Metric Transforms and Low Rank Matrices via Representation Theory of the Real Hyperrectangle
user-6144298de55422cecdaf68a5(2020)
摘要
In this paper, we develop a new technique which we call representation theory of the real hyperrectangle, which describes how to compute the eigenvectors and eigenvalues of certain matrices arising from hyperrectangles. We show that these matrices arise naturally when analyzing a number of different algorithmic tasks such as kernel methods, neural network training, natural language processing, and the design of algorithms using the polynomial method. We then use our new technique along with these connections to prove several new structural results in these areas, including: $\bullet$ A function is a positive definite Manhattan kernel if and only if it is a completely monotone function. These kernels are widely used across machine learning; one example is the Laplace kernel which is widely used in machine learning for chemistry. $\bullet$ A function transforms Manhattan distances to Manhattan distances if and only if it is a Bernstein function. This completes the theory of Manhattan to Manhattan metric transforms initiated by Assouad in 1980. $\bullet$ A function applied entry-wise to any square matrix of rank $r$ always results in a matrix of rank $< 2^{r-1}$ if and only if it is a polynomial of sufficiently low degree. This gives a converse to a key lemma used by the polynomial method in algorithm design. Our work includes a sophisticated combination of techniques from different fields, including metric embeddings, the polynomial method, and group representation theory.
更多查看译文
关键词
real hyperrectangle,low rank matrices,representation theory,metric
AI 理解论文
溯源树
样例
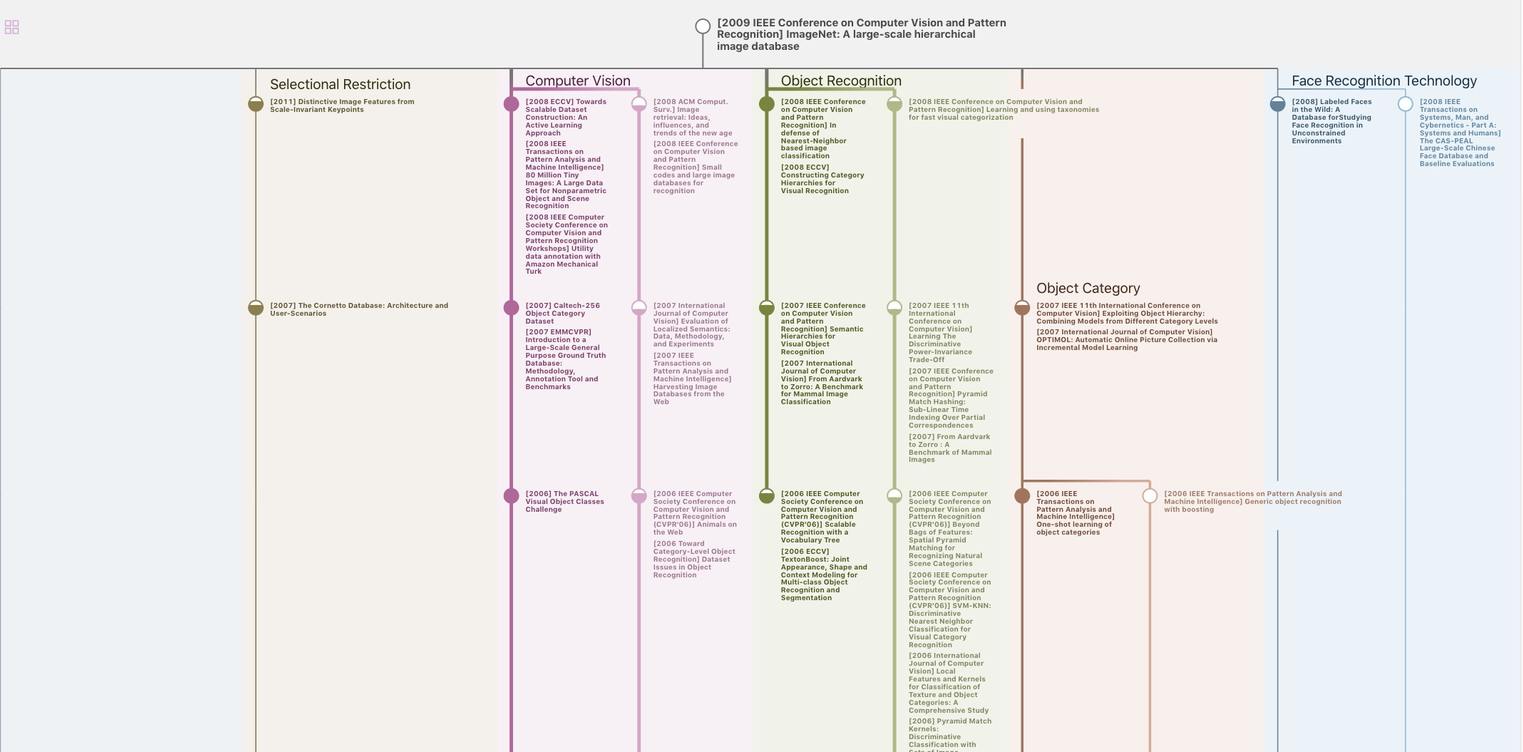
生成溯源树,研究论文发展脉络
Chat Paper
正在生成论文摘要