Analysis of Data Augmentation Methods for Low-Resource Maltese ASR
arxiv(2023)
摘要
Recent years have seen an increased interest in the computational speech processing of Maltese, but resources remain sparse. In this paper, we consider data augmentation techniques for improving speech recognition for low-resource languages, focusing on Maltese as a test case. We consider three different types of data augmentation: unsupervised training, multilingual training and the use of synthesized speech as training data. The goal is to determine which of these techniques, or combination of them, is the most effective to improve speech recognition for languages where the starting point is a small corpus of approximately 7 hours of transcribed speech. Our results show that combining the data augmentation techniques studied here lead us to an absolute WER improvement of 15% without the use of a language model.
更多查看译文
关键词
data augmentation methods,low-resource
AI 理解论文
溯源树
样例
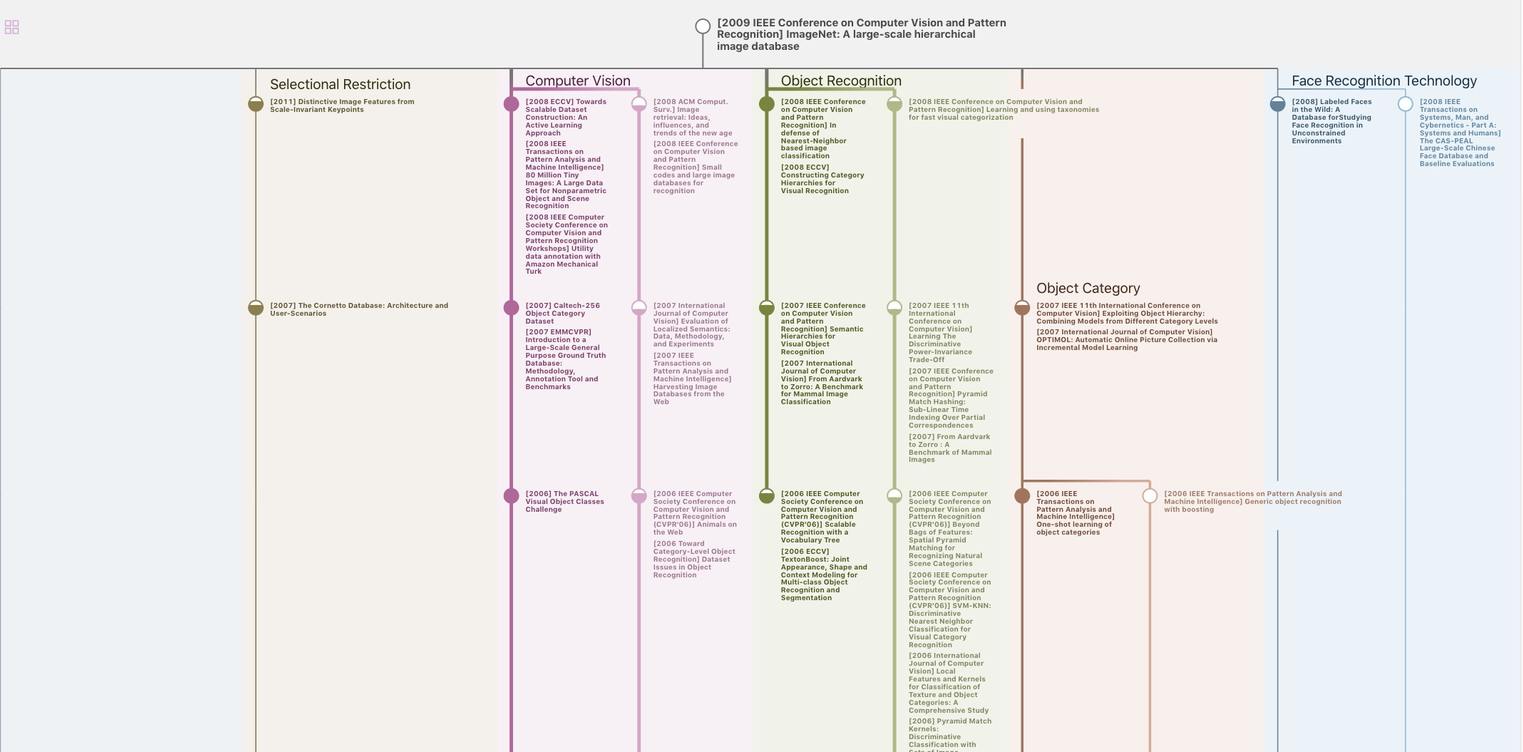
生成溯源树,研究论文发展脉络
Chat Paper
正在生成论文摘要