Outlier removal in biomaterial image segmentations using a non-stationary Bayesian learning
PATTERN ANALYSIS AND APPLICATIONS(2021)
摘要
Segmentation of dried amnion biomaterial tends to produce invalid (outlier) contour point detections due to texture and colour inhomogeneity of the biomaterial. In this paper, a novel implementation of a non-stationary Bayesian learning process for outlier contour point removal of amnion segmentations is presented. This outlier removal method is independent to algorithms used for the contour detection. The Bayesian process uses a non-stationary kernel to learn a function with complex shape that maps image features in a region-of-interest around each contour point to a discrete output. Based on this output, a contour point can be determined as valid or invalid (outlier). The hyper-parameters of the non-stationary kernel are learned by maximising the marginal likelihood of the combined likelihood of data and the prior of the kernel parameters. Moreover, a novel combination of gradient-ascend and harmonic heuristic search methods is presented to find the optimal hyper-parameters. To validate the method, experiments are conducted to detect and ignore invalid contour points on amnion biomaterial images. A comparison of the proposed method with a logistic regression classification as the baseline is performed. The results show that the proposed method can significantly improve the contour detection by removing outliers and, hence, can reduce waste of uncut biomaterials.
更多查看译文
关键词
Pattern recognition,Bayesian learning,Gaussian process,Non-stationary,Image segmentation
AI 理解论文
溯源树
样例
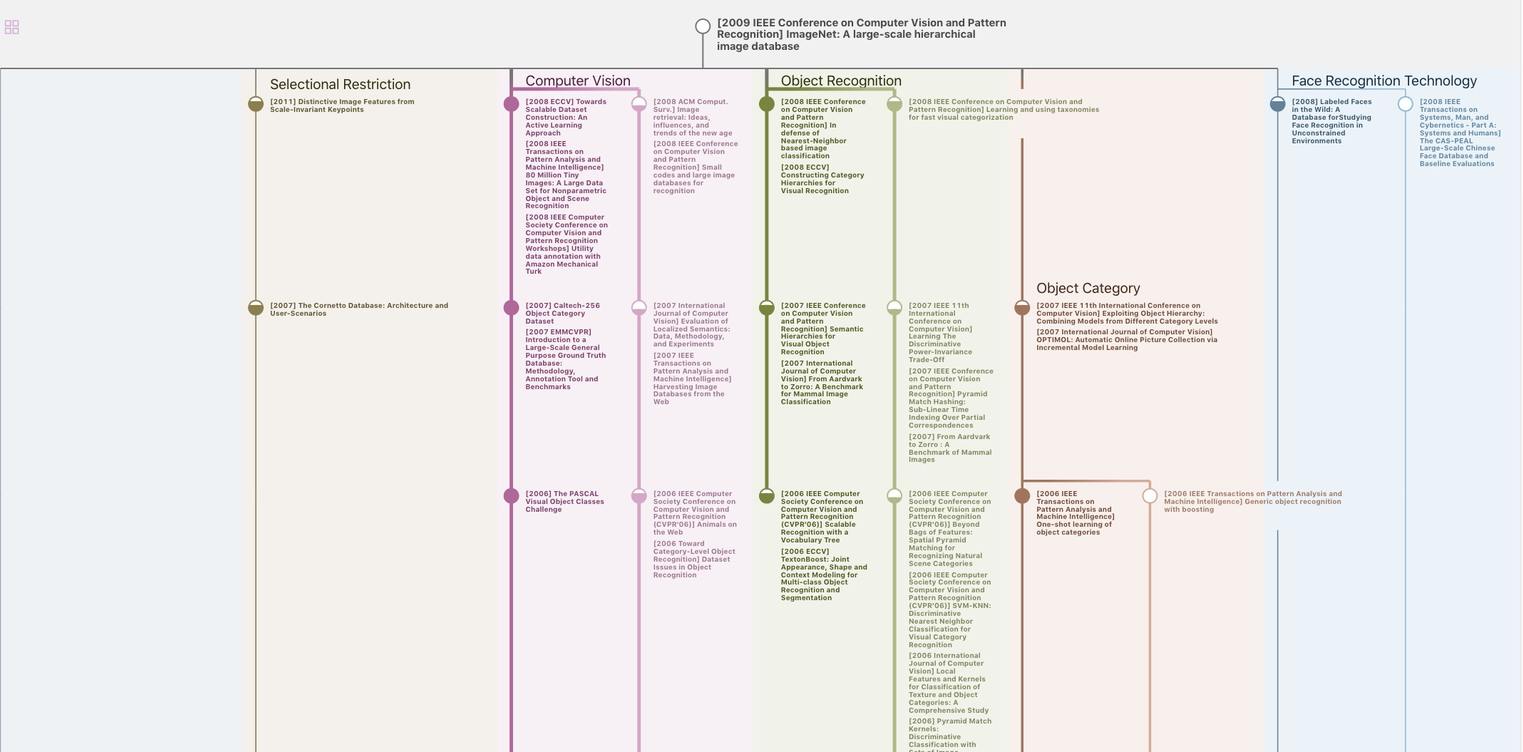
生成溯源树,研究论文发展脉络
Chat Paper
正在生成论文摘要