A Systematic Investigation of Commonsense Knowledge in Large Language Models
arxiv(2022)
摘要
Language models (LMs) trained on large amounts of data have shown impressive performance on many NLP tasks under the zero-shot and few-shot setup. Here we aim to better understand the extent to which such models learn commonsense knowledge -- a critical component of many NLP applications. We conduct a systematic and rigorous zero-shot and few-shot commonsense evaluation of large pre-trained LMs, where we: (i) carefully control for the LMs' ability to exploit potential surface cues and annotation artefacts, and (ii) account for variations in performance that arise from factors that are not related to commonsense knowledge. Our findings highlight the limitations of pre-trained LMs in acquiring commonsense knowledge without task-specific supervision; furthermore, using larger models or few-shot evaluation are insufficient to achieve human-level commonsense performance.
更多查看译文
关键词
commonsense knowledge,large language,models
AI 理解论文
溯源树
样例
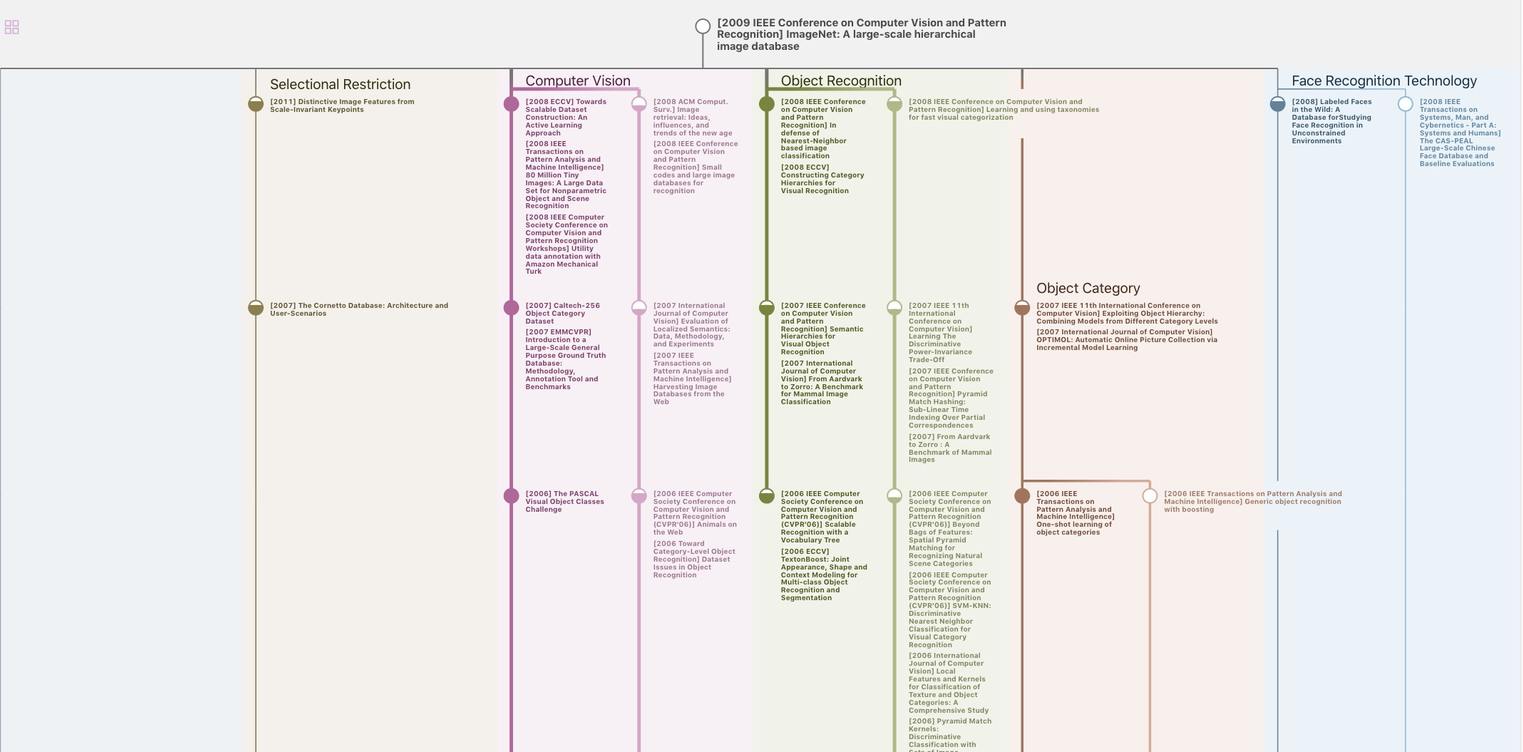
生成溯源树,研究论文发展脉络
Chat Paper
正在生成论文摘要