TimeMatch: Unsupervised cross-region adaptation by temporal shift estimation
ISPRS Journal of Photogrammetry and Remote Sensing(2022)
摘要
The recent developments of deep learning models that capture complex temporal patterns of crop phenology have greatly advanced crop classification from Satellite Image Time Series (SITS). However, when applied to target regions spatially different from the training region, these models perform poorly without any target labels due to the temporal shift of crop phenology between regions. Although various unsupervised domain adaptation techniques have been proposed in recent years, no method explicitly learns the temporal shift of SITS and thus provides only limited benefits for crop classification. To address this, we propose TimeMatch, which explicitly accounts for the temporal shift for improved SITS-based domain adaptation. In TimeMatch, we first estimate the temporal shift from the target to the source region using the predictions of a source-trained model. Then, we re-train the model for the target region by an iterative algorithm where the estimated shift is used to generate accurate target pseudo-labels. Additionally, we introduce an open-access dataset for cross-region adaptation from SITS in four different regions in Europe. On our dataset, we demonstrate that TimeMatch outperforms all competing methods by 11% in average F1-score across five different adaptation scenarios, setting a new state-of-the-art in cross-region adaptation. Our source code and dataset are available at https://github.com/jnyborg/timematch.
更多查看译文
关键词
Satellite image time series,Temporal shift,Crop classification,Domain adaptation,Deep learning
AI 理解论文
溯源树
样例
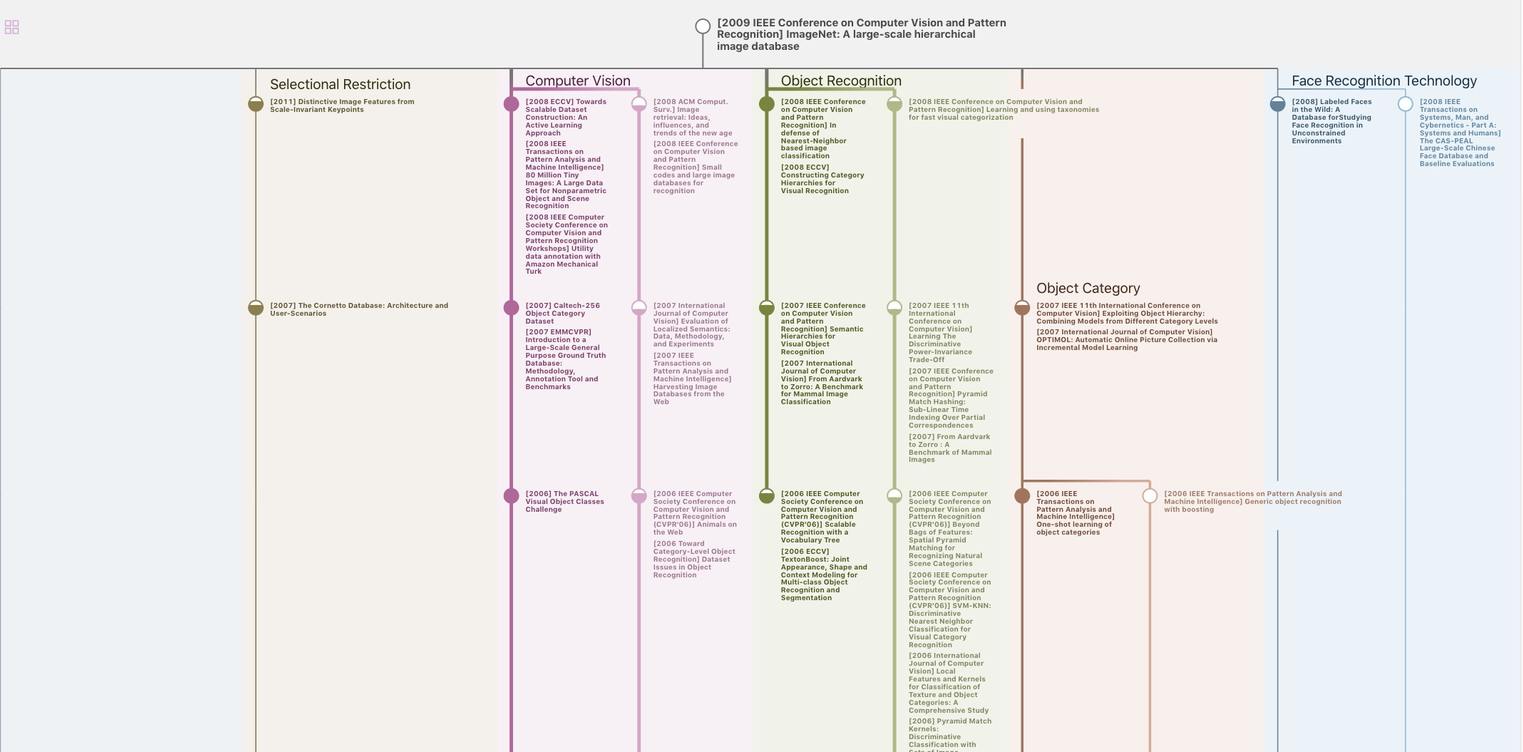
生成溯源树,研究论文发展脉络
Chat Paper
正在生成论文摘要