Selective Ensembles for Consistent Predictions
International Conference on Learning Representations (ICLR)(2022)
摘要
Recent work has shown that models trained to the same objective, and which achieve similar measures of accuracy on consistent test data, may nonetheless behave very differently on individual predictions. This inconsistency is undesirable in high-stakes contexts, such as medical diagnosis and finance. We show that this inconsistent behavior extends beyond predictions to feature attributions, which may likewise have negative implications for the intelligibility of a model, and one's ability to find recourse for subjects. We then introduce selective ensembles to mitigate such inconsistencies by applying hypothesis testing to the predictions of a set of models trained using randomly-selected starting conditions; importantly, selective ensembles can abstain in cases where a consistent outcome cannot be achieved up to a specified confidence level. We prove that that prediction disagreement between selective ensembles is bounded, and empirically demonstrate that selective ensembles achieve consistent predictions and feature attributions while maintaining low abstention rates. On several benchmark datasets, selective ensembles reach zero inconsistently predicted points, with abstention rates as low 1.5%.
更多查看译文
关键词
consistency,prediction consistency,model duplicity,inconsistent predictions,deep models,deep networks,explanations,saliency maps,gradient-based explanations,fairness,interpretability
AI 理解论文
溯源树
样例
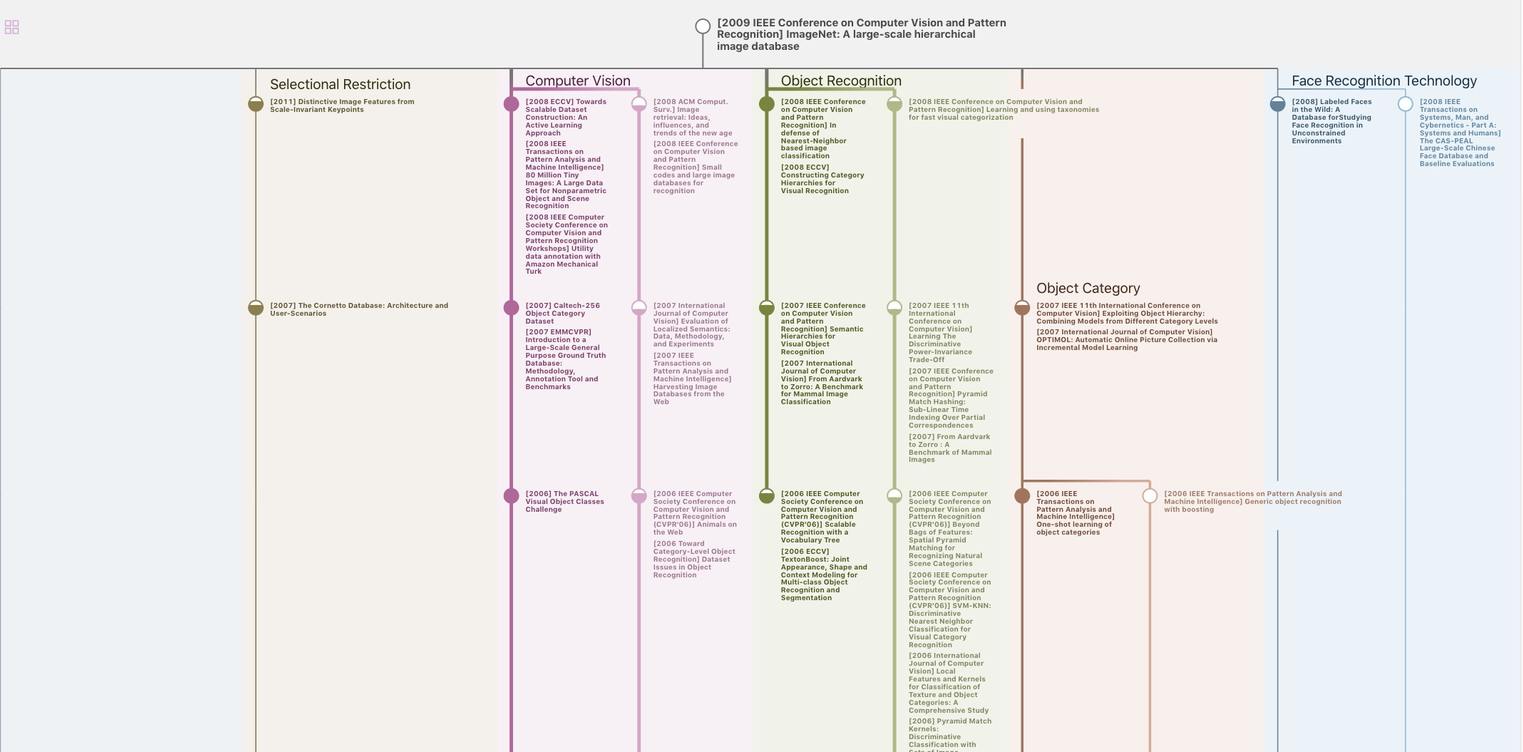
生成溯源树,研究论文发展脉络
Chat Paper
正在生成论文摘要