Constructing Neural Network-Based Models for Simulating Dynamical Systems
ACM Computing Surveys(2021)
摘要
Dynamical systems see widespread use in natural sciences like physics, biology, chemistry, as well as engineering disciplines such as circuit analysis, computational fluid dynamics, and control. For simple systems, the differential equations governing the dynamics can be derived by applying fundamental physical laws. However, for more complex systems, this approach becomes exceedingly difficult. Data-driven modeling is an alternative paradigm that seeks to learn an approximation of the dynamics of a system using observations of the true system. In recent years, there has been an increased interest in data-driven modeling techniques, in particular neural networks have proven to provide an effective framework for solving a wide range of tasks. This paper provides a survey of the different ways to construct models of dynamical systems using neural networks. In addition to the basic overview, we review the related literature and outline the most significant challenges from numerical simulations that this modeling paradigm must overcome. Based on the reviewed literature and identified challenges, we provide a discussion on promising research areas.
更多查看译文
关键词
Neural ODEs,physics-informed neural networks,physics-based regularization
AI 理解论文
溯源树
样例
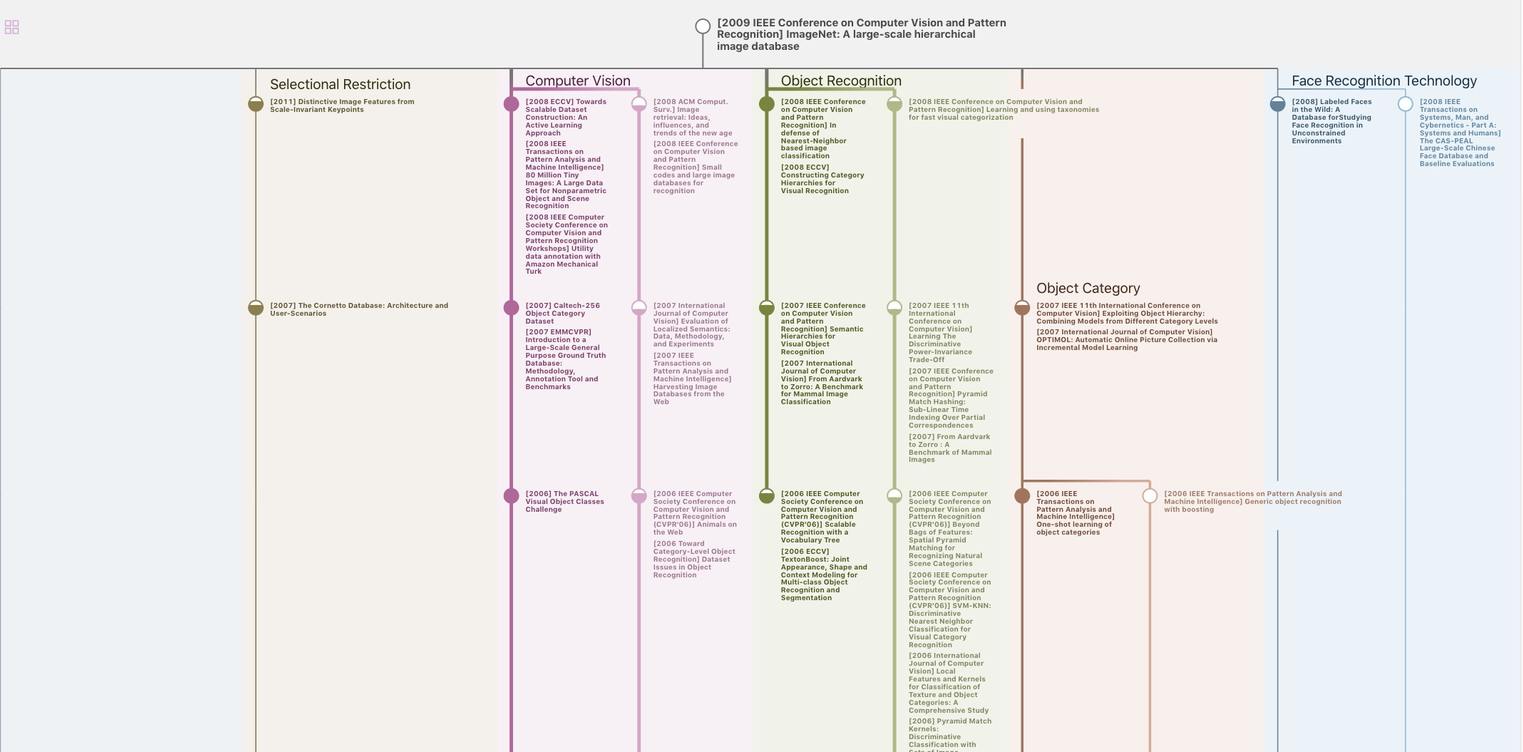
生成溯源树,研究论文发展脉络
Chat Paper
正在生成论文摘要