A Review of Scaling Genome Sequencing Data Anonymisation.
AINA (3)(2021)
摘要
Sequencing genomes and analysing their variations can make an essential contribution to healthcare research on drug discovery and advancing clinical care, for instance. Genome sequencing data, however, presents a special case of highly sparsely populated, multi-attribute, high-dimensional data, in which each record (tuple) can be associated with more than tens of thousands of attributes on average. Since anonymising genome sequencing data is a necessary pre-processing step for privacy-preserving genomic data analysis for personalised care, discovering all the quasi-identifier combinations required to preserve anonymity is essential; This requires verifying an exponential number of quasi-identifier candidates to identify and remove all unique data values, an NP-hard problem for larger datasets. Furthermore, recent work classifies this problem to be at the very least W [2]-complete and not a fixed-parameter tractable problem. Thus, achieving efficient and scalable anonymisation of genome sequence data is a challenging problem. In this paper, we summarise the uniqueness of ensuring privacy in the context of (whole) genome sequencing. Further, we show and compare the latest trends to discover quasi-identifiers (QID) in large-scale genome data and concepts to counter the exponential runtime growth during QID candidate processing in this field. Finally, we present an architecture incorporating previous enhancements to enable near real-time QID discovery in high-dimensional genome data based on vectorised GPU-acceleration. Achieving anonymisation processing in our experiments in just a few seconds, which corresponds to speedups by factor 100, can be essential in life-or-death situations like triage.
更多查看译文
关键词
genome,data
AI 理解论文
溯源树
样例
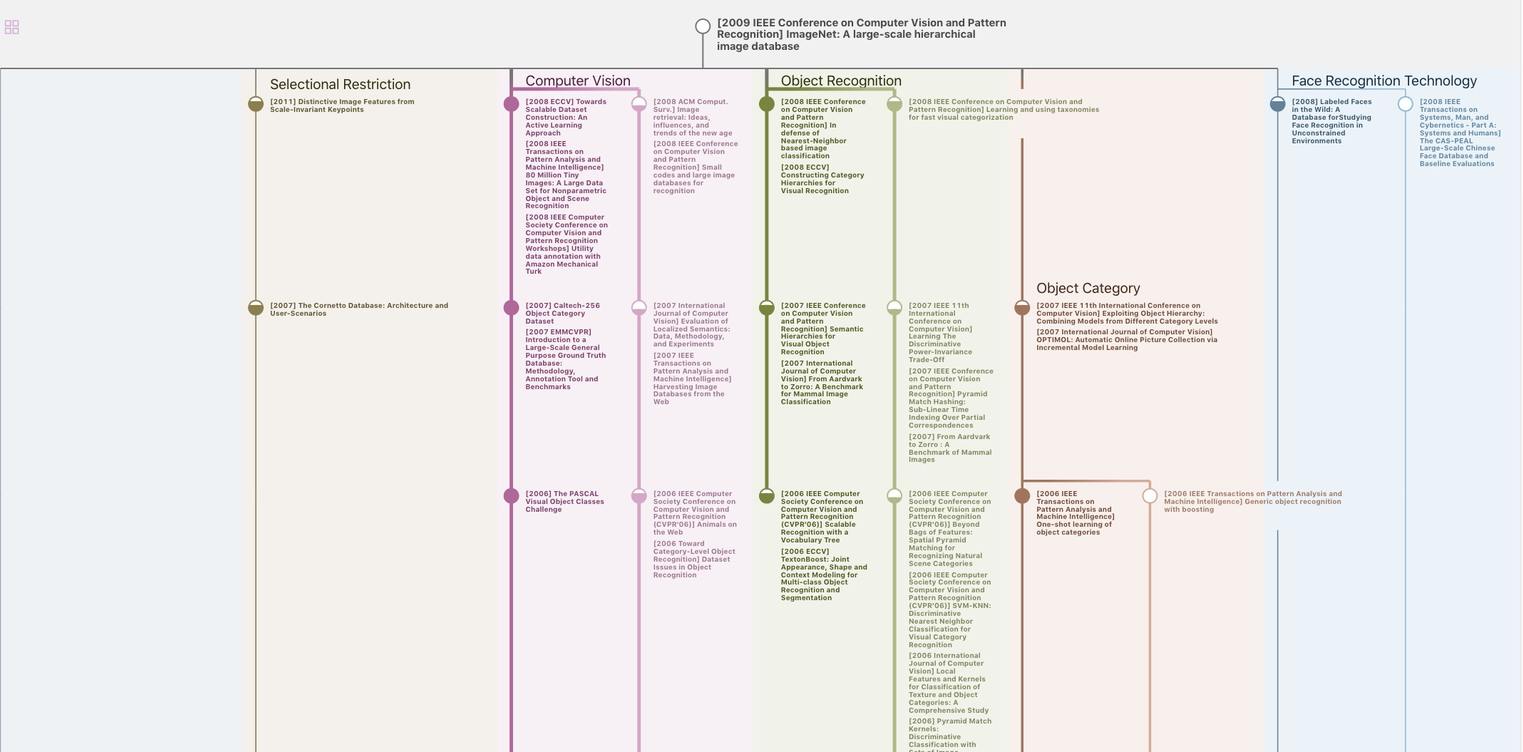
生成溯源树,研究论文发展脉络
Chat Paper
正在生成论文摘要