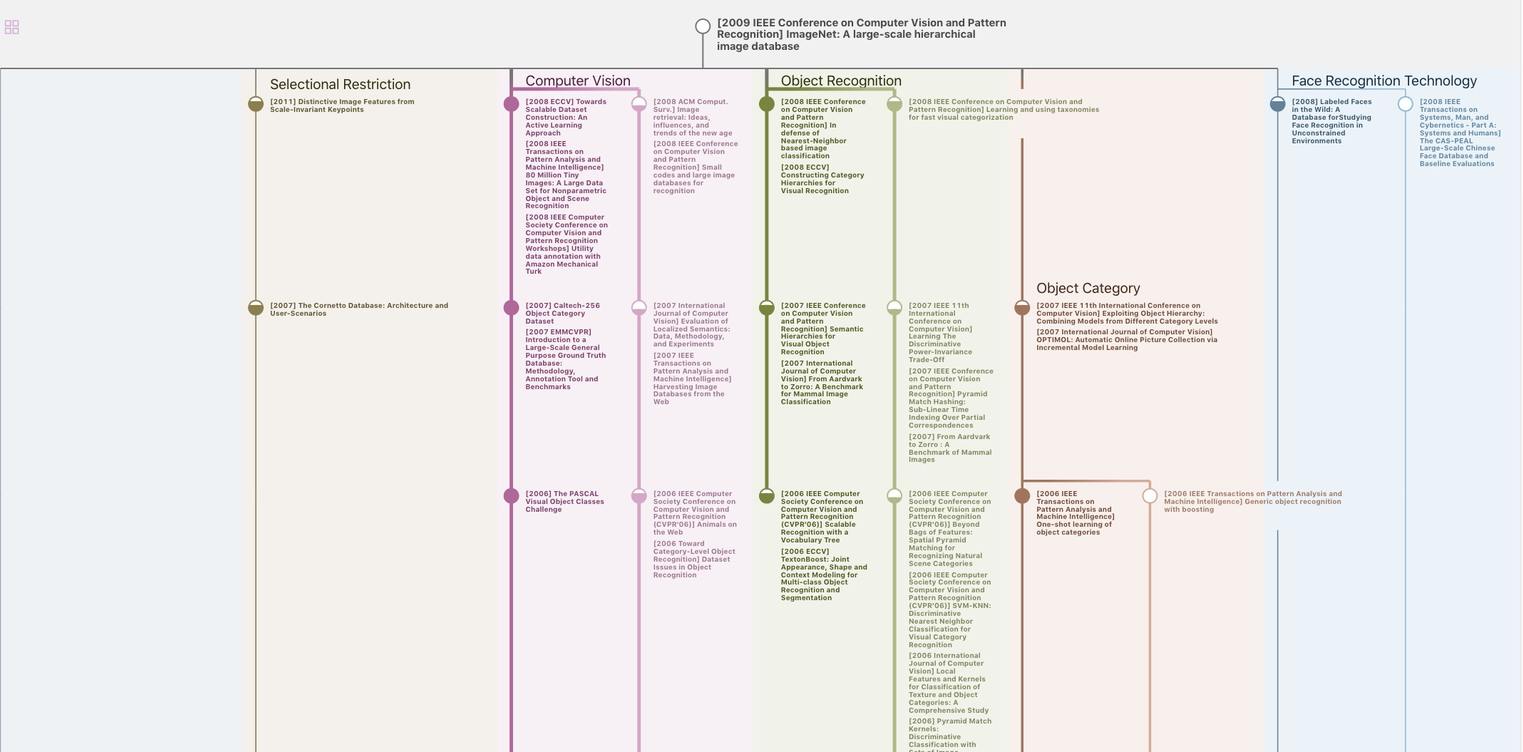
生成溯源树,研究论文发展脉络
Chat Paper
正在生成论文摘要