Positive-unlabeled learning in bioinformatics and computational biology: a brief review
BRIEFINGS IN BIOINFORMATICS(2022)
摘要
Conventional supervised binary classification algorithms have been widely applied to address significant research questions using biological and biomedical data. This classification scheme requires two fully labeled classes of data (e.g. positive and negative samples) to train a classification model. However, in many bioinformatics applications, labeling data is laborious, and the negative samples might be potentially mislabeled due to the limited sensitivity of the experimental equipment. The positive unlabeled (PU) learning scheme was therefore proposed to enable the classifier to learn directly from limited positive samples and a large number of unlabeled samples (i.e. a mixture of positive or negative samples). To date, several PU learning algorithms have been developed to address various biological questions, such as sequence identification, functional site characterization and interaction prediction. In this paper, we revisit a collection of 29 state-of-the-art PU learning bioinformatic applications to address various biological questions. Various important aspects are extensively discussed, including PU learning methodology, biological application, classifier design and evaluation strategy. We also comment on the existing issues of PU learning and offer our perspectives for the future development of PU learning applications. We anticipate that our work serves as an instrumental guideline for a better understanding of the PU learning framework in bioinformatics and further developing next-generation PU learning frameworks for critical biological applications.
更多查看译文
关键词
positive unlabeled learning, semi-supervised learning, machine learning, bioinformatics, pattern recognition
AI 理解论文
溯源树
样例
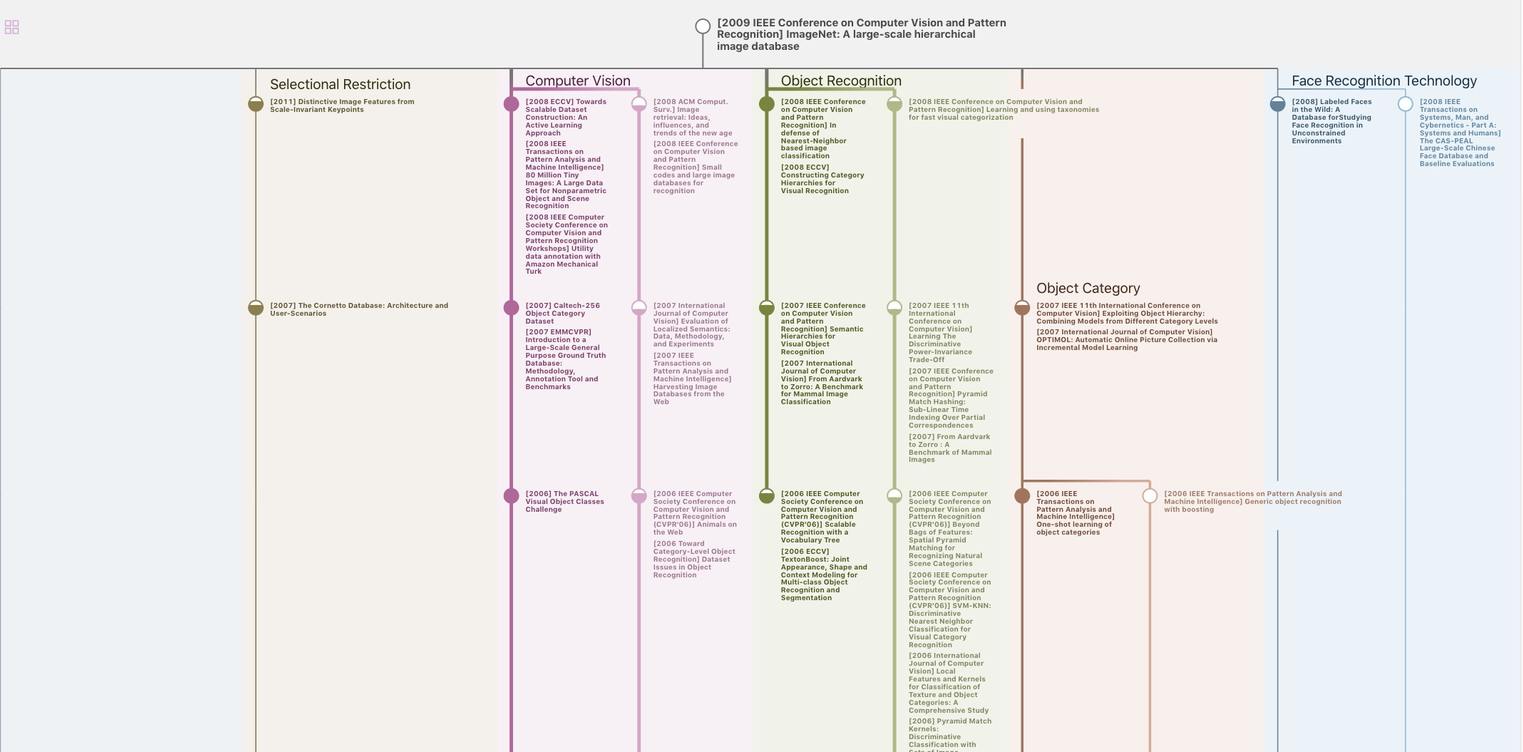
生成溯源树,研究论文发展脉络
Chat Paper
正在生成论文摘要