Assessing Algorithmic Fairness in Automatic Classifiers of Educational Forum Posts.
AIED (1)(2021)
摘要
Automatic classifiers of educational forum posts are essential in helping instructors effectively implement their teaching practices and thus have been widely investigated. However, existing studies mostly stressed the accuracy of a classifier, while the fairness of the classifier remains largely unexplored, i.e., whether the posts generated by a group of students are more likely to be correctly labeled than those generated by other groups of students. Undoubtedly, any unfairness based on student performance, sex, or other subjective views can have a detrimental effect on a student's learning experience and performance. Therefore, this study aimed to assess the algorithmic fairness of six popular models used in building automatic classifiers of educational forum posts. Here, we measured the algorithmic fairness displayed (i) between students of different sex (female vs. male) and (ii) between students of different first languages (English-as-first-language speakers vs. Englishas-second-language speakers). Besides, we investigated whether a classifier's fairness could be enhanced by applying data sampling techniques. Our results demonstrated that: 1) traditional Machine Learning models slightly outperformed up-to-date Deep Learning models in delivering fair predictions; 2) students of different first languages faced more unfair predictions than students of different sex, and most of the classifiers tended to favor English-as-first-language students; and 3) with equal numbers of posts generated by different groups of students in the training data, the fairness of a classifier could be greatly enhanced.
更多查看译文
关键词
Educational forum post,Text classification,Algorithmic fairness
AI 理解论文
溯源树
样例
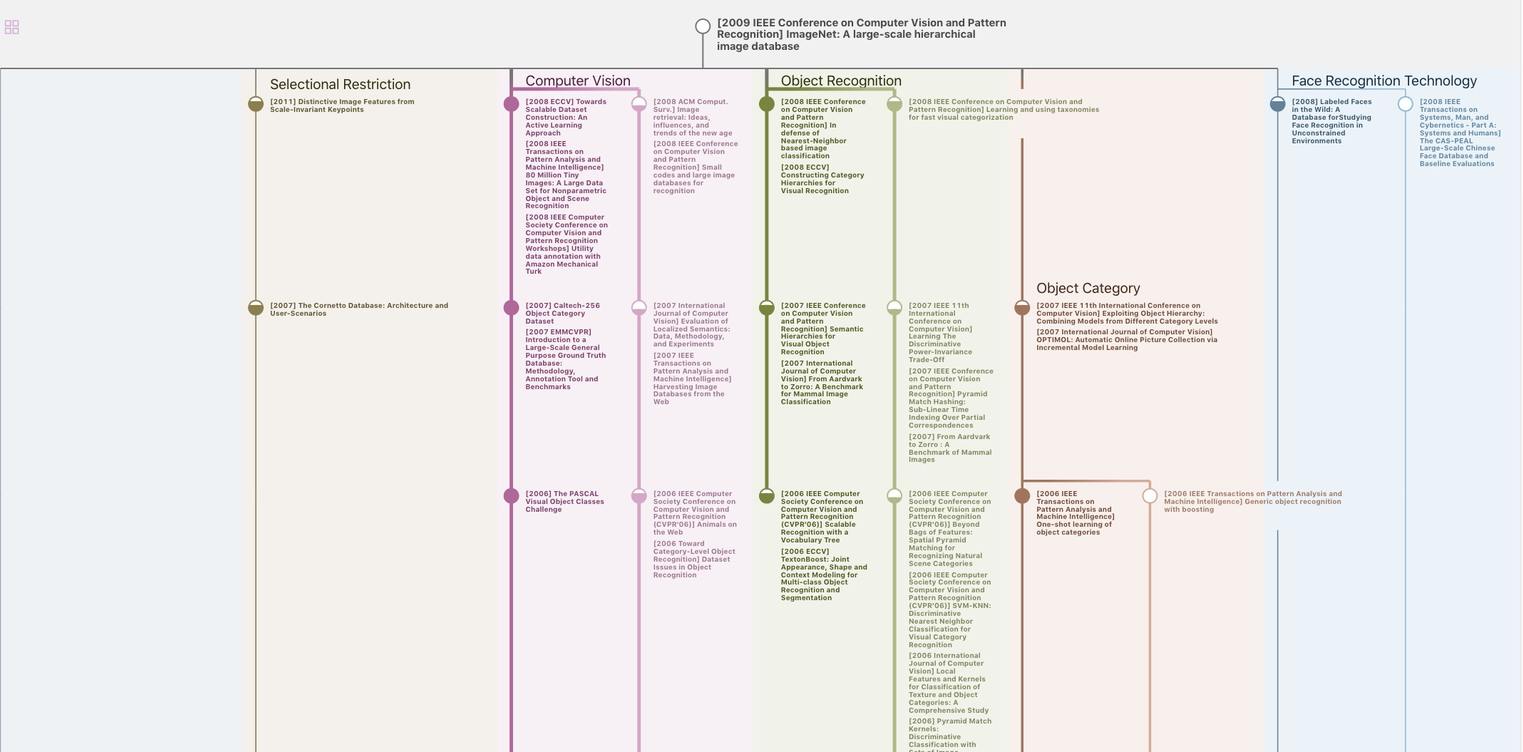
生成溯源树,研究论文发展脉络
Chat Paper
正在生成论文摘要