Aha! Adaptive History-driven Attack for Decision-based Black-box Models.
ICCV(2021)
摘要
The decision-based black-box attack means to craft adversarial examples with only the top-1 label of the victim model available. A common practice is to start from a large perturbation and then iteratively reduce it with a deterministic direction and a random one while keeping it adversarial. The limited information obtained from each query and inefficient direction sampling impede attack efficiency, making it hard to obtain a small enough perturbation within a limited number of queries. To tackle this problem, we propose a novel attack method termed Adaptive History-driven Attack (AHA) which gathers information from all historical queries as the prior for current sampling. Moreover, to balance between the deterministic direction and the random one, we dynamically adjust the coefficient according to the ratio of the actual magnitude reduction to the expected one. Such a strategy improves the success rate of queries during optimization, letting adversarial examples move swiftly along the decision boundary. Our method can also integrate with subspace optimization like dimension reduction to further improve efficiency. Extensive experiments on both ImageNet and CelebA datasets demonstrate that our method achieves at least 24.3% lower magnitude of perturbation on average with the same number of queries. Finally, we prove the practical potential of our method by evaluating it on popular defense methods and a real-world system provided by MEGVII Face++.
更多查看译文
关键词
Adversarial learning
AI 理解论文
溯源树
样例
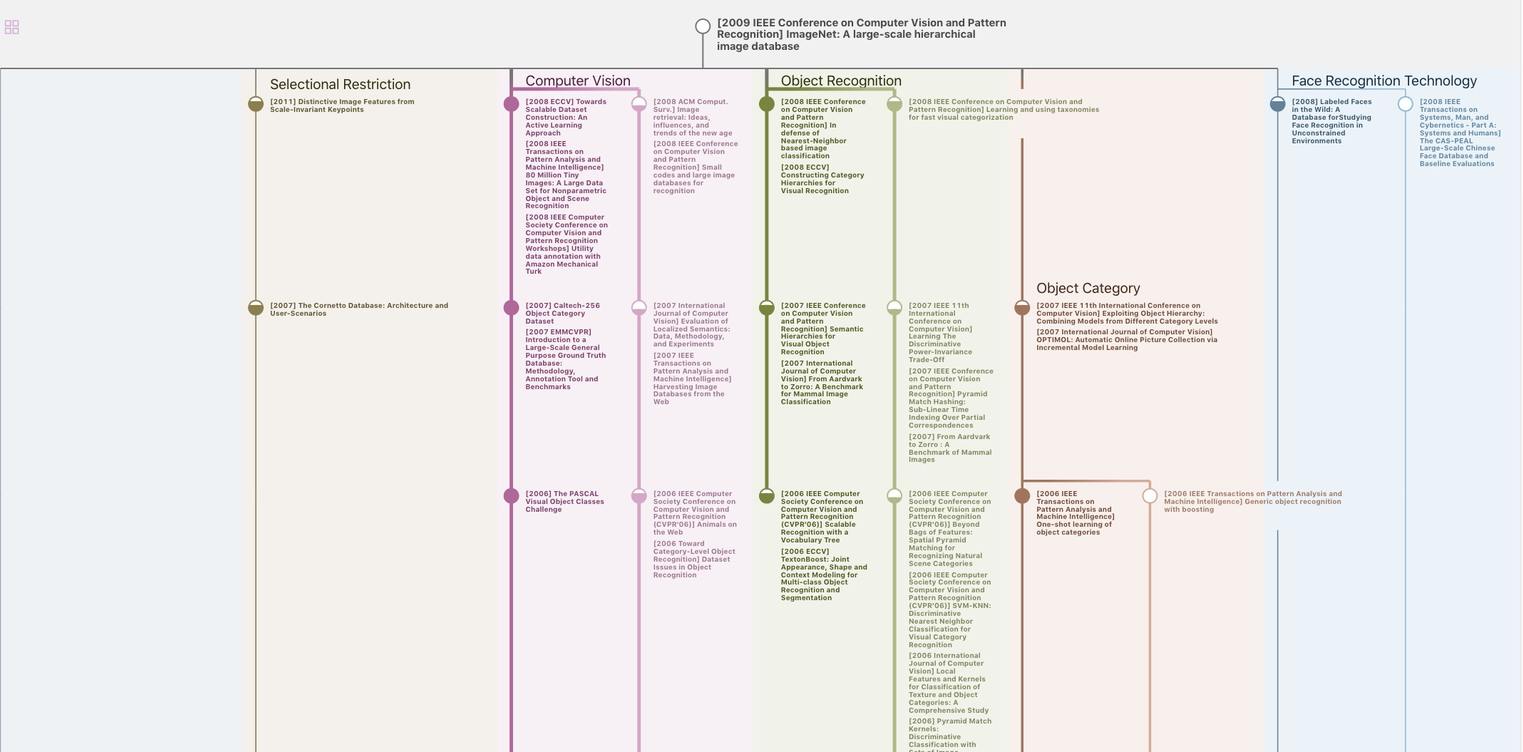
生成溯源树,研究论文发展脉络
Chat Paper
正在生成论文摘要