A Thorough Evaluation of Task-Specific Pretraining for Summarization.
EMNLP(2021)
摘要
Task-agnostic pretraining objectives like masked language models or corrupted span prediction are applicable to a wide range of NLP downstream tasks (Raffel et al., 2019), but are outperformed by task-specific pretraining objectives like predicting extracted gap sentences on summarization (Zhang et al., 2020). We compare three summarization specific pretraining objectives with the task agnostic corrupted span prediction pretraining in a controlled study. We also extend our study to a low resource and zero shot setup, to understand how many training examples are needed in order to ablate the task-specific pretraining without quality loss. Our results show that task-agnostic pretraining is sufficient for most cases which hopefully reduces the need for costly task-specific pretraining. We also report new state-of-the-art number for two summarization tasks using a T5 model with 11 billion parameters and an optimal beam search length penalty.
更多查看译文
关键词
thorough evaluation,task-specific
AI 理解论文
溯源树
样例
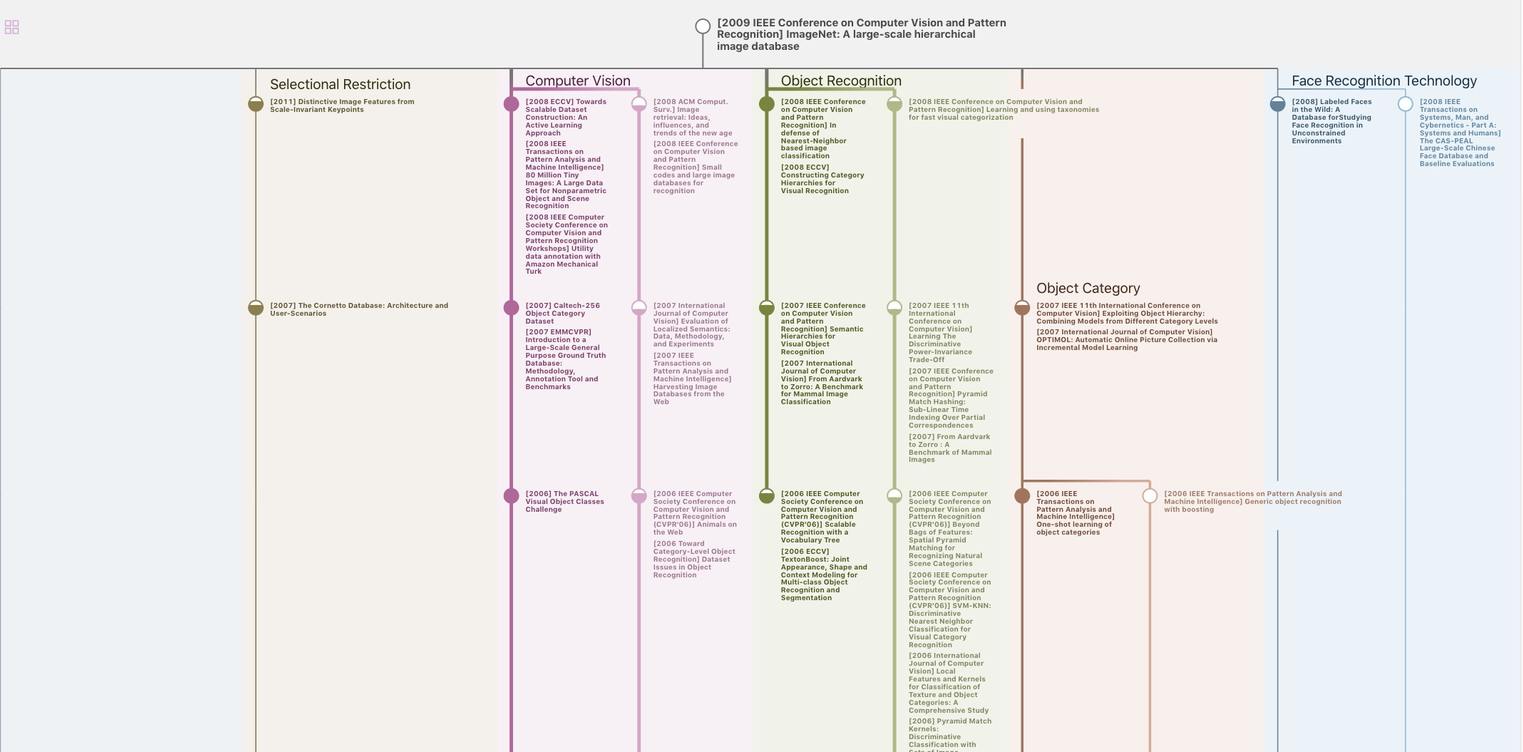
生成溯源树,研究论文发展脉络
Chat Paper
正在生成论文摘要