Reinforcement Learning for Mixed Autonomy Intersections.
ITSC(2021)
摘要
We propose a model-free reinforcement learning method for controlling mixed autonomy traffic in simulated traffic networks with through-traffic-only two-way and four-way intersections. Our method utilizes multi-agent policy decomposition which allows decentralized control based on local observations for an arbitrary number of controlled vehicles. We demonstrate that, even without reward shaping, reinforcement learning learns to coordinate the vehicles to exhibit traffic signal-like behaviors, achieving near-optimal throughput with 33-50% controlled vehicles. With the help of multi-task learning and transfer learning, we show that this behavior generalizes across inflow rates and size of the traffic network. Our code, models, and videos of results are available at https://github.com/ZhongxiaYan/mixed_autonomy_intersections.
更多查看译文
关键词
mixed autonomy,reinforcement learning,multi-agent systems
AI 理解论文
溯源树
样例
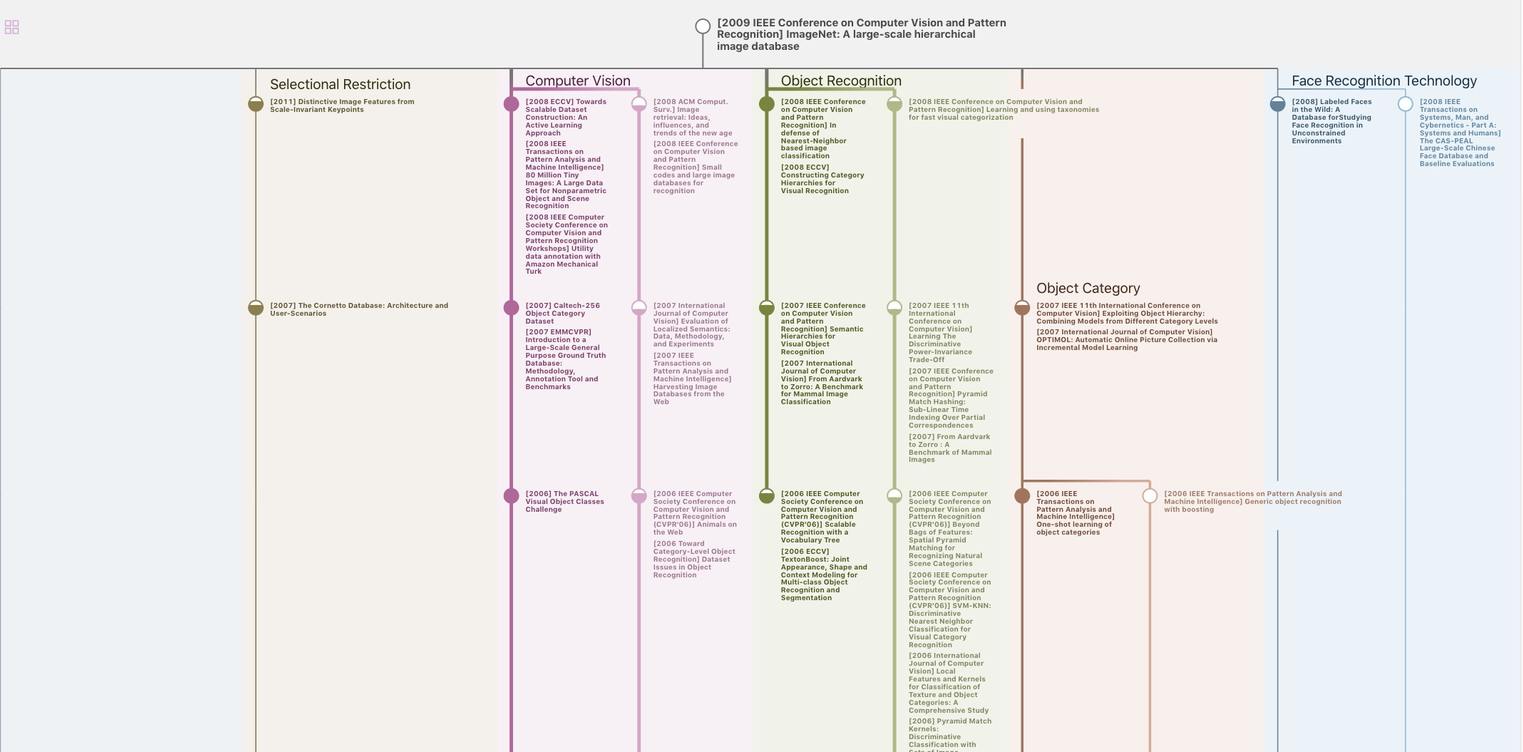
生成溯源树,研究论文发展脉络
Chat Paper
正在生成论文摘要