On Johnson's "Sufficientness" Postulates for Feature-Sampling Models
MATHEMATICS(2021)
摘要
In the 1920s, the English philosopher W.E. Johnson introduced a characterization of the symmetric Dirichlet prior distribution in terms of its predictive distribution. This is typically referred to as Johnson's "sufficientness " postulate, and it has been the subject of many contributions in Bayesian statistics, leading to predictive characterization for infinite-dimensional generalizations of the Dirichlet distribution, i.e., species-sampling models. In this paper, we review "sufficientness " postulates for species-sampling models, and then investigate analogous predictive characterizations for the more general feature-sampling models. In particular, we present a "sufficientness " postulate for a class of feature-sampling models referred to as Scaled Processes (SPs), and then discuss analogous characterizations in the general setup of feature-sampling models.
更多查看译文
关键词
Bayesian nonparametrics, exchangeability, feature-sampling model, de Finetti theorem, Johnson's "sufficientness" postulate, predictive distribution, scaled process prior, species-sampling model
AI 理解论文
溯源树
样例
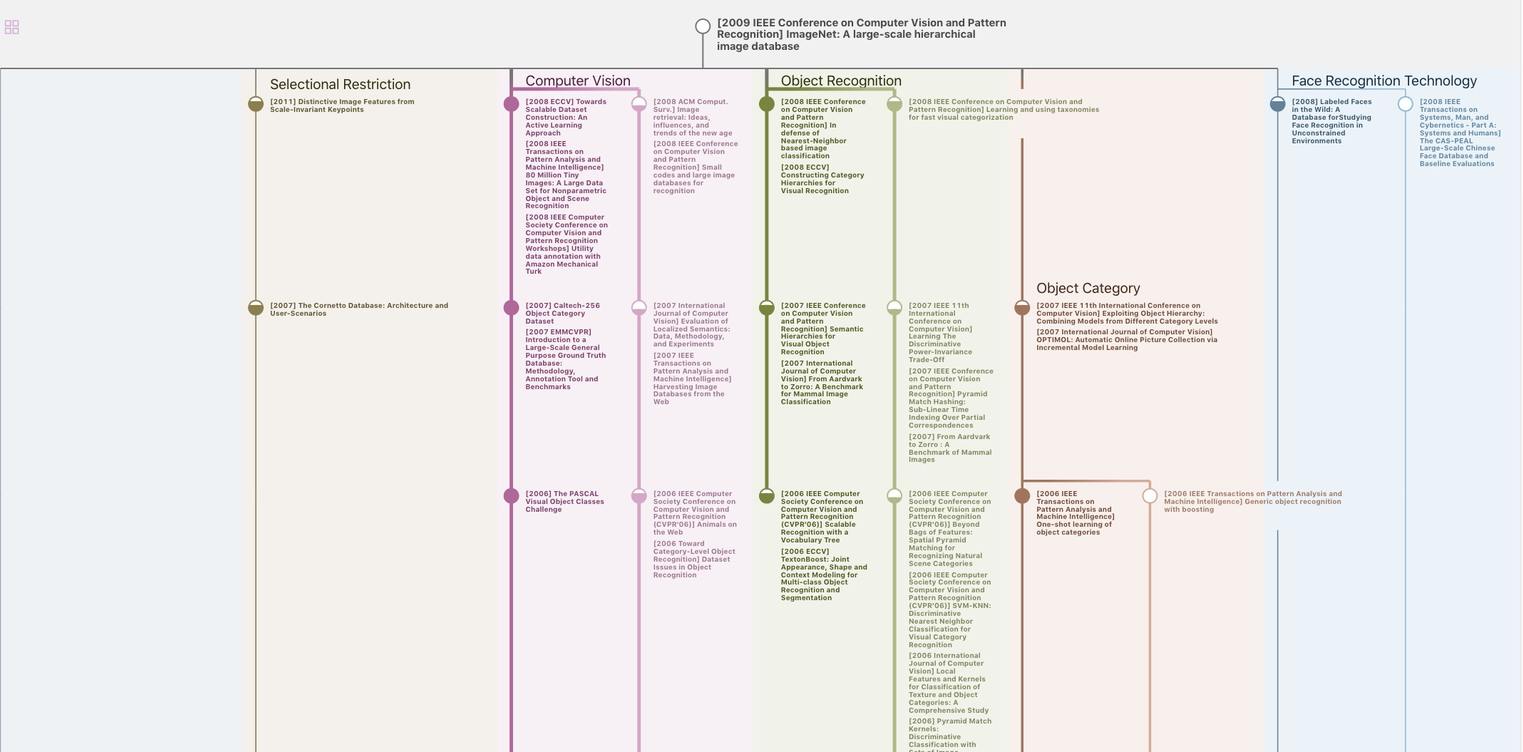
生成溯源树,研究论文发展脉络
Chat Paper
正在生成论文摘要