Label and Context Augmentation for Response Selection at DSTC8
IEEE-ACM TRANSACTIONS ON AUDIO SPEECH AND LANGUAGE PROCESSING(2021)
摘要
This paper studies the dialogue response selection task. As state-of-the-arts are neural models requiring a large training set, data augmentation has been considered as a means to overcome the sparsity of observational annotation, where only one observed response is annotated as gold. In this paper, we first consider label augmentation, of selecting, among unobserved utterances, that would "counterfactually" replace the labeled response, for the given context, and augmenting labels only if that is the case. The key advantage of this model is not incurring human annotation overhead, thus not increasing the training cost, i.e., for low-resource scenarios. In addition, we consider context augmentation scenarios where the given dialogue context is not sufficient for label augmentation. In this case, inspired by open-domain question answering, we "decontextualize" by retrieving missing contexts, such as related persona. We empirically show that our pipeline improves BERT-based models in two different response selection tasks without incurring annotation overheads.
更多查看译文
关键词
Task analysis,Training,Annotations,Bit error rate,Gold,Estimation,Context modeling,Conversation,response selection,data augmentation,counterfactual estimation
AI 理解论文
溯源树
样例
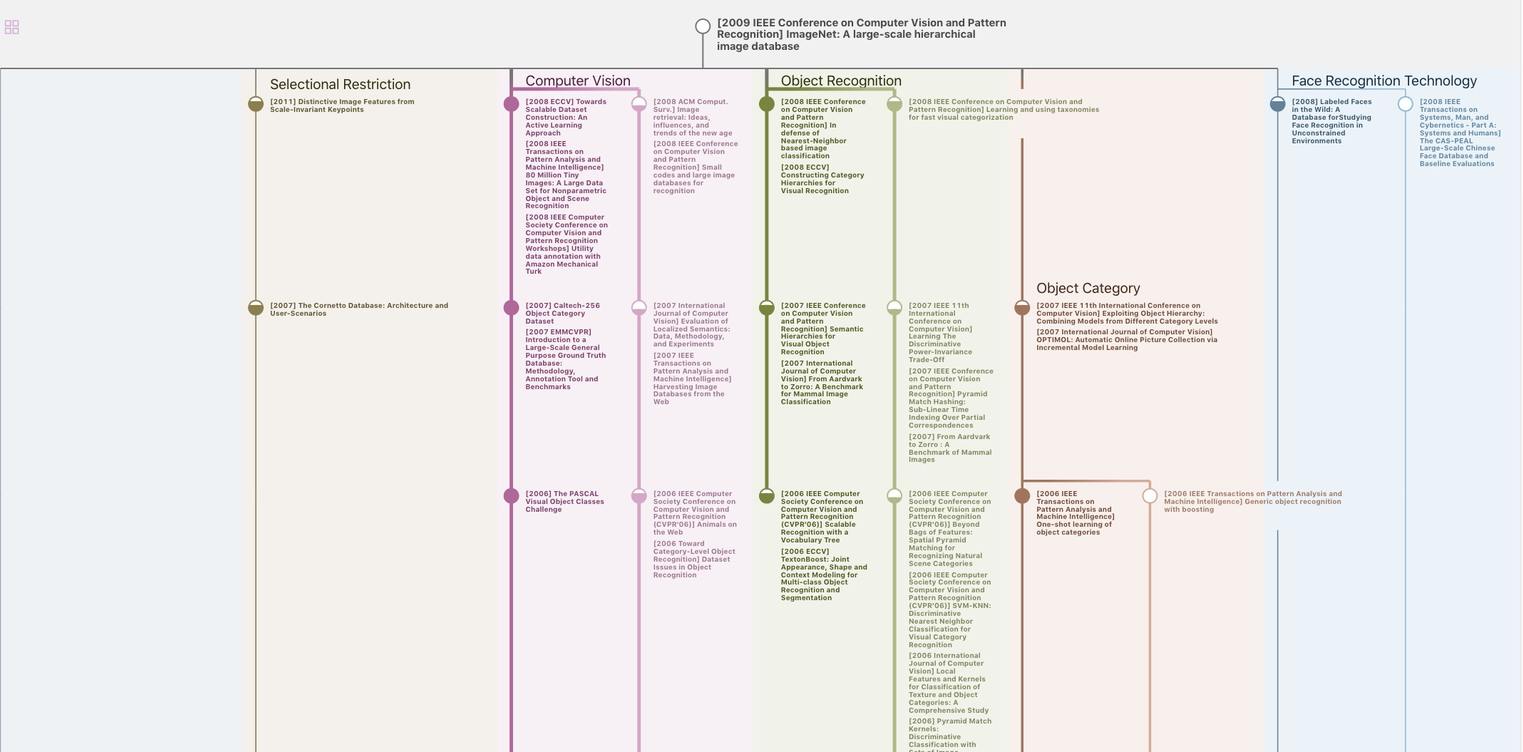
生成溯源树,研究论文发展脉络
Chat Paper
正在生成论文摘要