SNRi Target Training for Joint Speech Enhancement and Recognition
Conference of the International Speech Communication Association (INTERSPEECH)(2022)
摘要
This study aims to improve the performance of automatic speech recognition (ASR) under noisy conditions. The use of a speech enhancement (SE) frontend has been widely studied for noise robust ASR. However, most single-channel SE models introduce processing artifacts in the enhanced speech resulting in degraded ASR performance. To overcome this problem, we propose Signal-to-Noise Ratio improvement (SNRi) target training; the SE frontend automatically controls its noise reduction level to avoid degrading the ASR performance due to artifacts. The SE frontend uses an auxiliary scalar input which represents the target SNRi of the output signal. The target SNRi value is estimated by the SNRi prediction network, which is trained to minimize the ASR loss. Experiments using 55,027 hours of noisy speech training data show that SNRi target training enables control of the SNRi of the output signal, and the joint training reduces word error rate by 12% compared to a state-of-the-art Conformer-based ASR model.
更多查看译文
关键词
Speech enhancement, signal-to-noise ratio improvement, multi-task learning, noise robust ASR
AI 理解论文
溯源树
样例
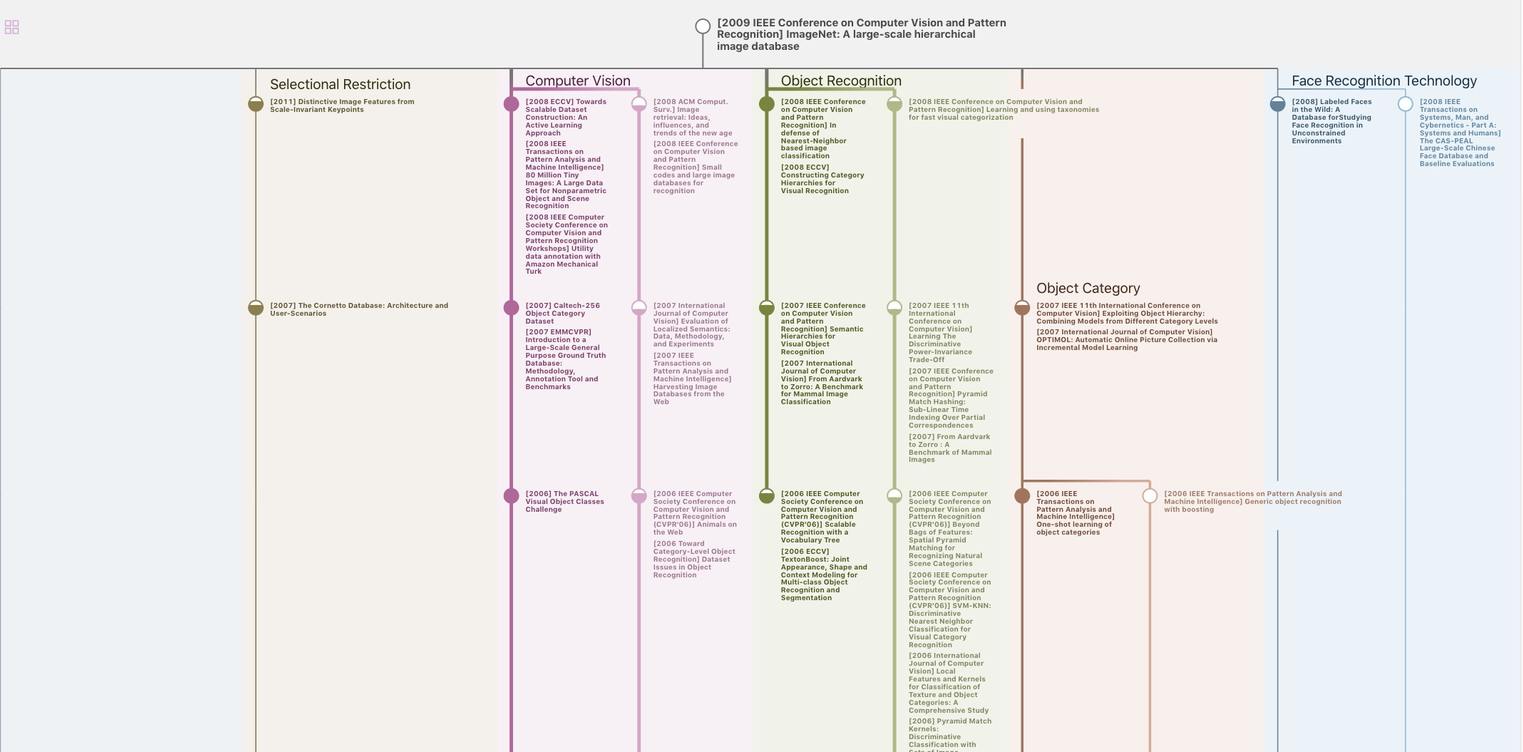
生成溯源树,研究论文发展脉络
Chat Paper
正在生成论文摘要