Learning Congestion Propagation Behaviors for Traffic Prediction.
ITSC(2021)
摘要
Traffic prediction is a challenging task as the traffic flow is influenced by many seasonal, stochastic, and structural factors. In addition, the spatial and temporal distribution of traffic flow can induce direct and indirect congestion propagation patterns. While existing works have attempted to model spatial-temporal graphs to capture the spatial correlations and temporal dependencies, they fail to consider congestion propagation behavior among road segments. In this paper, we propose a novel traffic prediction model that takes into account the congestion propagation tendencies to improve prediction accuracy. A novel diffusion graph convolution network model is developed to capture the spatial traffic correlations while considering the congestion propagation behavior. Our model also jointly learns the importance of seasonal traffic speed correlations, road contextual information (structural information), and stochastic factors (external factors) through an attention layer. Experimental results on real-world data-set demonstrate the superiority of our method over state-of-the-art traffic prediction techniques, and confirm the significance of congestion propagation behavior in traffic prediction.
更多查看译文
关键词
stochastic factors,structural factors,direct congestion propagation patterns,indirect congestion propagation patterns,spatial-temporal graphs,congestion propagation tendencies,spatial traffic correlations,seasonal traffic speed correlations,traffic prediction techniques,congestion propagation behavior learning,traffic flow temporal distribution,traffic flow spatial distribution,diffusion graph convolution network model,road contextual information,attention layer
AI 理解论文
溯源树
样例
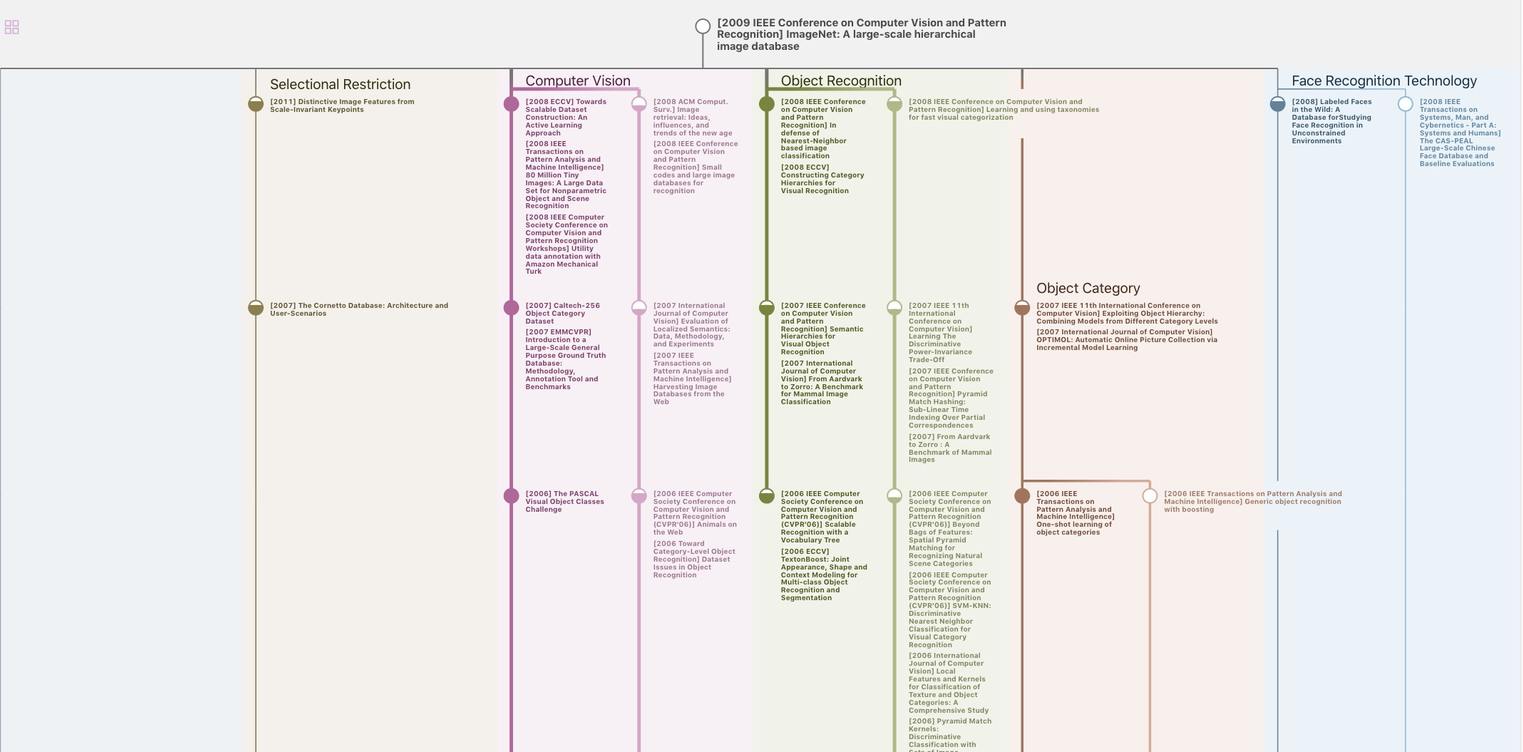
生成溯源树,研究论文发展脉络
Chat Paper
正在生成论文摘要