Students Who Study Together Learn Better - On the Importance of Collective Knowledge Distillation for Domain Transfer in Fact Verification.
EMNLP(2021)
摘要
While neural networks produce state-of-the-art performance in several NLP tasks, they depend heavily on lexicalized information, which transfers poorly between domains. Previous work (Suntwal et al., 2019) proposed delexicalization as a form of knowledge distillation to reduce dependency on such lexical artifacts. However, a critical unsolved issue that remains is how much delexicalization should be applied? A little helps reduce over-fitting, but too much discards useful information. We propose Group Learning (GL), a knowledge and model distillation approach for fact verification. In our method, while multiple student models have access to different delexicalized data views, they are encouraged to independently learn from each other through pair-wise consistency losses. In several cross-domain experiments between the FEVER and FNC fact verification datasets, we show that our approach learns the best delexicalization strategy for the given training dataset and outperforms state-of-theart classifiers that rely on the original data.
更多查看译文
AI 理解论文
溯源树
样例
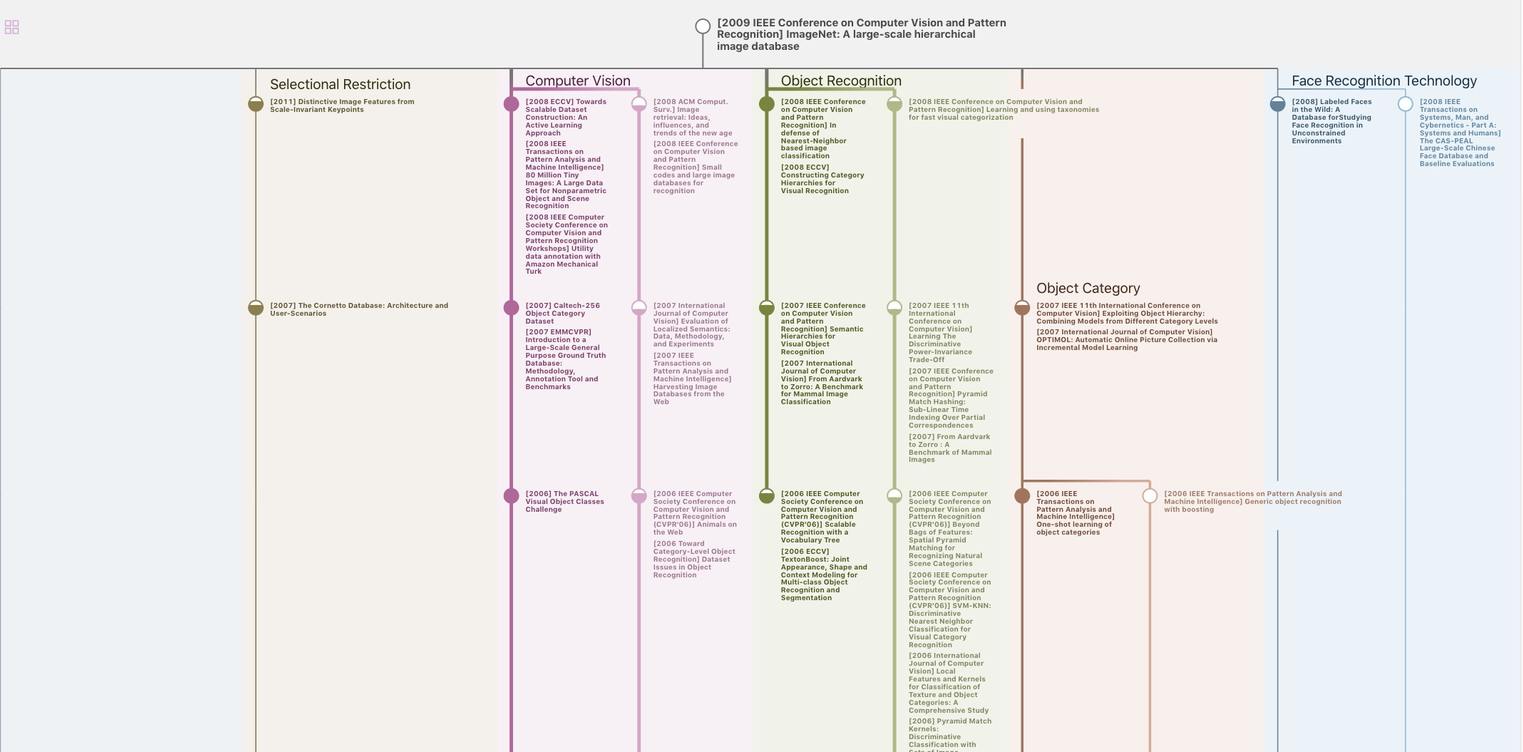
生成溯源树,研究论文发展脉络
Chat Paper
正在生成论文摘要