Invariant Object Representation Based on Principle of Maximal Dependence Capturing
biorxiv(2021)
摘要
Sensory inputs conveying information about the environment are often noisy and incomplete, yet the brain can achieve remarkable consistency in object recognition. This cognitive robustness is thought to be enabled by transforming the varying input patterns into invariant representations of objects, but how this transformation occurs computationally remains unclear. Here we propose that sensory coding should follow a principle of maximal dependence capturing to encode associations among structural components that can uniquely identify objects. We show that a computational framework incorporating dimension expansion and a specific form of sparse coding can capture structures that contain maximum information about specific objects, allow redundancy coding, and enable consistent representation of object identities. Using symbol and face recognition, we demonstrate that a two-layer system can generate representations that remain invariant under conditions of occlusion, corruption, or high noise.
### Competing Interest Statement
The authors declare the existence of a financial competing interest in the form of a patent application based on this work.
更多查看译文
AI 理解论文
溯源树
样例
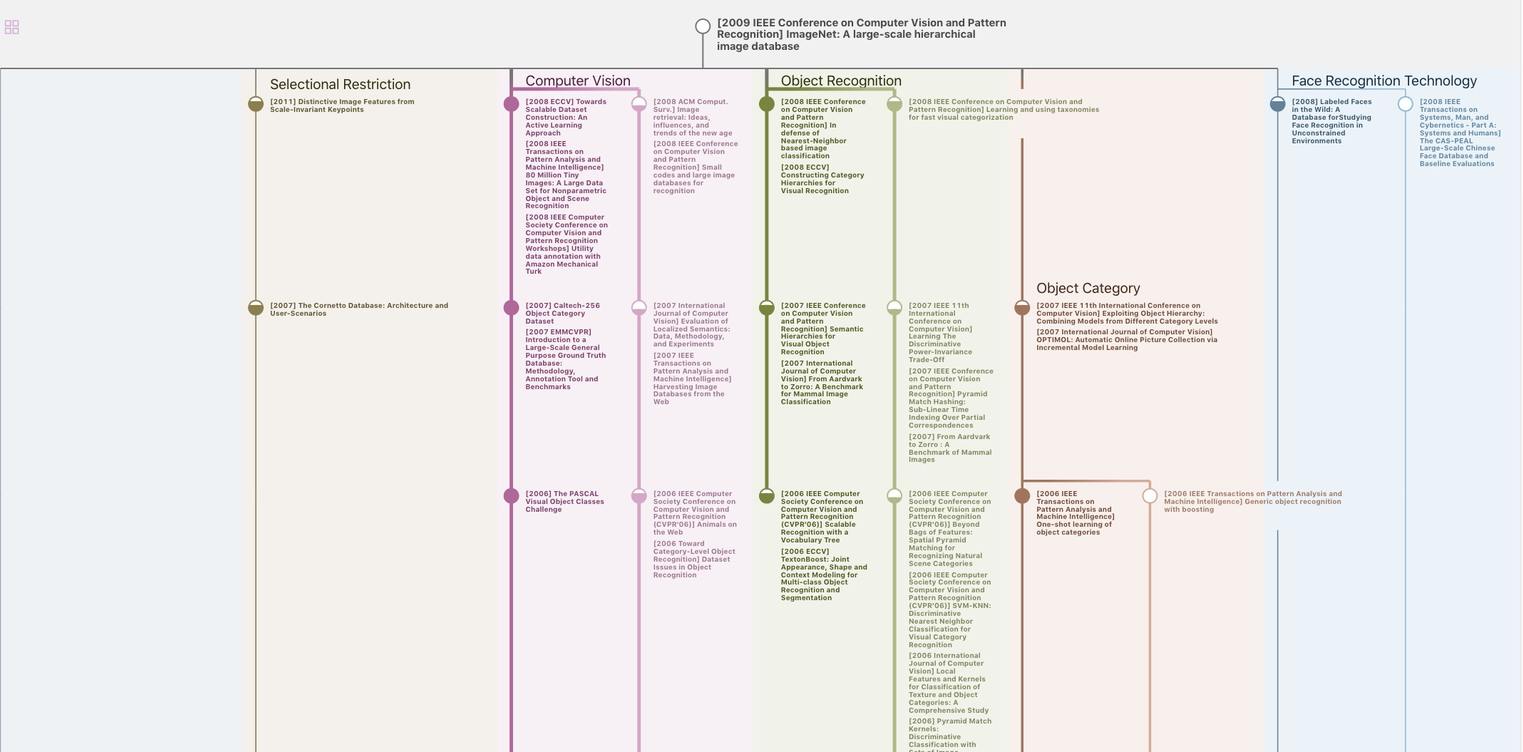
生成溯源树,研究论文发展脉络
Chat Paper
正在生成论文摘要