Developing an Image-Based Deep Learning Framework for Automatic Scoring of the Pentagon Drawing Test
JOURNAL OF ALZHEIMERS DISEASE(2022)
摘要
Background: The Pentagon Drawing Test (PDT) is a common assessment for visuospatial function. Evaluating the PDT by artificial intelligence can improve efficiency and reliability in the big data era. This study aimed to develop a deep learning (DL) framework for automatic scoring of the PDT based on image data. Methods: A total of 823 PDT photos were retrospectively collected and preprocessed into black-and-white, square-shape images. Stratified fivefold cross-validation was applied for training and testing. Two strategies based on convolutional neural networks were compared. The first strategy was to perform an image classification task using supervised transfer learning. The second strategy was designed with an object detection model for recognizing the geometric shapes in the figure, followed by a predetermined algorithm to score based on their classes and positions. Results: On average, the first framework demonstrated 62% accuracy, 62% recall, 65% precision, 63% specificity, and 0.72 area under the receiver operating characteristic curve. This performance was substantially outperformed by the second framework, with averages of 94%, 95%, 93%, 93%, and 0.95, respectively. Conclusion: An image-basedDLframework based on the object detection approach may be clinically applicable for automatic scoring of the PDT with high efficiency and reliability. With a limited sample size, transfer learning should be used with caution if the newimages are distinct from the previous training data. Partitioning the problem-solvingworkflowinto multiple simple tasks should facilitate model selection, improve performance, and allow comprehensible logic of the DL framework.
更多查看译文
关键词
Computer vision, convolutional neural network, deep learning, image classification, object detection, Pentagon Drawing Test
AI 理解论文
溯源树
样例
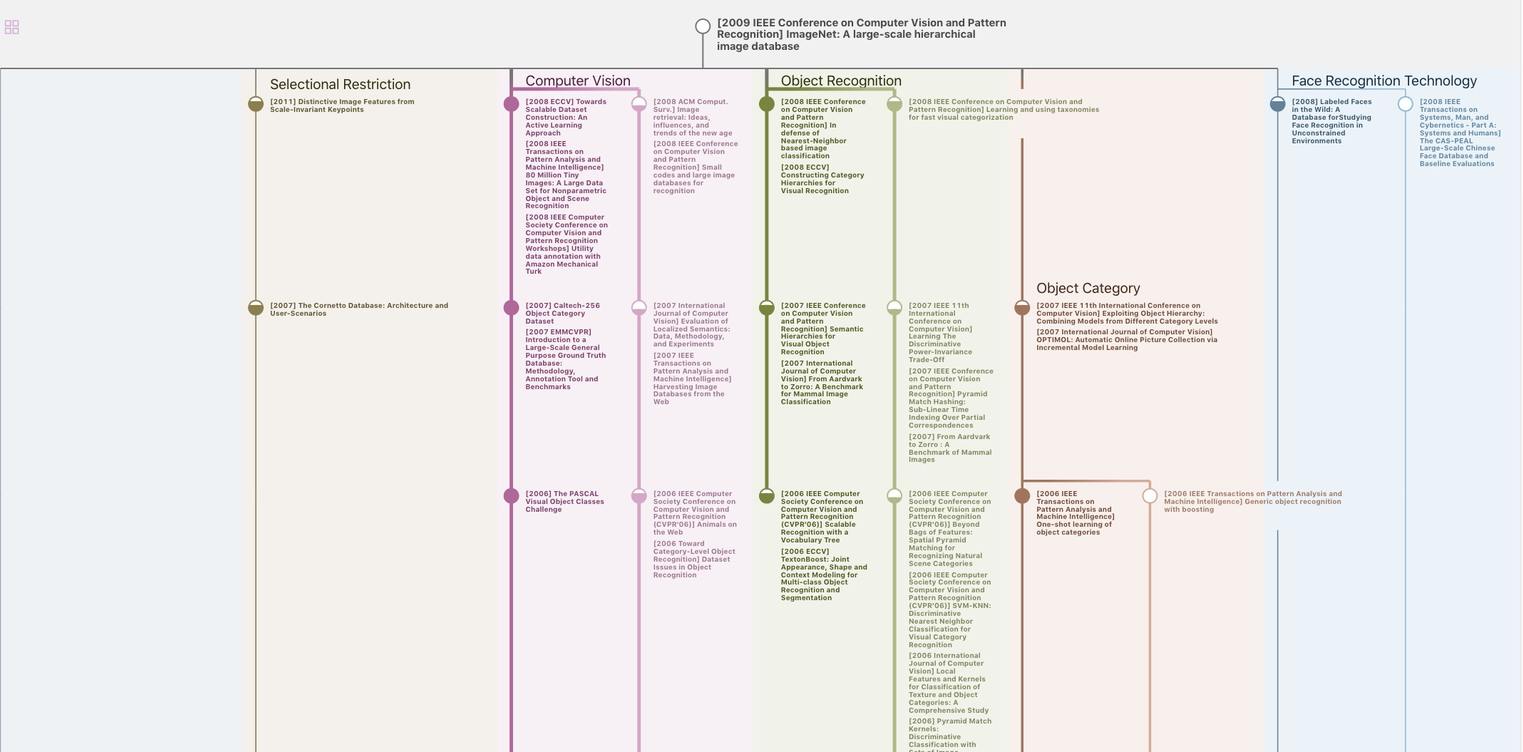
生成溯源树,研究论文发展脉络
Chat Paper
正在生成论文摘要