Answering POI-recommendation Questions using Tourism Reviews
Conference on Information and Knowledge Management(2021)
摘要
ABSTRACTWe introduce the novel and challenging task of answering Points-of-interest (POI) recommendation questions, using a collection of reviews that describe candidate answer entities (POIs). We harvest a QA dataset that contains 47,124 paragraph-sized user questions from travelers seeking POI recommendations for hotels, attractions and restaurants. Each question can have thousands of candidate entities to choose from and each candidate is associated with a collection of unstructured reviews. Questions can include requirements based on physical location, budget, timings as well as other subjective considerations related to ambience, quality of service etc. Our dataset requires reasoning over a large number of candidate answer entities (over 5300 per question on average) and we find that running commonly used neural architectures for QA is prohibitively expensive. Further, commonly used retriever-ranker based methods also do not work well for our task due to the nature of review-documents. Thus, as a first attempt at addressing some of the novel challenges of reasoning-at-scale posed by our task, we present a task specific baseline model that uses a three-stage cluster-select-rerank architecture. The model first clusters text for each entity to identify exemplar sentences describing an entity. It then uses a neural information retrieval (IR) module to select a set of potential entities from the large candidate set. A reranker uses a deeper attention-based architecture to pick the best answers from the selected entities. This strategy performs better than a pure retrieval or a pure attention-based reasoning approach yielding nearly 25% relative improvement in [email protected] over both approaches. To the best of our knowledge we are the first to present an unstructured QA-style task for POI-recommendation, using real-world tourism questions and POI-reviews.
更多查看译文
AI 理解论文
溯源树
样例
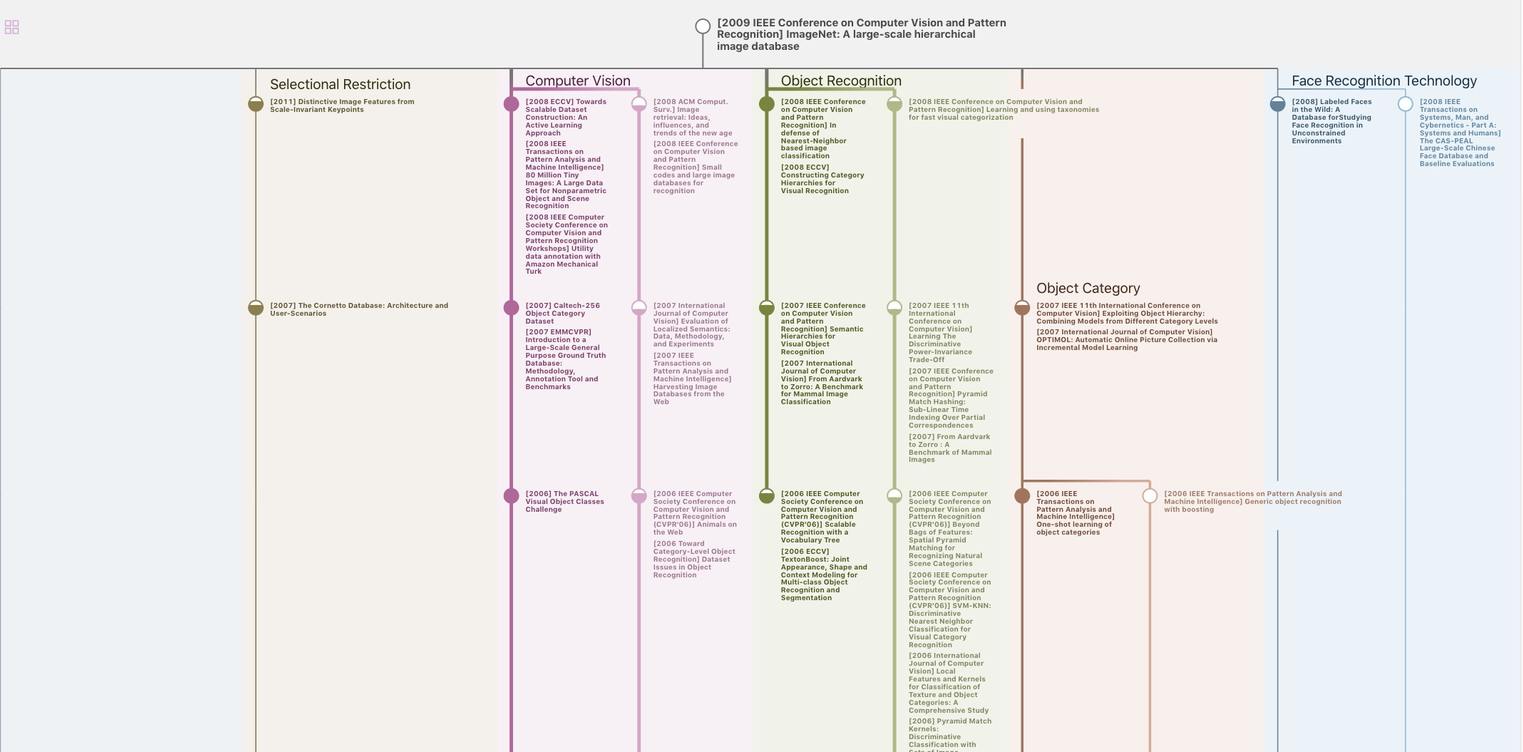
生成溯源树,研究论文发展脉络
Chat Paper
正在生成论文摘要