A comprehensive and fair comparison of two neural operators (with practical extensions) based on FAIR data
Computer Methods in Applied Mechanics and Engineering(2022)
摘要
Neural operators can learn nonlinear mappings between function spaces and offer a new simulation paradigm for real-time prediction of complex dynamics for realistic diverse applications as well as for system identification in science and engineering. Herein, we investigate the performance of two neural operators, which have shown promising results so far, and we develop new practical extensions that will make them more accurate and robust and importantly more suitable for industrial-complexity applications. The first neural operator, DeepONet, was published in 2019 (Lu et al., 2019), and its original architecture was based on the universal approximation theorem of Chen & Chen (1995). The second one, named Fourier Neural Operator or FNO, was published in 2020 (Li et al., 2020), and it is based on parameterizing the integral kernel in the Fourier space. DeepONet is represented by a summation of products of neural networks (NNs), corresponding to the branch NN for the input function and the trunk NN for the output function; both NNs are general architectures, e.g., the branch NN can be replaced with a CNN or a ResNet. According to Kovachki et al. (2021), FNO in its continuous form can be viewed conceptually as a DeepONet with a specific architecture of the branch NN and a trunk NN represented by a trigonometric basis. In order to compare FNO with DeepONet computationally for realistic setups, we develop several extensions of FNO that can deal with complex geometric domains as well as mappings where the input and output function spaces are of different dimensions. We also develop an extended DeepONet with special features that provide inductive bias and accelerate training, and we present a faster implementation of DeepONet with cost comparable to the computational cost of FNO, which is based on the Fast Fourier Transform.
更多查看译文
关键词
Nonlinear mappings,Operator regression,Deep learning,DeepONet,FNO,Scientific machine learning
AI 理解论文
溯源树
样例
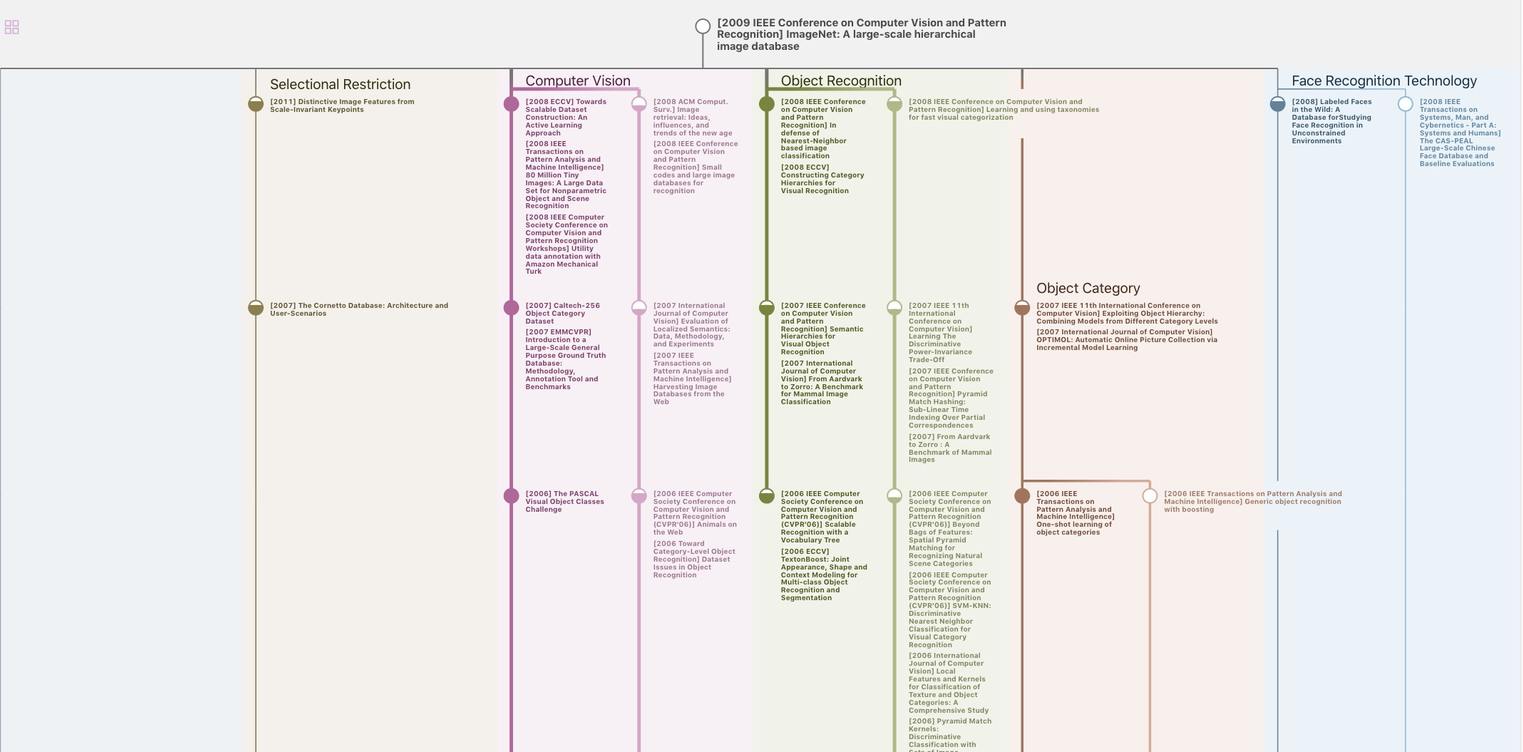
生成溯源树,研究论文发展脉络
Chat Paper
正在生成论文摘要