Adversarial Domain Adaptation for Medieval Instrument Recognition.
IntelliSys (2)(2021)
摘要
Image classification models have improved drastically due to neural networks. But as a direct consequence of being trained on a specific dataset, neural networks tend to be biased towards theirs training data and provide worse results on other domains. Hence, a new sub field of Transfer Learning emerged namely Domain Adaptation which uses in the most case a combination of adversarial methods and mathematical heuristics which are applied to the model’s latent space. In this paper we present a new method for Unsupervised Domain Adaptation that is both fast and resilient. Our method starts by applying style transformations to the input images and train a transformation discriminator module to predict these style changes. Whereas the feature extractor part of our model is trained on the adversarial part of that loss allowing to forget and not extract style information which in return improves the accuracy of the classifier. Our second contribution is our new dataset of Musical Instruments Recognition In Medieval Artworks which provides a better benchmark for transfer learning and domain adaptation methods and pushes the research in this area further. We evaluated our method on two main benchmarks namely MNIST-USPS-SVHN and MIMI-Musiconis-Vihuelas benchmark and in all cases our method provides state of the art performances.
更多查看译文
关键词
medieval instrument recognition,adversarial domain adaptation,domain adaptation
AI 理解论文
溯源树
样例
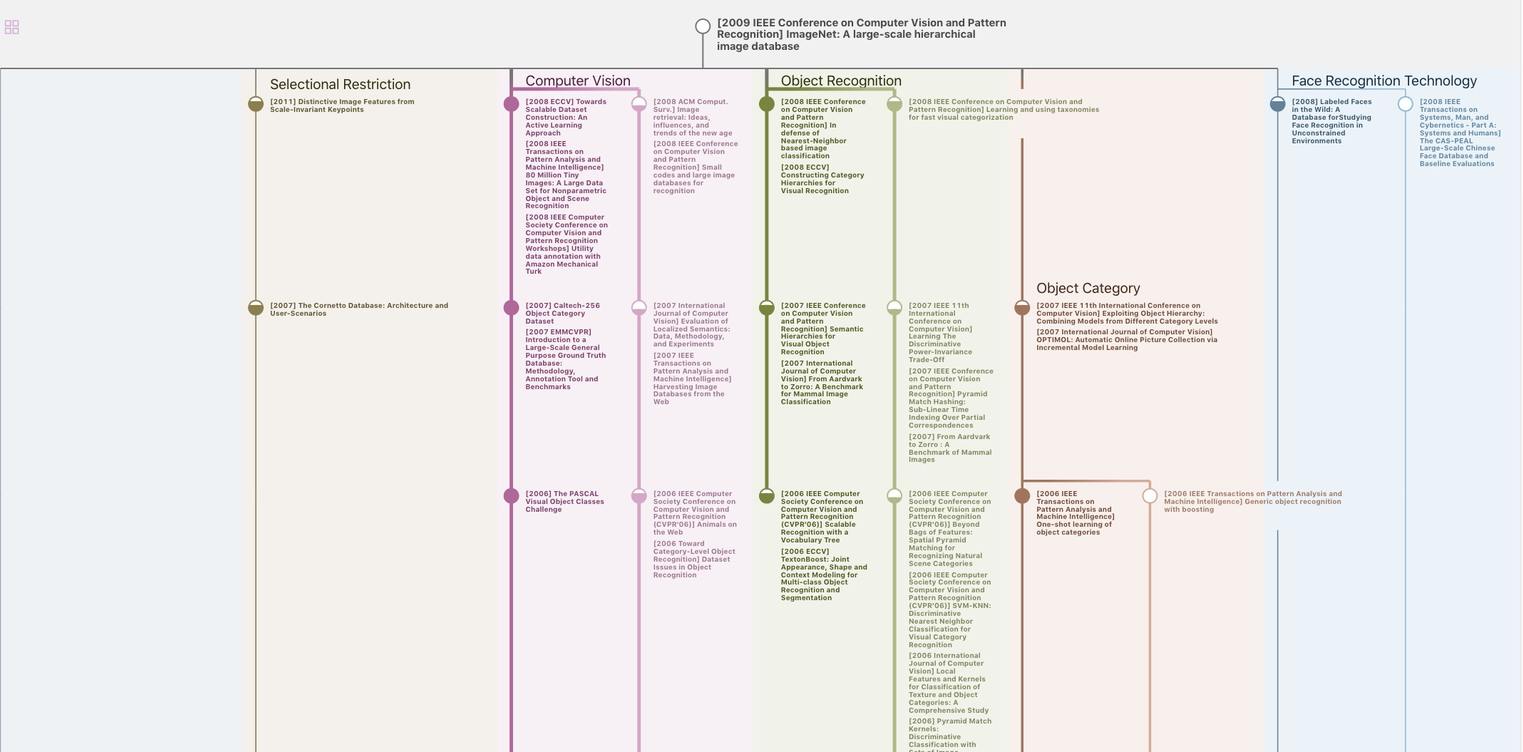
生成溯源树,研究论文发展脉络
Chat Paper
正在生成论文摘要