Non-Parametric Model-Based Estimation of the Effective Reproduction Number for SARS-CoV-2
ALGORITHMS(2023)
摘要
Describing viral outbreaks, such as the COVID-19 pandemic, often involves employing compartmental models composed of ordinary differential equation (ODE) systems. Estimating the parameter values for these ODE models is crucial and relies on accessible data. To accurately represent realistic pandemic scenarios with diverse situations, it is necessary to consider model parameters as time dependent. However, estimating such time-dependent parameters, like transition rates in compartmental models, is notoriously challenging due to the unknown function class of these parameters. In this study, we propose a novel approach by using an Augmented Kalman Smoother (AKS) combined with an Expectation-Maximization (EM) algorithm to simultaneously estimate all time-dependent parameters in an SIRD compartmental model. Our approach can be applied to general ODE systems with time-varying parameters, requiring no prior knowledge of model parameters or additional assumptions on the function class of the ODE time dependencies. A key advantage of our method compared to other methods is that it does not require assumptions about the parameterization of the serial interval distribution for estimating SIRD model parameters. Applying our approach to COVID-19 data in Germany, we adequately describe time-series data with strong fluctuations and multiple waves, obtaining non-parametric model-based time-course estimates for the effective reproduction number.
更多查看译文
关键词
SARS-CoV-2,augmented Kalman smoother,SIRD compartmental model,effective reproduction number,estimation of time-dependent parameters,ordinary differential equations
AI 理解论文
溯源树
样例
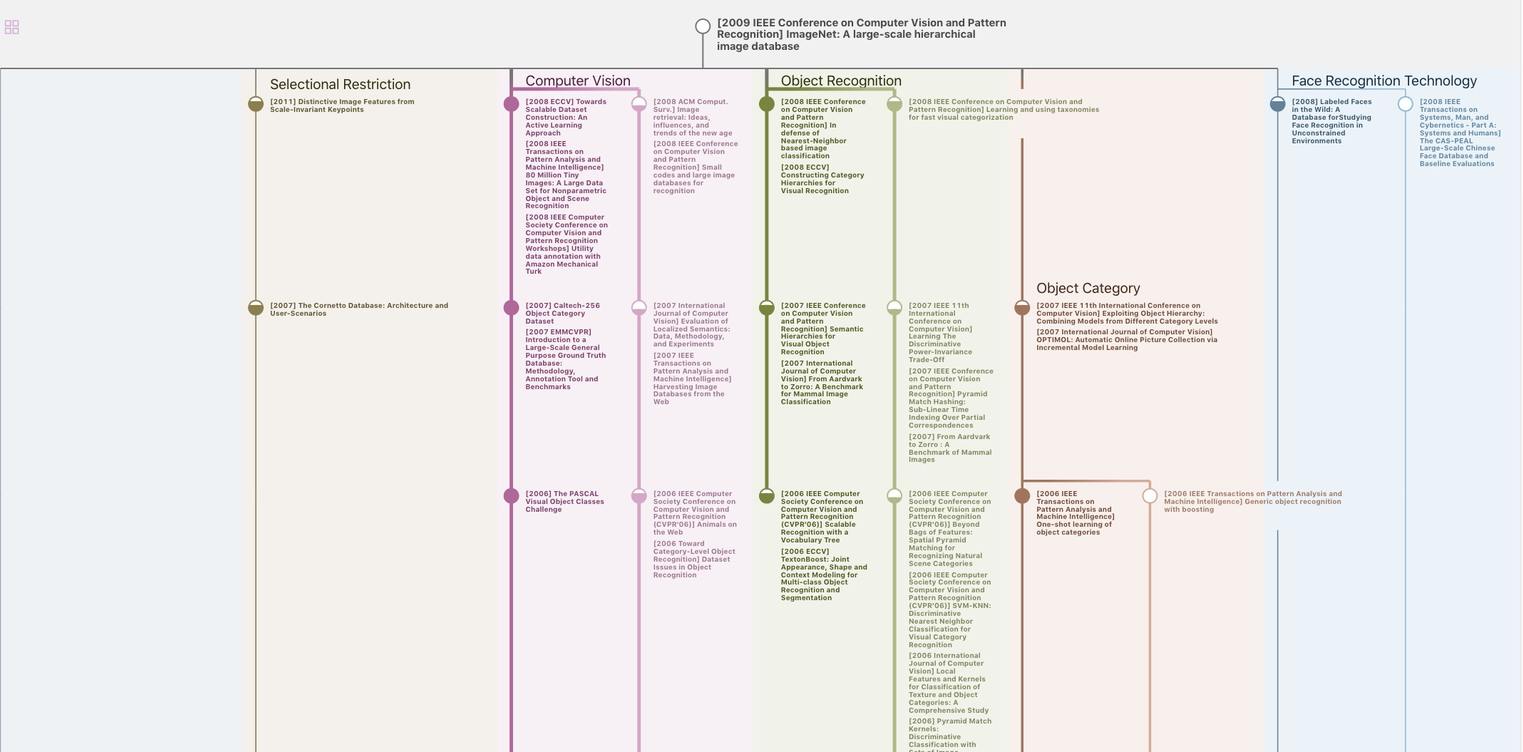
生成溯源树,研究论文发展脉络
Chat Paper
正在生成论文摘要